Recent Discovery: VALL-E The Voice Of DALL-E by Microsoft

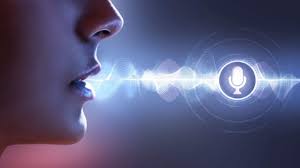
Microsoft’s new zero-shot text-to-speech model can duplicate everyone’s voice in three seconds.
This Article is all about the recent Discovery and research by Microsoft in the field of text-to-speech that come up with the Voice version of DALL-E.
Recently we have all been Amazed by the work ChatGPT does and again AI industry got shaken up by this latest discovery “VALL-E”!! 🤩The voice of DALL-E. It’ll definitely make a revolution in AI for the people who can’t speak but can write. Any human can speak because of VALL-E right now.
VALL-E is an amazing technological feat that has the potential to change the way we interact with digital media.
Without further ado let’s see How it’s created!!
Introduction
Source: Research Paper of VALL-E
As Microsoft recently released VALL-E, a new language model approach for text-to-speech synthesis (TTS) that uses audio codec codes as intermediate representations. It demonstrated in-context learning capabilities in zero-shot scenarios after being pre-trained on 60,000 hours of English speech data*.*
With just a three-second enrolled recording of an oblique speaker serving as an acoustic prompt, VALL-E can create high-quality personalized speech. It supports contextual learning and prompt-based zero-shot TTS techniques without additional structural engineering, pre-designed acoustic features, and fine-tuning. Microsoft has leveraged a large amount of semi-supervised data to develop a generalized TTS system in the speaker dimension, which indicates that the scaling up of semi-supervised data for TTS has been underutilized.
VALL-E can generate various outputs with the same input text while maintaining the speaker’s emotion and the acoustical prompt. VALL-E can synthesize natural speech with high speaker accuracy by prompting in the zero-shot scenario. According to evaluation results, VALL-E performs much better on LibriSpeech and VCTK than the most advanced zero-shot TTS system. VALL-E even achieved new state-of-the-art zero-shot TTS results on LibriSpeech and VCTK. This is a significant improvement over previous models, which required a much longer training period in order to generate a new voice.
It is interesting to note that people who have lost their voice can ‘talk’ again through this text-to-speech method if they have previous voice recordings of themselves. Two years ago, a Stanford University Professor, Maneesh Agarwala, also told AIM that they were working on something similar, where they had planned to record a patient’s voice before the surgery and then use that pre-surgery recording to convert their electrolarynx voice back into their pre-surgery voice.
AI can mimic emotions
The field of voice synthesis has advanced significantly in recent years thanks to the development of neural networks and end-to-end modeling. Currently, vocoders and acoustic models are often utilized in cascaded text-to-speech (TTS) systems, with mel spectrograms acting as the intermediary representations. High-quality speech from a single speaker or a group of speakers can be synthesized by sophisticated TTS systems.
TTS technology has been integrated into a wide range of applications and devices, such as virtual assistants like Amazon's Alexa and Google Assistant, navigation apps, and e-learning platforms. It's also used in industries such as entertainment, advertising, and customer service to create more engaging and personalized experiences.
Features Of VALL-E
The features of Vall-E are as follows:
Synthesis of Diversity: VALL- E’s output varies for the same input text since it generates discrete tokens using the sampling-based method. So, using various random seeds, it can synthesize different personalized speech samples.
Acoustic Environment Maintenance: While retaining the speaker prompt’s acoustic environment, VALL-E can generate personalized speech. VALL-E is trained on a large-scale dataset with more acoustic variables than the data used by the baseline. Samples from the Fisher dataset were used to create the audio and transcriptions.
Speaker’s emotion maintenance: Based on the Emotional Voices Database for sample audio prompts, VALL-E can build personalized speech while preserving the speaker prompt’s emotional tone. The speech correlates to a transcription and an emotion label in a supervised emotional TTS dataset, which is how traditional approaches train a model. In a zero-shot setting, VALL-E can maintain the emotion in the prompt.
but there are some cons that vall-e model suffers through and they are yet to be improved i.e. synthesis robustness, data coverage, and model structure.
Synthesis robustness: During testing, they found that some words may be unclear, missed, or duplicated in speech synthesis. It is mainly because the phoneme-to-acoustic language part is an autoregressive model, in which disordered attention alignments exist and no constraints to solving the issue. The phenomenon is also observed in vanilla Transformer-based TTS, which was addressed by applying non-autoregressive models or modifying the attention mechanism in modeling.
Data coverage: Even if we use 60K hours of data for training, it still cannot cover everyone’s voice, especially accent speakers.
Model Structure:
Broader impacts: It could mislead one’s identity and leads to impersonating people.
"Since VALL-E could synthesize speech that maintains speaker identity, it may carry potential risks in misuse of the model, such as spoofing voice identification or impersonating a specific speaker. To mitigate such risks, it is possible to build a detection model to discriminate whether an audio clip was synthesized by VALL-E. We will also put Microsoft AI Principles into practice when further developing the models."
for more detailed info about the model and its features Here is the Research Paper of VALL-E. you can get a brief understanding of each and every technique/idea behind creating VALL-E.
This article is just for you to get overviewed of the recent advancement and discoveries in the text-to-speech field. For more such articles in AI and want to update yourself in the evolving field of AI, you can follow me on Linkedin, and GitHub. I’ll be happy to connect with you all.
Thank you and Happy Learning:)
Subscribe to my newsletter
Read articles from Samiksha Kolhe directly inside your inbox. Subscribe to the newsletter, and don't miss out.
Written by

Samiksha Kolhe
Samiksha Kolhe
Hey I'm Samiksha Kolhe. a Data Enthusiast and aspiring Data Scientist. One day Fascinated by a fact that "We can built Time machines and predict future using AI". That hit my dream to explore the Vector space and find out what the dark matter is about. World and Technology every day brings new challenges, and new learnings. Technology fascinated me, I'm constantly seeking out new challenges and opportunities to learn and grow. A born-ready girl with deep expertise in ML, Data Science, and Deep Learning, generative AI. Curious & Self-learner with a go-getter attitude that pushes me to build things. My passion lies in solving business problems with the help of Data. Love to solve customer-centric problems. Retail, fintech, e-commerce businesses to solve the customer problems using Data/AI. Currently learning MLops to build robust Data/ML systems for production-ready applications. exploring GenAI. As a strong collaborator and communicator, I believe in the power of teamwork and diversity of thoughts to solve a problem. I'm always willing to lend a helping hand to my colleagues and juniors. Through my Hashnode blog, I share my insights, experiences, and ideas with the world. I love to writing about latest trends in AI and help students/freshers to start in their AI journey. Outside technology I'm a spiritual & Yoga person. Help arrange Yoga and mediation campaigns, Volunteering to contribute for better society. Love Travelling, Reading and Learn from world.