Understanding Augmented Analytics

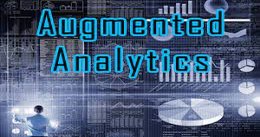
In the 2017 Hype Cycle for Emerging Technologies report, Gartner coined the phrase "augmented analytics," stating it would be the "future of data analytics." Augmented analytics is the process of automatically extracting data from raw data sources, cleaning, analyzing, and communicating it in a report that humans can comprehend using natural language processing. Augmented analytics searches for patterns in data or explores other insightful information using machine learning without the engagement of data scientists. This information can then be shared with the human team. People within the organization do not need to wait for an intermediary tech professional to explain what the machine saw in the data because the reporting is simple enough for a non-technical person to comprehend.
Thus many business problems cannot be solved through algorithms or machine learning. Augmented analytics combines human inquisitiveness and artificial intelligence. This makes business intelligence and data analytics more usable by allowing users to ask questions and generate knowledge from data via simple colloquial and visual interactions. Finally, this leads to deeper insights and data-driven choices that boost a firm's productivity. This article will briefly introduce augmented analytics, its benefits, use cases, and challenges. We would also look at some augmented analytics software available for use, and lastly, we would see how data-augmented analytics can be applied.
Prerequisites
Before reading this article, you need to have prior knowledge of at least one of the following:
Data analysis
Data visualization
Business intelligence
AI (Data science and machine learning)
Benefits of Augmented Analytics
There are many advantages to using augmented analytics in your organization; we’ll look at the benefit of augmented analytics to both companies and the software suppliers, which:
- Benefits of augmented analytics to organizations
Augmented analytics aid in data literacy
Augmented analytics minimizes the chance of losing crucial information
It improves the outcome of the decisions
It lowers the amount of time spent on investigation and the discovery of incorrect and irrelevant information.
2.Benefits of augmented analytics to software suppliers
It improves the user experience
It boosts your revenue streams
Use Cases of Augmented Analytics
Augmented analytics can be used in a variety of fields and for a variety of purposes to help push business changes; a few of the use cases are listed below.:
Healthcare: Enhancing operations to improve patient outcomes
Hospitals can maximize their operations using augmented analytics by evaluating key parameters such as duration of stay and bed occupancy rate. The information acquired would help hospitals divide resources and manage crucial ground operations like doctor and patient schedules.
Manufacturing: For Successful Business, Effective Maneuvering
Manufacturers can examine crucial data such as manufacturing line capabilities, shipment timelines, labor schedules, and warehouse space availability with the help of augmented analytics. The information gained will enable manufacturers to make a strategic shift to produce in-demand products, such as face masks during the pandemic, to generate much-needed income to keep the business afloat.
Telecommunications: Maintaining Competitiveness with a Changing Customer Base
Telecommunications companies can use augmented intelligence to test their data and gain insights into how to bundle services to meet the needs of today's customers while maintaining optimal profitability. This entails examining data on the costs of their services (like internet speed, voice-call minutes, or client usage statistics for the same services) to create distinct packages that are both reasonable and suited to each customer profile.
Reasons Why Organisations Should Adopt Augmented Analytics
Rather than replacing employees, the basic idea behind augmented intelligence is to augment human information, automate repetitive operations, and empower enterprises. Let's look at some of the reasons why companies should use augmented analytics.
Democratization data: Data democratization necessitates a well-thought-out data strategy, culture, and structuration. Systems for augmented analytics aren't just for analysts; they're for everyone. These systems are for non-technical business users, and as a result, everyone can communicate with data.
**Providing more value: **While creating deeper enterprise solutions, it is more time-consuming to perform repetitive tasks manually rather than taking the effort to incorporate analytics with human intelligence. As a result, it is advantageous to create an automated system capable of performing tasks such as data preparation, machine learning (ML) and deep learning (DL) algorithm implementation, and insight extraction., among other things. In the long run, this is helpful to all types of businesses.
Effective data-driven decisions: Augmented analysis makes it possible for users to make better effective, data-driven decisions. It also enables large business users to pinpoint the source of problems and implement data-driven solutions.
Augmented analytics software and their applications
The following are some examples of augmented analytics software that are currently available and how they are used.
- Cognos Analytics from IBM: The Cognos Analytics platform is a self-service solution that allows users to create dashboards and reports using data. IBM Watson Analytics provides a machine learning-enabled user experience with automated pattern detection, natural language query, generation assistance, and enhanced actionable insights. On-premises and cloud-hosted versions of IBM's business intelligence software are usable.
- Microsoft's Power BI: Power BI is a business intelligence tool that runs in the cloud on Microsoft's Azure platform. Individual or power users who create complex data mashups with in-house data sources can also use on-prem capabilities. Power BI is unique in that it allows users to prepare data, discover data, and create dashboards, all with the same tool. The platform integrates with Excel and Office 365, and a thriving user community aids in the tool's development.
- Einstein Analytics Platform from Salesforce: The Salesforce Einstein Analytics platform comes in various flavors, depending on the role, business, and attributes included. Users can customize their applications' analytics and improve observations with specific recommendations and help. In addition, you can develop advanced experiences with Einstein by using customizable templates, third-party apps, or custom-built dashboards.
Challenges of Augmented Analytics
The following are some of the organisations' most significant obstacles when implementing augmented analytics.
Scalability and effectiveness: Augmented analytics may need significant computational power based on your system and abilities. Bear in mind that the volume of data significantly impacts reaction time.
Building trust: Admins who use augmented data exploration must understand how to instill faith in the insights that emerge, as well as confidence that employees will not be laid off.
The efficiency of the training set: Your insights will be worthless if you don't have the appropriate data for training your analytical models. Maintain and update models to ensure that the quality of your insights remains high.
Conclusion
Any company seeking to be data-driven should invest in augmented analytics. Augmented analytics is a worthwhile investment for businesses looking to scale their production process. However, organizations should exercise proper data management to get the most out of an augmented analytics platform.
References
Tags: #Data-Analysis, #Business-Intelligence, #Augmented-Analytics, and #Artificial-Intelligence.
Subscribe to my newsletter
Read articles from Abdulsamod Azeez directly inside your inbox. Subscribe to the newsletter, and don't miss out.
Written by

Abdulsamod Azeez
Abdulsamod Azeez
I’m a Data Scientist and Machine Learning Engineer with years of experience implementing data-driven solutions (to improve the effectiveness, accuracy, and utility of internal data preprocessing), building ML models using predictive data, delivering insights, and implementing action-oriented business problems.