Defeat The Machine Learning Engineer Impostor Syndrome
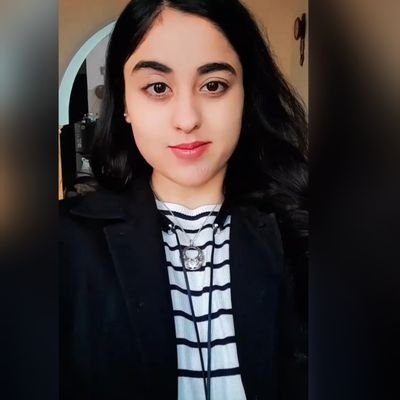
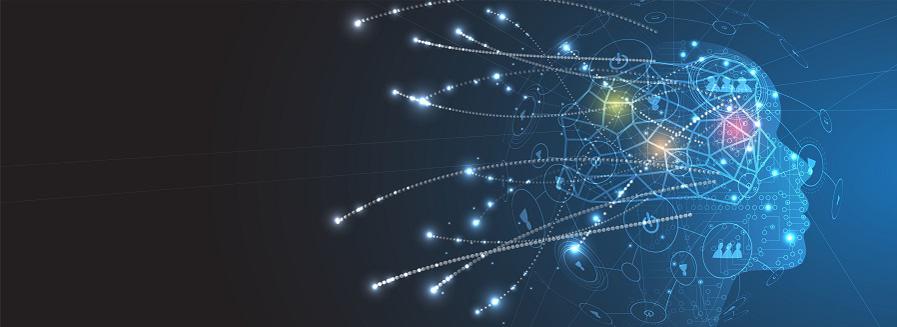
#1. Be brave and try freelancing
Being brave is the thing that will help you the most. And working freelance IS brave. When you work as a freelancer/contractor, feedback from your work does not come in quarterly or yearly reviews. It comes every single day. And there is no way to hack that. Clients expect you to deliver quality and fast. By the way, this is the main reason why you will be better paid than in your current job.
Once you feel you have the fundamentals of ML right, put yourself in the ring. Test yourself. You are smart, and you can do it. Taking more online courses does not make the impostor syndrome go away. Believe me.
The top 2 freelancing platforms IMHO are
Toptal. The world’s number 1 top-talent freelance platform in the world. They have a rather harsh application process, but it is very worth going through it. Great enterprises and rising startups are using Toptal to implement ML solutions these days. Getting there will give you plenty of opportunities to shine. Braintrust. An emerging talent network, inspired by a fresh new economic model (heard of Ethereum?). They are growing fast, and I expect them to catch Toptal soon.
#2. Never forget the data. NEVER
ML engineering is harder than traditional software engineering because of DATA.
Rare are the occasions when you are given a clean and complete set of features and labels to build your ML model. Instead, you often need to generate the training data yourself. The most common problems I have faced in this sense are:
The training data has a bug. Usually, you generate this data from an SQL query and some kind of Python script to automate the extraction of raw features and labels. Writing SQL queries is simple, but debugging them can get pretty hard. Working on your SQL skills is one of the best things you can do to become a better ML engineer. The training data is incomplete. After generating the first version of your training data, you jump to the next phase of ML development and build a quick baseline model. More often than not, this baseline model is not good enough to solve the business problem, and so you need to iterate. Non-experienced ML developers tend to focus too much on improving the model and forget about the dataset they created. This is a typical mistake that causes frustration and impostor syndrome kind of feelings. Go back to the DATA. Extend your SQL query to add more relevant features. Talk to domain experts in your environment (data engineers, business intelligence guys…) that can help you fetch the data that will move the needle and unblock you. DATA is the magic ingredient that fuels all models, from simple linear regressions to colossal Transformer models. If the fuel is not ok, it does not matter which car you are driving. You are not going to move.
This sounds so trivial and stupid that we (I include myself) ML engineers have a surprising tendency to forget. As you get more experience building ML solutions, you get better at remembering this and going back to the DATA whenever you hit a wall.
You cannot use Stackoverflow to debug your dataset. You are alone there. And you need to change your mindset. You have to behave as a problem-solver. You need to get to know the dataset, and the best way is to visualize it. I personally love Tableau Desktop, but there are other options out there like Power BI, Apache Superset, etc. There are even Python libraries if you prefer, like sweetviz.
No matter the tool you prefer, go back to the DATA every time you get stuck.
#3: Do not expect to know everything (especially at the beginning).
Machine learning is a field that covers a wide spectrum of technical complexity: software development, operationalization (MLOps), classical ML, cutting-edge research on Deep Learning, hardware optimization…
If you try to cover everything, you will lose focus and wander too much on the surface. Knowing something in ML means you have implemented it yourself. Full stop.
It is fantastic to keep up to date with the latest advancements in DL, for example. But do it in a principled way. Set yourself a clear goal (e.g., I want to become an expert in Transformer models) and build yourself a path towards that goal, selecting relevant papers, libraries, webinars, and even conferences.
Jumping from one topic to another keeps you busy but not focused. Stay humble. Start small and focused. Once you get there, take the next step and conquer another field.
Conclusion Conquering your fears is a daily (full-time) job. Not only in Machine Learning but in every aspect of your life in which you want to grow and be better tomorrow.
Subscribe to my newsletter
Read articles from Samridhi Bhandari directly inside your inbox. Subscribe to the newsletter, and don't miss out.
Written by
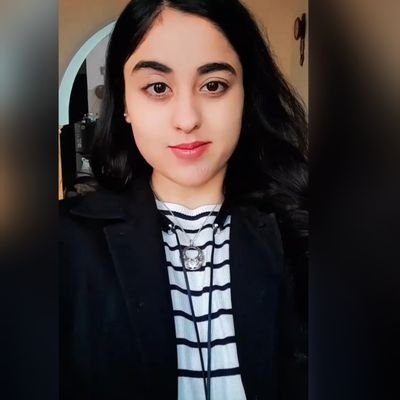
Samridhi Bhandari
Samridhi Bhandari
I believe in making projects that solve real-world problems. Languages I Know C#,C/C++,Elixir,Go,HTML/CSS,Java,JavaScript,Kotlin,PHP,Python,Flask framework. I'm currently pursuing my undergraduate degree in Information and Technology from IPU.