Setting up a Spark Cluster to Read Messages from Kafka(Beat~lytica part 2)
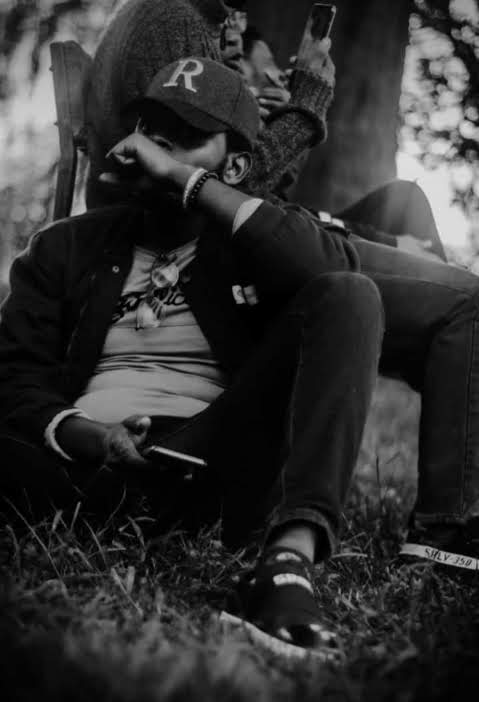
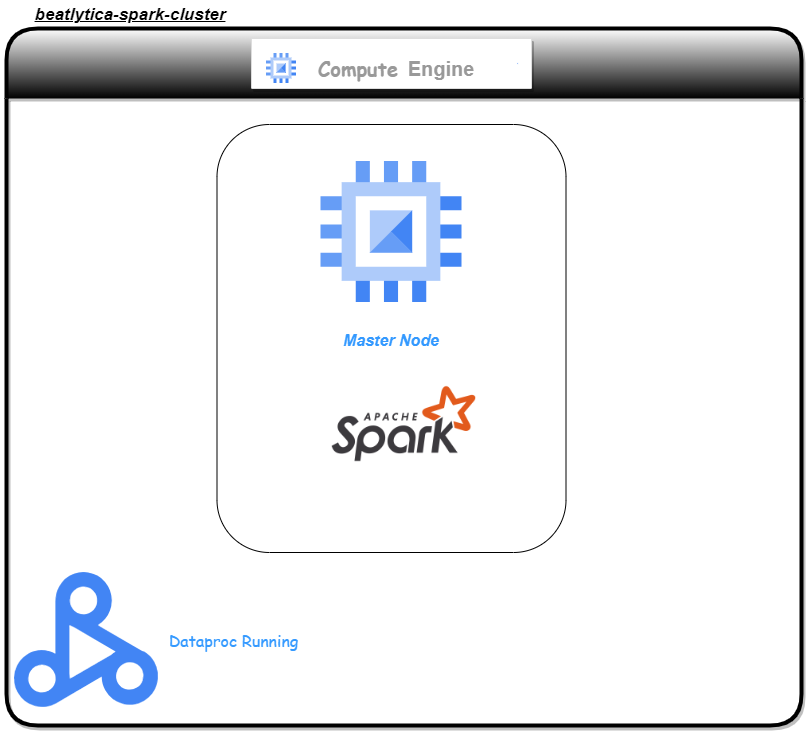
Welcome to my second installation of the Beatlytica Series.
Welcome back, fellow data enthusiasts! I'm thrilled to share with you the second installation of the Beatlytica blog series, where I dive into the exciting world of real-time data streaming and build a live dashboard powered by data streamed from Eventsim through Kafka topics.
If you missed the first installation, don't worry, you can catch up on it anytime, and I promise it's worth the read! In that post, I gave an overview of the Beatlytica project and shared how I used Eventsim to generate synthetic music data and stream it into Kafka topics.
In this blog, I'm going to be discussing the consumer of messages from Kafka topics. What is a consumer? A Kafka topic consumer is an application or process that reads messages from a specific topic in Kafka. In Kafka, messages are stored in topics, and a consumer subscribes to a topic and reads messages from it in real time. The consumer can read messages from multiple partitions of a topic in parallel, which allows for high throughput and scalability.
In my case, I chose Apache Spark to be my consumer, and the specific service I used was Spark-Streaming. Apache Spark is a powerful data processing framework used to process large datasets. The reason behind my choice is that I wanted to use the tool to apply my learnings, as well as the large community backing Apache Spark. This provided a sense of confidence in the event that I got stuck.
Prerequisites:
A Google Cloud Platform (GCP) account
A Kafka instance running on a virtual machine (VM) in GCP (set up previously)
A GCP bucket to store the data processed by Spark
Step 1: Setting up
Since the cluster was provisioned by running Terraform, I used VSCode to SSH into the instance by setting up a configuration file with the host, hostname (which is the public IP of the cluster), the user (in my case the cluster was running Debian, so I named the user Debian), and the path to the private RSA key that I generated from part 1.
Step 2: Access and Installation
I then cloned the code I had written for Spark streaming from my repo. The repo contains the code that installs the Spark streaming service into the cluster. I also set up the environment variables, which specified the external IP address of the Kafka VM instance and the name of my GCS bucket:
export KAFKA_ADDRESS=IP.ADD.RE.SS
export GCP_GCS_BUCKET=bucket-name
Note: You will need to set these environment variables every time you create a new shell session or stop/start your cluster.
The service is not running yet at this point, so to read messages, I ran "spark-submit
--packages org.apache.spark:spark-sql-kafka-0-10_2.12:3.1.2
stream_all_events.py" .
This command starts up the Spark streaming service and enables the messages from Kafka topics to be read/streamed through. The Python script governs how the data is fetched and where and how it will be stored.
Step 3: Running
The service will communicate with the Kafka instance via port 9092. At this point, to confirm if the installation was running okay, I logged in to the Google Cloud Storage bucket. And voila, there were new parquet files in my GCS bucket. These files were created for each topic that the spark streaming was reading from. In my case, the topics were:
listen_events
page_view_events
auth_events
Step 4: Rejoicing
Having confirmed that my data was streaming in I got too excited because my weekend project was working and I was halfway through my journey toward achieving a live dashboard.
Next steps:
to figure out orchestration tools such as airflow and dbt for applying large-scale transformations to the data.
I hope that this was a short and sweet read.
See you next time!
Subscribe to my newsletter
Read articles from Warui Wanjiru directly inside your inbox. Subscribe to the newsletter, and don't miss out.
Written by
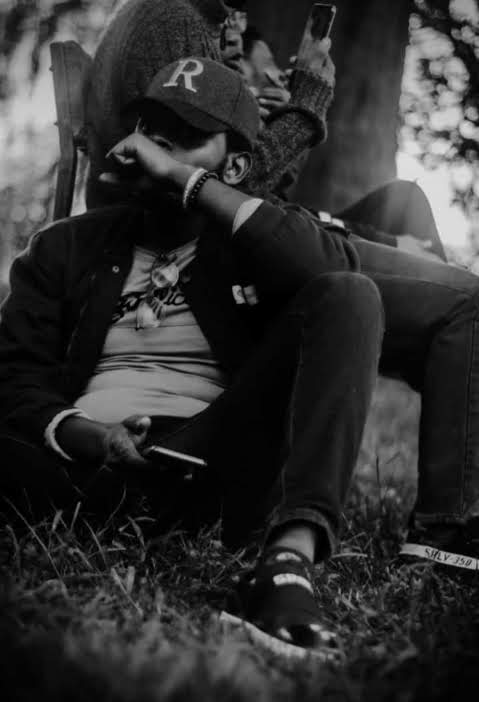
Warui Wanjiru
Warui Wanjiru
I am a passionate and highly motivated Junior Data Engineer, driven by my curiosity and eagerness to explore the vast world of data. With a solid foundation in data analytics, programming, and database management, I thrive on the challenge of extracting, cleaning, transforming, and visualizing data to uncover valuable insights. My journey in the realm of data engineering has exposed me to various programming languages and tools, including Python, SQL, and Tableau. However, I don't stop there—I am constantly seeking new knowledge and skills to stay ahead of the curve. Currently, I am immersing myself in the world of Rust, harnessing its speed and efficiency prowess. Embracing this new language allows me to tackle complex data engineering tasks with even greater efficiency and effectiveness. What truly sets me apart is my genuine enthusiasm for learning and taking on new challenges. I thrive in dynamic environments that push me to think creatively and find innovative solutions. With a solid understanding of data structures and algorithms, I relish the opportunity to dive into complex datasets, unearthing patterns and unlocking actionable insights. Above all, I am dedicated to making a real impact through data engineering. I believe in the power of data to drive transformative change and improve decision-making processes. By harnessing the power of data, I strive to empower organizations to make informed choices and achieve tangible results. Let's embark on this exciting journey together, where I can contribute my authentic enthusiasm, my thirst for knowledge, and my unwavering commitment to delivering exceptional data solutions.