Outlier Detection and Treatment: Advanced Techniques for Handling Outliers in Data
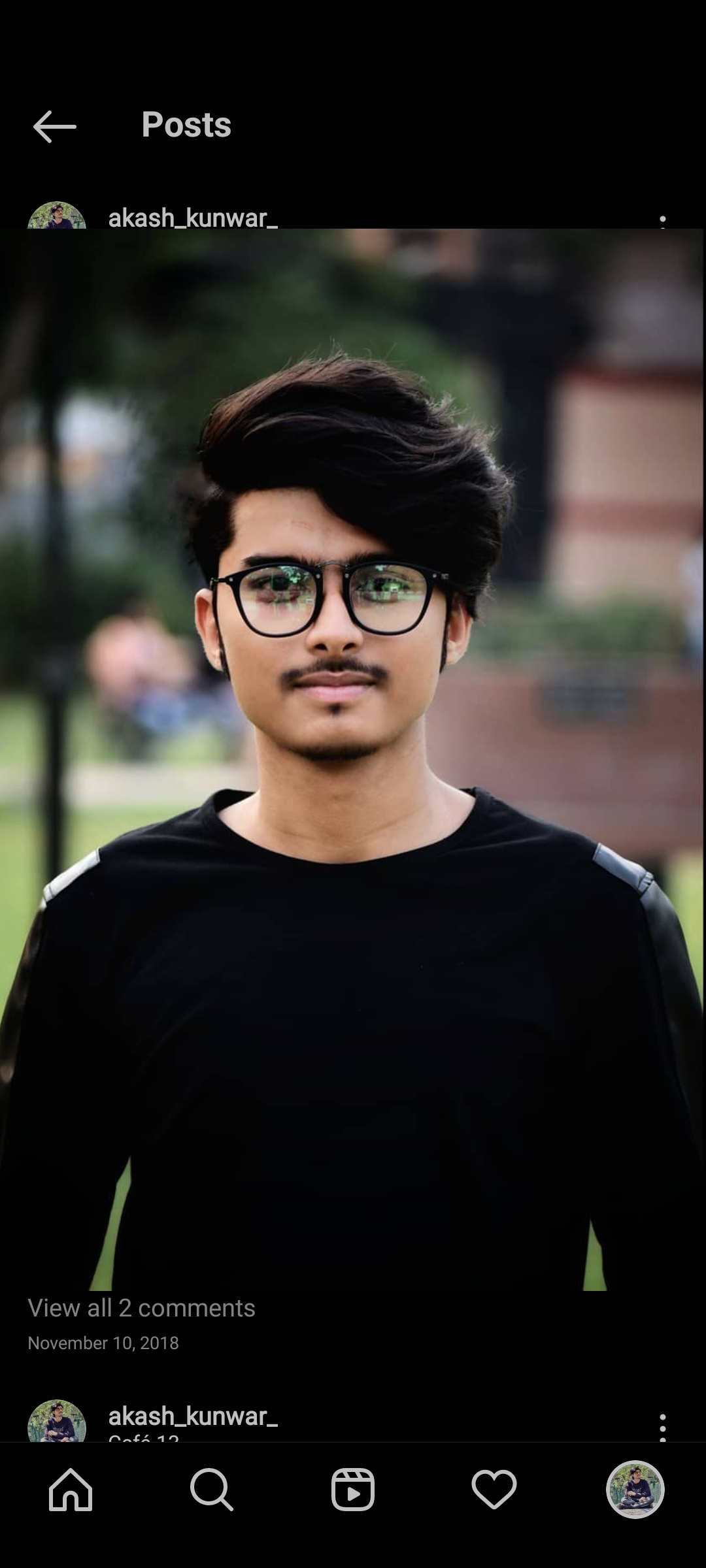
Table of contents
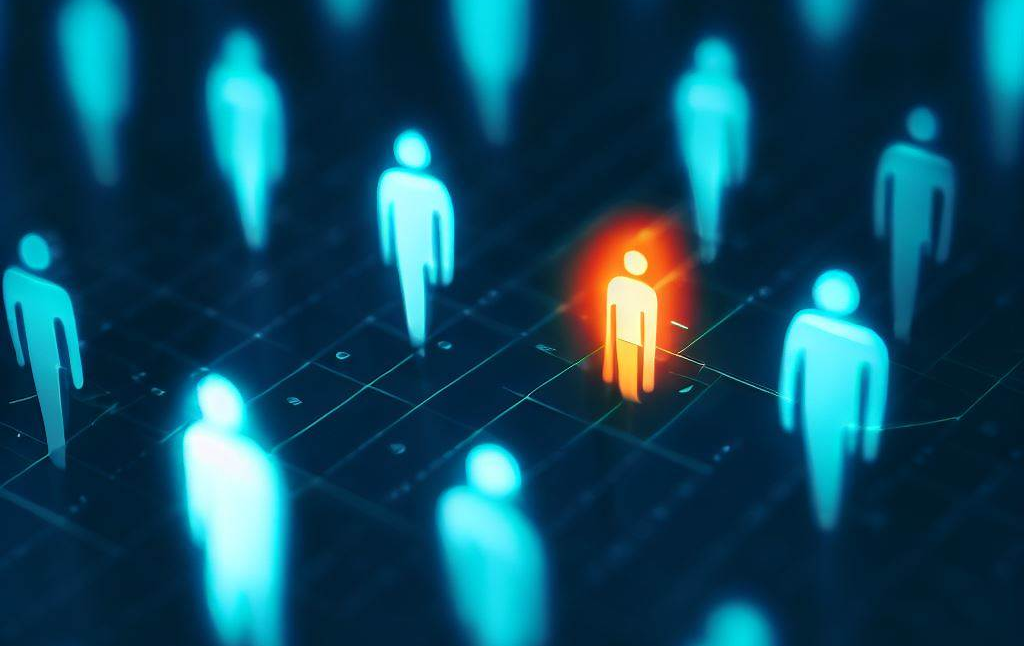
Outliers are extreme values that can have a significant impact on statistical analysis, leading to erroneous conclusions. It's essential to identify and handle outliers to obtain accurate results. Here's how to find and handle outliers in a dataframe.
Finding Outliers:
Visualizing the data: Visualizing the data is a great way to spot outliers. Scatter plots, box plots, and histograms can provide insights into the distribution of data and any potential outliers. For example, in a scatter plot, points that are far away from the main cluster can be considered outliers.
Statistical methods: There are several statistical methods to identify outliers, including Z-score and IQR (Interquartile Range). Z-scores calculate how far a value is from the mean. Any value that falls outside a certain Z-score threshold (typically 2 or 3) can be considered an outlier. IQR is another method that identifies the middle 50% of the data and calculates the range between the 25th and 75th percentiles. Values outside this range can be considered outliers.
Handling Outliers:
Once we've identified the outliers, we need to handle them correctly. Here are some common methods for handling outliers:
Removing outliers: One of the simplest methods to handle outliers is to remove them from the dataset. It should be used with caution, though, since it can lead to loss of information and bias.
Transforming data: Transforming data is another method to handle outliers. To reduce the impact of outliers, we can use mathematical functions like logarithms, square roots, or reciprocals. Transforming data can also help to normalize the distribution and make it easier to analyze.
Winsorization: Winsorization is a method that replaces extreme values with less extreme values. In this method, we replace the extreme values with the maximum or minimum values of the dataset. This method can be useful when we have a small number of outliers that are very different from the rest of the data.
Robust statistical methods: Robust statistical methods are designed to handle outliers and are less sensitive to extreme values. For example, instead of calculating the mean, we can use the median or mode, which are less affected by outliers.
Conclusion:
The accuracy of statistical analysis can be affected by outliers, so identifying and dealing with them is crucial. In this blog post, we explored different methods to find and handle outliers in a dataframe. Visualizing data, statistical methods, removing outliers, transforming data, Winsorization, and robust statistical methods are some of the common methods used to identify and handle outliers. However, the choice of method depends on the nature of the data and the analysis we're performing.
Subscribe to my newsletter
Read articles from Akash Kumar directly inside your inbox. Subscribe to the newsletter, and don't miss out.
Written by
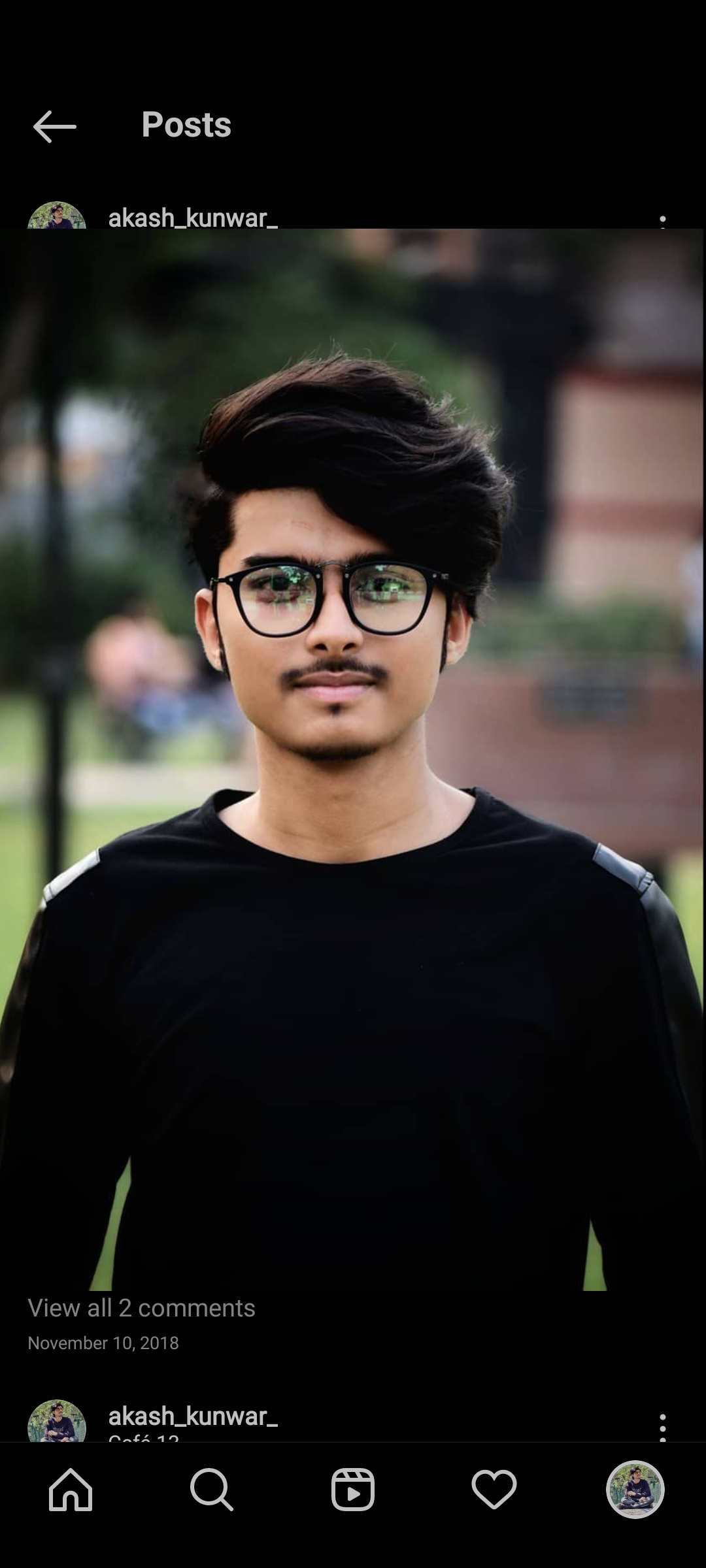
Akash Kumar
Akash Kumar
As a skilled data analyst and machine learning practitioner, I have worked on various projects in Kaggle using Python and other analytical tools. For me, working with data is not just a profession but a passion, and I enjoy exploring and discovering insights hidden in data sets. With expertise in Advanced Excel, Machine Learning, Power BI, Data Analysis, SQL, MongoDB, and Business Administration, I have a comprehensive understanding of data-driven decision-making, which enables me to deliver valuable insights and solutions to complex business problems. I hold a Bachelor's degree in Business Administration, with a focus on marketing and financial analysis, from Tula's Institute, and I am currently pursuing my Data Science course from IIT Madras, which has enhanced my technical skills in data science and machine learning. As a strong entrepreneur, I have a proven track record of delivering projects that meet or exceed expectations, and I am committed to continuous learning and growth to stay ahead in the field of data science. Thank you for taking the time to read my profile, and I look forward to connecting with like-minded professionals in the industry.