Types of normalization in Machine learning
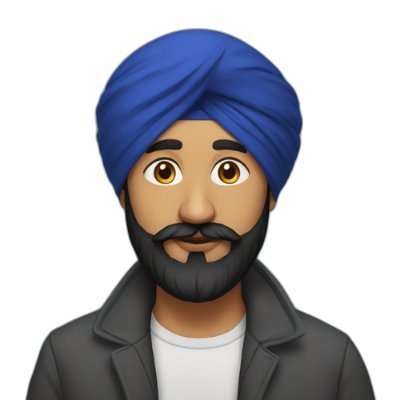
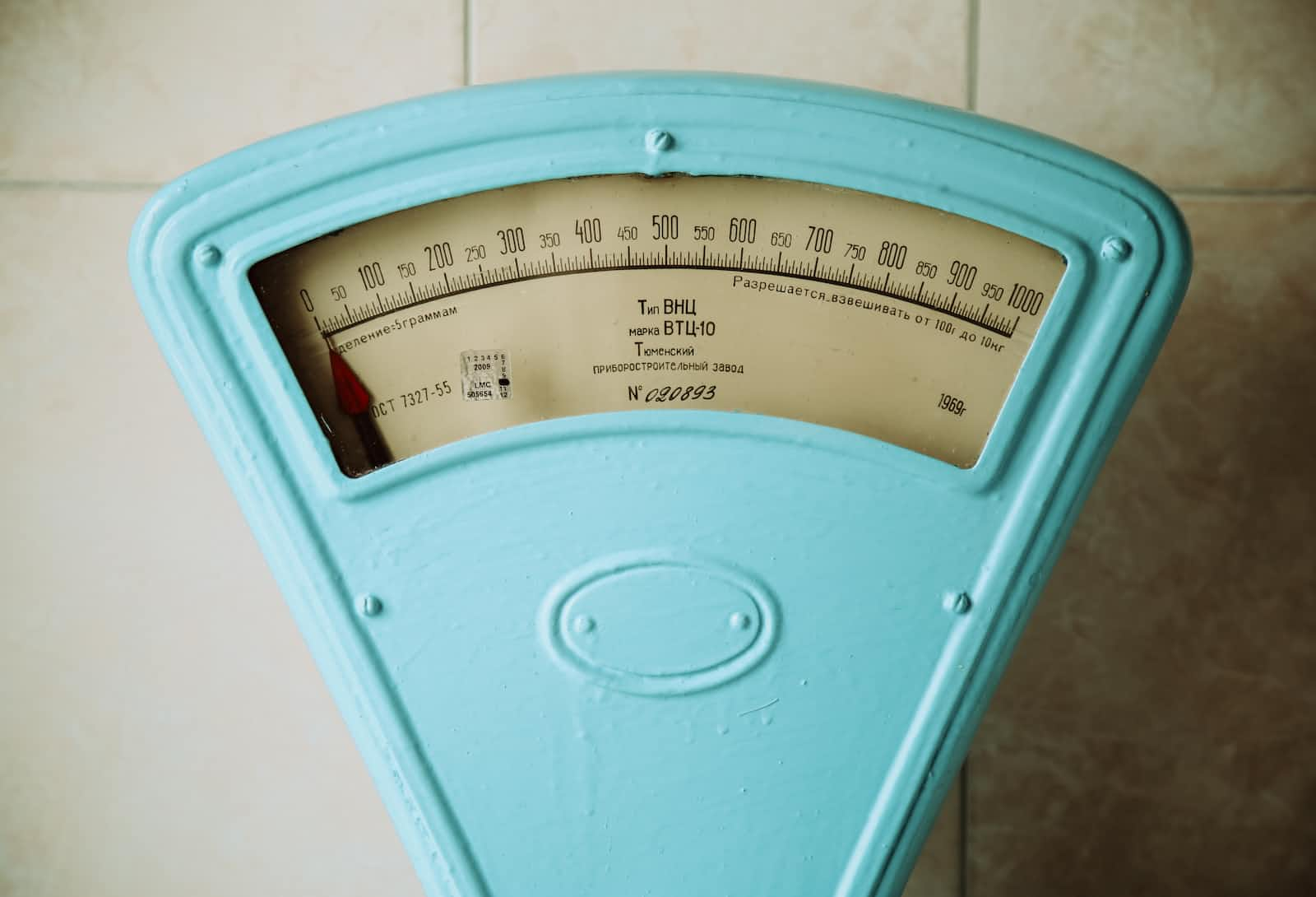
I hope you are doing great, this blog is basically a continuity of previous blog "" and in this blog as I promised we will talk about normalization in detail and we will see what are the various types of normalization and when to use which type. So without any further delay let's get started.
What is normalization and when to use ?
Normalizatoin is basically a feature scaling technique which is used in those kind of scenarios where we are not aware about the distribution of data or when our algorithm doesn't make any assumption about underlying data distribution and after applying normalization on our data or on some particular feature the values get transformed into new values ranging between 0 to 1.
Types of normalization
If you would see the scikit documentation for normalization you would find that there are various types of normalization, but for around 95% of the time you would use only the 4 main types of normalization. The 4 types of normalization which we will discuss in today's blog are: Min max normliaztion, Mean normalization, Max absolute normalization and robust scaling.
Min-max normalization
Min-max normalization is a data normalization technique which used to scale numeric values to a specific range, typically between 0 and 1. The process involves subtracting the minimum value from each data point and dividing it by the difference between the maximum and minimum values. Mathematicall it is defined as
Mean normalization
Mean normalization is another data normalization technique that is used to scale numeric values. It involves subtracting the mean (average) of the dataset from each data point and then dividing it by the range of the data i.e maximum - minmum value.
Max absolute scaling
Maximum Absolute Scaling is a data scaling technique used to normalize numerical data by dividing each data point by the maximum absolute value in the dataset. It scales the data to a range between -1 and 1. Mathematicall defined as
Robust scaling
Robust Scaling, also known as Robust Standardization or Median and MAD Normalization, is a data scaling technique used to normalize numerical data while being robust to outliers. It is particularly useful when dealing with datasets that contain extreme values that can significantly impact the scaling process.
Short Note
I hope you good understanding of what is normalization, what are the various type fo normalization and when to apply which normalization technique. So if you liked this blog or have any suggestion kindly like this blog or leave a comment below it would mean a to me.
Subscribe to my newsletter
Read articles from Yuvraj Singh directly inside your inbox. Subscribe to the newsletter, and don't miss out.
Written by
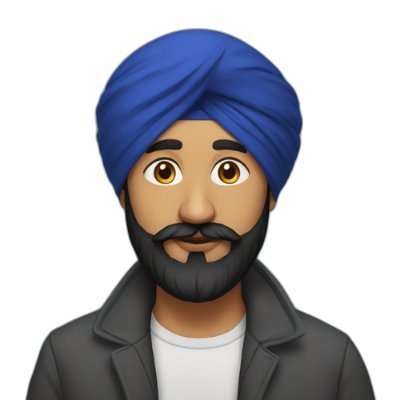
Yuvraj Singh
Yuvraj Singh
With hands-on experience from my internships at Samsung R&D and Wictronix, where I worked on innovative algorithms and AI solutions, as well as my role as a Microsoft Learn Student Ambassador teaching over 250 students globally, I bring a wealth of practical knowledge to my Hashnode blog. As a three-time award-winning blogger with over 2400 unique readers, my content spans data science, machine learning, and AI, offering detailed tutorials, practical insights, and the latest research. My goal is to share valuable knowledge, drive innovation, and enhance the understanding of complex technical concepts within the data science community.