Data-driven decisions and Metrics
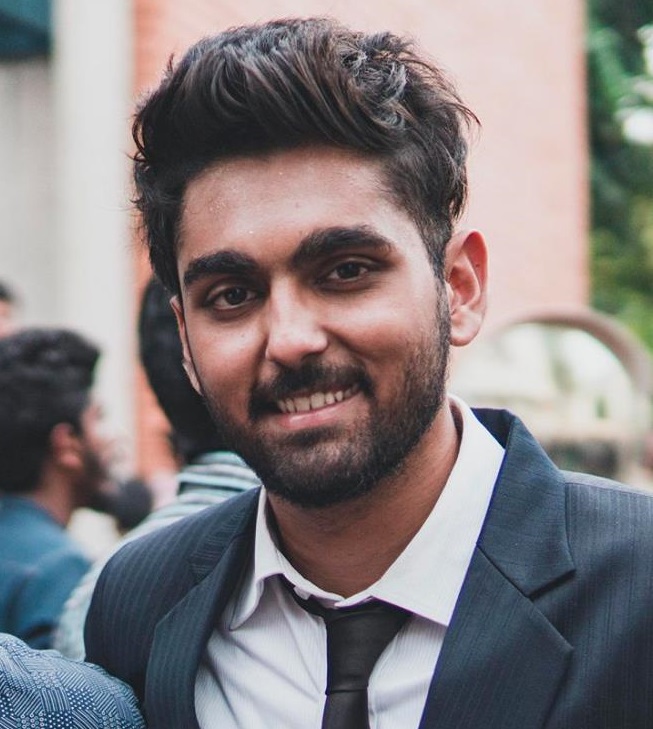
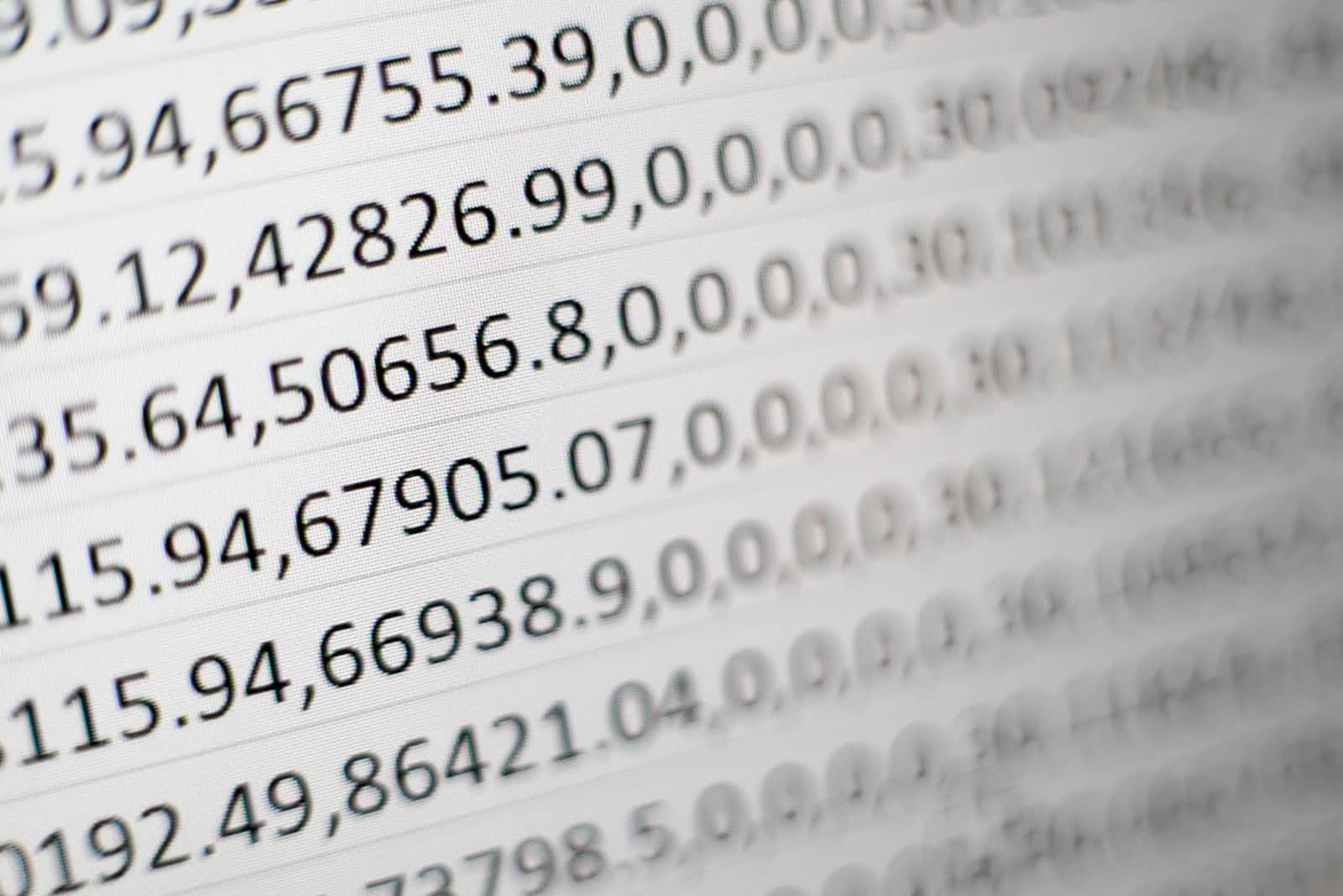
How do humans make decisions?
We are exposed to large and unique types of information in the process of growing up. Factors like the city, society, what kind of friends we had, what kind of family we had, macroeconomic situations we have seen, etc all create a model in our brains about how the world works. And since these influences are unique to everyone, these models also differ in one way or another.
When we make decisions on our own using our mental models, it's called intuition. And this can be right or wrong depending on what type of influences we were exposed to and how seriously we took those influences.
This type of decision-making is arguably the worst because we are not validating the correctness of our mental models and there is no feedback involved. An exception to this is however if the decision maker has good experience in the topic regarding which he/she is making the decision. For example, Roger Federer predicting the outcome of a tennis match or Warren Buffet predicting the value of a company.
A more consistently better way is to collaborate with people with different experiences. Through this, there is a much better chance that the people participating in this collaboration validate each other's intuitions. This would be more effective if the people involved had widely different experiences and also they communicate those experiences effectively. Although this type of decision-making is arguably better, it still has a lot of flaws. The main one is that it is still limited by the experiences of the group.
How do large companies make decisions?
Large corporations don't just rely on individuals' intuition. They try to gather data on a macro scale and make decisions based on that data. This is arguably better than just vanilla collaboration since it is not limited by the biases of the individuals involved. Moreover, since a lot of data can be collected, the information analyzed is more, which is better for fine-tuning the decisions.
The major flaw in these types of decisions is that good data is not easy to collect. There is a lot of data that is not recorded and even the data which is recorded, is not always analyzable. For example, quantifying how a user is feeling, the views of society on a particular matter, etc.
Also, due to the way data is collected, a lot of times the data itself has a bias and thus any intuition you derive from it could be biased as well. For example, you surveyed 100 people but only surveyed people from a particular region or age group or political affiliation. This would result in data that is not representative of the population. Although companies try very hard to remove biases in all steps of collecting data and analyzing it, some level of bias always remains.
Thus, In practice what ends up happening is sort of a hybrid form of decision-making where a group of people analyze large amounts of data and filter it using their intuitions. It's arguably better than all the other approaches not still not without flaws.
What are metrics?
Metrics are effectively just the data that the company is tracking. They can be anything and everything that can be tracked. For example, for a company like Netflix, some metrics that are tracked could be:
Number of logins per user per day
Sessions per week per user
Average session duration
Number of clicks of the home button per user per session
Avg time spent browsing
You can potentially track pretty much anything.
But tracking metrics is not free of cost. You have to pay for building the software that is tracking this data, for storing the data you are collecting, etc. Moreover, metrics can be useless as well. For example, for Netflix, a useless metric could be the number of times a user issues a down command over the user's lifetime.
So, companies try to track something which they think could come in handy in the future. Also, it depends on what kind of resources they have as well. For example, a big company would probably want to track more things than say a startup for the same feature/user flow simply because they can afford to do so.
What are success metrics?
When companies create new products or features, they do so with a specific goal in mind. For example, to increase the number of subscribers, to sell more products, to increase monthly active users etc.
Tracking metrics is a good way to judge the success or failure of the product. Based on the goal of the feature/product, metrics are prioritized based on how truly they reflect what the product/feature is meant for.
For example, for a mature product like Netflix, the company's goal could be to improve engagement and retain subscribers. In this case, they could track the number of hours watched per user per week and subscriber churn per month.
Based on the goal of the feature/product, some metrics would be given more importance than others and the product's success/failure will ultimately be judged based on these metrics.
The change in these metrics would also help the company in understanding what to build next since it would help them understand which metrics they want to improve.
For example, a couple of months ago, Netflix came up with a feature that allowed users to just start watching something instantly. This was aimed at reducing the time wasted by its users in selecting a movie/show. They probably build something like this because they were tracking the amount of time people were spending browsing and wanted to reduce it.
What are counter metrics?
Sometimes it's possible that even when a feature is successful as seen from its success metrics, it affects some other feature or some other metric in a negative manner.
Thus to keep track of something like this, companies also track metrics that might get negatively affected as a side effect of the feature. These metrics are called counter metrics.
For Example
Suppose Netflix decides to run a marketing campaign aimed at increasing the number of subscribers.
Some potential success metrics for this could be the number of new subscribers per month, the Average number of hours streamed per day, and the Percentage of users who renew their subscriptions.
And some potential counter metrics for this could be Average cost per acquisition (CPA), Average cost per hour streamed (CPH) and Number of negative reviews for original content.
Subscribe to my newsletter
Read articles from Utkarsh Bindal directly inside your inbox. Subscribe to the newsletter, and don't miss out.
Written by
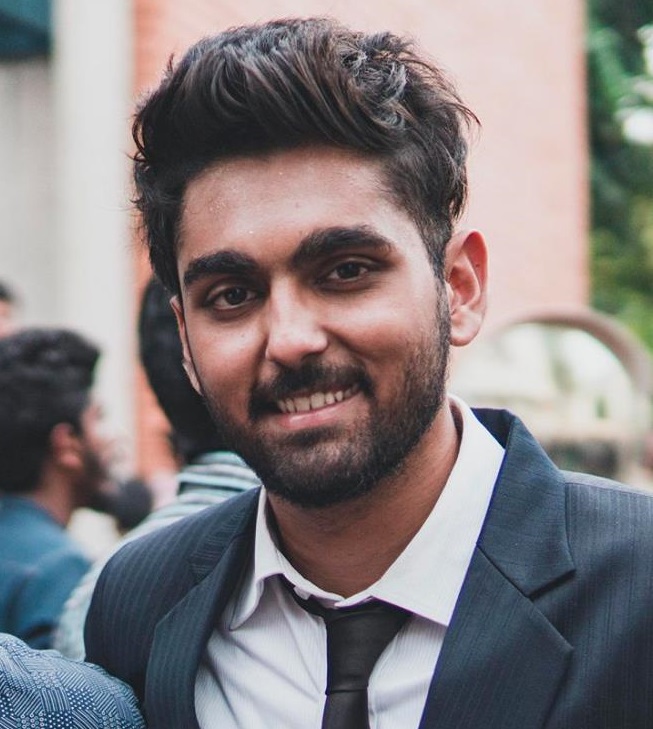