Deepfake Content: Possible Risks And Ways To Mitigate Them Through AI
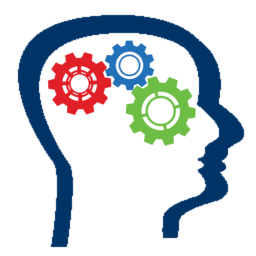
Table of contents
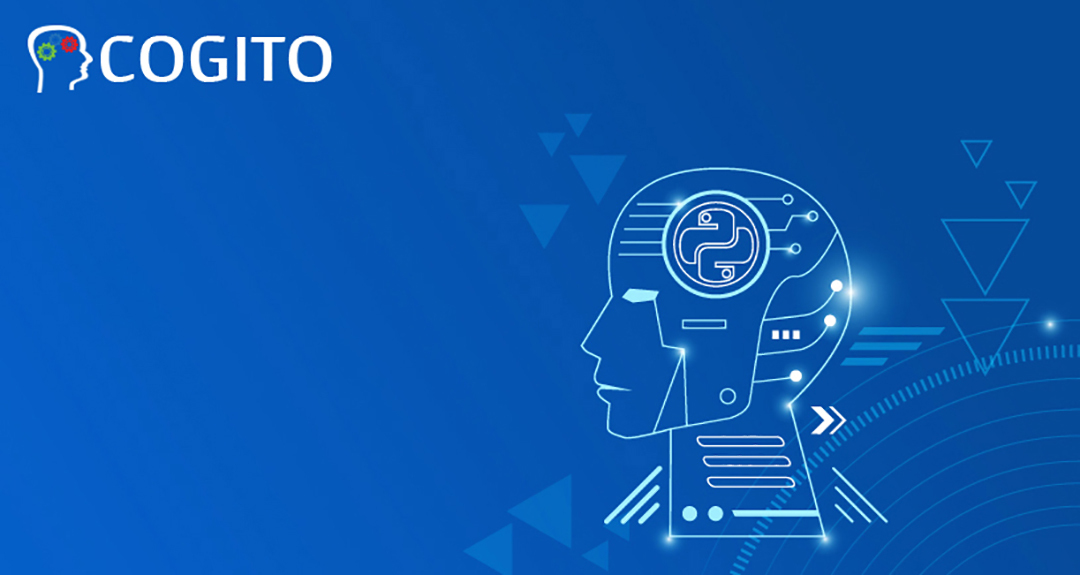
Technology that distorts reality has become more sophisticated than ever before. There is a disruption in the truth as a result of this technological change. Some of such distortions of reality are intended to be humorous, while others are not. In addition to posing a threat to national security and democracy, they might also threaten the identities of individuals. You could be defamed and have your privacy invaded by a deepfake video. The stir and, violence triggered by fake online content can create tensions between politicians and cause violence; it can jeopardize the career or life dreams of teenagers.
With recent advances in artificial intelligence (AI) and cloud computing technologies, audio, video, and image manipulation techniques have become more accessible to the mass, providing ease and efficiency to manipulate authentic audio, video, and image data. Media content manipulated using AI-based tools is commonly referred to as "deepfake." For example, the voice of a public figure can be recreated, or a face superimposed onto the body of another.
Duality is a characteristic of deepfake content. There are a number of positive aspects to using deepfake video technology. In order to facilitate telephonic communication for those with hearing impairments, an app that generates lip movements based on the audio can be downloaded on a smartphone or a tablet. Furthermore, it can facilitate the production of realistic multilingual films. Sadly, negative uses of deepfake content are more prevalent.
Deepfake Generation
This new technique reduces manipulation traces, which are crucial to traditional forged media generation techniques. It overcomes the significant flaws that traditionally existed. Forgery detection methods like biometrics or compression artifacts inconsistencies have also been exploited to find fake yet real-looking fingerprints. Using a deep neural network, it extracts input characteristics and reconstructs the entire new fake media content based on the segmentation map or latent representation.
Deepfake Detection
There are two approaches to detecting deep fakes. In the first method, handcrafted features are relied upon, and in the second method, computers may be utilized. Learned features are the focus of deep learning approaches. Deepfake detection pipelines also involve input preprocessing, feature extraction, and classification, just like traditional forgeries detection methods, such as copy-move, splicing, and inpainting.
How deepfake content destroys the integrity and authenticity of social media content
Deepfake videos have made image forgery a serious problem in the last few years. Using deep learning technology, deep fake videos replace a person's face, emotion, or speech with another person's. Due to their sophistication, it is often difficult to detect manipulation traces in these videos. Both individuals & society, and brands & businesses can be negatively affected by them on social, political, and emotional levels. As social media platforms are vulnerable, they can be used to blackmail or defame someone.
Modern society can't exist without social networks and media. Although deepfake content on social media shouldn't negatively affect people's mental health, it has negatively impacted someone's social status and mental health. It is possible to take photos and videos with a mobile or mini camera anywhere and at any time. Fake images/videos can be created with commercial photo editing tools. Therefore, in the face of multimedia forgery, the privacy and identity of people should be protected, especially on social media.
In what ways can the DFDC model be applied to deepfake detection?
To address the problem of the malicious use of deepfake technology, the research and commercial communities have developed a number of methods to verify the integrity of multimedia files and to detect deepfake videos.
Most of the methods attempt to detect deepfake videos by analyzing pixel values. These methods rely on the visual artifacts created while placing the fake face on the target face. The artifacts are significant because they represent missing information that the deep neural network did not see in the training data (e.g., teeth), sometimes because it is hidden behind another object (e.g., hair strand).
The deep network cannot estimate the information and therefore assigns it a lower quality in the deepfake version or even creates holes in these parts.
Challenges in resolving the deepfake content
A high level of compression is applied to uploaded images/videos on social media and other online platforms. Both smartphones and tablets are used to access social media accounts. In order to detect deepfakes, existing solutions usually work on uncompressed data, which is not suitable for videos uploaded to social media. Due to the popularity of smartphones for checking social media accounts, the model should also be small. Three things have to be done simultaneously: detect deepfakes, develop lightweight models for compressed videos, and implement the models.
Having addressed the challenges mentioned above, we propose a new method that utilizes a key video frame approach in conjunction with our previously proposed neural network-based model, which can detect deepfake videos in social media that are compressed at any level. We limit the number of frames extracted from each video during data analysis to only the keyframes. In some cases, it is even possible to reduce the number of video frames that must be checked for authenticity while maintaining high levels of accuracy.
We don't check every frame for authenticity since we only check changes to key video frames during detection. The computations are reduced as a result. Our network can be applied at the edge since the detection process involves fewer data computations. We propose a lightweight approach to counter the high computational cost of existing approaches.
Cogito can help develop algorithms to detect deepfakes using DFDC datasets
It is challenging and technically demanding to identify manipulated or falsified content. Detecting image and video forgery has been a major focus in media forensics for two decades. It's mostly copies-moves, dropped frames, duplicates, or varying interpolations that are proposed for video forensics. Prior detection techniques have not been able to detect such computer-generated forged videos.
With the deepfake detection challenge dataset, Cogito can assist with the development of AI tools that can detect deepfake content posted on social media and digital platforms. The dataset can be used in machine learning models to detect deepfake content. We have acquired the expertise to develop quality training data addressing the deepfake detection challenge as a result of our decade-long exposure to AI and machine learning.
The AI enterprise can automatically utilize our artificial intelligence training data to identify false content on social media and digital platforms with the help of automated deepfake content detection tools. We can help with AI training data to develop a deepfake content detection model that promises a high level of accuracy and lower computational requirements.
Final Thought
With AI, social media deepfakes can be detected with very high accuracy and less computing power. We have built a deepfake content detection model based on our data that can detect nearly all fake videos with multiple keyframes. Cogito's 1500+ data experts can help provide your deepfake content detention model with the DFDC datasets as it has experience preparing AI training data for this purpose. Having a GDPR-compliant business facility is one of our major advantages in ensuring your data is completely secure and private while still ensuring that deepfake detection is performed at par with accuracy and privacy.
Subscribe to my newsletter
Read articles from Rohan Agrawal directly inside your inbox. Subscribe to the newsletter, and don't miss out.
Written by
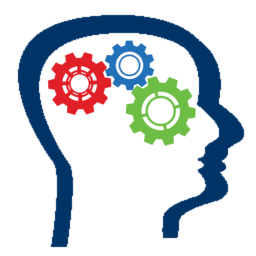
Rohan Agrawal
Rohan Agrawal
Cogito Tech offers Enterprise Data Labeling Services (EDLS), specializing in data curation and labeling. Our commitment to quality and ethical integrity is verified by the DataSum certification, ensuring transparency, ethical sourcing, and the highest data governance standards. This commitment enables us to deliver high-quality, ethically sourced data that you can trust, ensuring compliance, reliability, and effectiveness. You'll never need to choose between achieving a competitive edge and sourcing data responsibly.