Lakehouse Architecture - will it be the future of data warehouse?
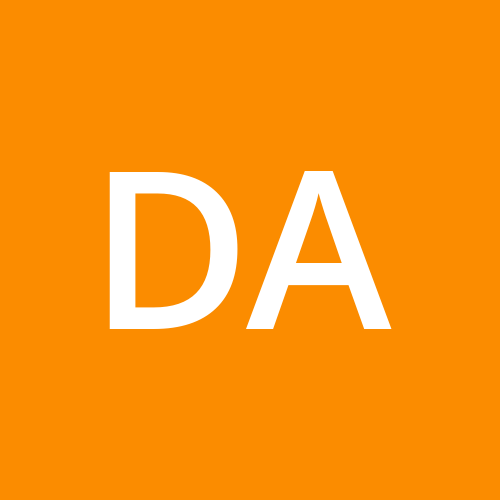
Image Source: Data Lakehouse – Databricks
One of the foundational papers (Lakehouse: A New Generation of Open Platforms that Unify Data Warehousing and Advanced Analytics (cidrdb.org)) coined the idea of Lakehouse which explores the opportunity to have a new architecture pattern based on open direct-access data formats that offers state-of-the-art performance and support for ML and Data Science but gives significant cost benefits.
The most popular two tier (data lake + warehouse) architecture of today, such as: a combination of S3 and Redshift (AWS) or ADLS and Synapse SQL pools (Azure), which started to evolve from first generation analytics platforms a decade ago and with the invent of data lake it resolved some key problems of the older platforms.
Although major cloud data warehouse services have now the capability to connect to object store data and also started supporting Machine Learning & DS, the current architecture has its own challenges, TCO, complexity to maintain data reliability and keeping data up-to-date, limited support to advanced analytics, not so performant connectors between Data warehouse and cheaper object store.
One of the key challenges of implementing the idea of building a complete data warehouse on cheaper object store was its inability to support ACID transactions, and Lakehouse architecture with the help of Delta Lake engine has now proved that is possible. But the biggest question is will it be able to match the performance, and according to this paper it's possible with the optimizations such as: caching, auxiliary data structures and data layout optimizations.
I have been working on implementing a Unified Data Lakehouse architecture based on Azure Synapse. While I found it's significantly cheaper compared to an MPP like Synapse Dedicated SQL pool, but the main question is performance & complexity, and depending on your organization's data volume, user base and requirements, the decision need to be made. A lot depends on the design & orchestration of the overall process as well.
With the increase in requirements of ingesting and storing heterogenous data, data lake will continue to be the primary storage for Organizations and thus a better management of data in data lake and better support for large scale ML and DS workloads will be required. Lakehouse is on the right path, but may still need a few more years to gain the popularity.
A few points from my experience:
1. Technology innovation and latest trends should not take full control of the Organization data architecture decisions, as every Organization has different data landscape and requirements
2. It's important to serve business data quickly and efficiently, but we have to be pragmatic and do what is right given the scenario. We should not skip basics and key data architecture principles.
3. In reality, implementations are way more complex than a new solution idea, so a feasibility check is always required
What do you think is the future of Data warehouse? Thoughts?
Read my Original post here: Data Lakehouse
Subscribe to my newsletter
Read articles from dataguru directly inside your inbox. Subscribe to the newsletter, and don't miss out.
Written by
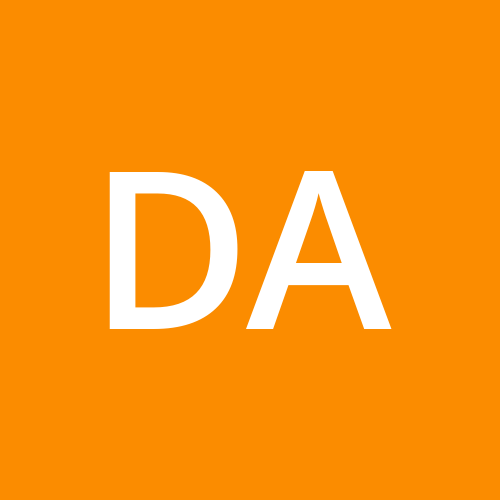
dataguru
dataguru
I have 15 years of experience in Data Architecture, Data Engineering, Data Warehousing, Data Modelling, Data Analysis, Building modern data platforms on Cloud (AWS & Azure), AI/ML Strategy & Solutioning, Tech Consulting, Data Strategy etc. I love gardening, photography and soft music.