Azure Data Factory with local SQL server database

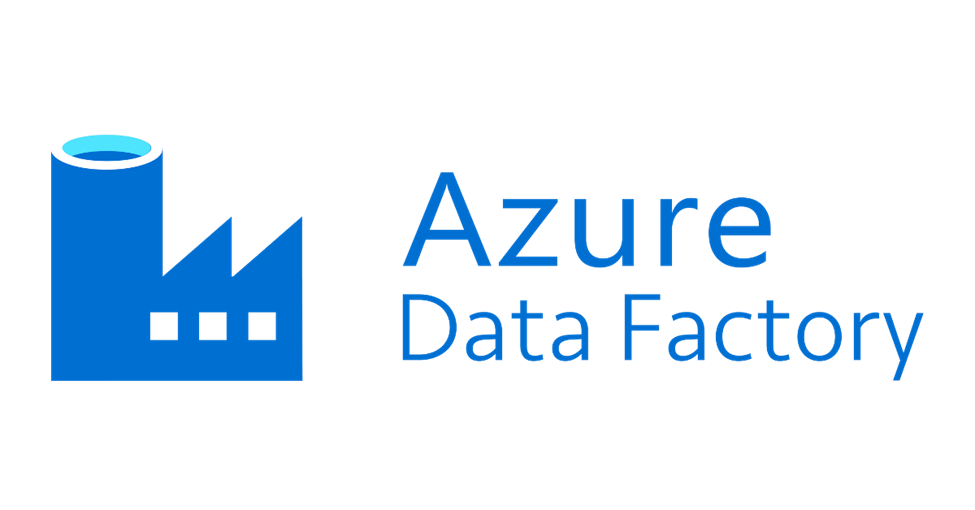
Follow these steps to set up and use Azure Data Factory with a local SQL Server database:
- Install and configure a Self-hosted Integration Runtime (IR)
- ADF uses the Integration Runtime (IR) to access on-premises resources like your local SQL Server. To set up a self-hosted IR, follow the instructions in the official documentation.
- Create a SQL Server Linked Service in ADF
- In ADF, Linked Services define the connection settings to your data sources. To create a SQL Server Linked Service:
- a. Sign in to the Azure portal. b. Navigate to your Azure Data Factory. c. Go to the “Author & Monitor” tab. d. Click the “Author” tab on the left-hand side. e. Click the “Connections” (lightning bolt) icon at the bottom. f. Click “New” and search for “SQL Server”. g. Fill in the required fields, such as the server name, authentication type, username, and password. For “Connect via integration runtime,” choose the self-hosted IR you created earlier. h. Test the connection, and if successful, click “Create.”
- Create datasets for source and destination
- In ADF, datasets are used to represent the structure of the data within the Linked Services. Create a dataset for your local SQL Server database (source) and another dataset for the destination, such as Azure SQL Database or Azure Blob Storage.
- Create a data movement pipeline
- Now you can create a pipeline to move and process data from your local SQL Server to the destination. In the pipeline, use activities like “Copy Data” or “Mapping Data Flows” to move and transform the data as needed.
- Trigger the pipeline
- After creating the pipeline, you can manually trigger it or set up a schedule to run it at specific intervals. Use the “Trigger Now” button to run the pipeline immediately, or create a new trigger with the desired schedule.
- Monitor the pipeline
- In the “Monitor” tab in ADF, you can track the progress and status of your pipelines, view activity run details, and troubleshoot any issues that may occur.
For a more detailed guide, refer to the official Azure Data Factory documentation.
Subscribe to my newsletter
Read articles from Ahmed Sayed directly inside your inbox. Subscribe to the newsletter, and don't miss out.
Written by

Ahmed Sayed
Ahmed Sayed
An industrious professional specializing in data engineering for more than 13 years, I am currently leading the data engineers' team with a comprehensive background in various data stack technologies. My prowess extends across Hadoop, SQL, Spark, Python, Airflow, Scala, Java, Talend, SSIS, Data Stage, PDI, Google Cloud, and Azure. Data Streaming is a domain that particularly enthralls me, and I have acquired significant expertise in using Kafka for the same. As a core competency, I specialize in designing and building robust data architectures on diverse platforms, be it on-premises or cloud-based platforms like Azure, Google Cloud Platform, and AWS. Over the years, I've provided numerous solutions in data migration and automation, effortlessly crafting data models and designing warehouses using dimensional models, data vaults, and data marts. A keen advocate for modern lake houses, I have substantial experience in Data Lake designs and implementations. Proficient in a wide array of databases such as SQL Server, PostgreSQL, MySQL, Oracle, Netezza, Cassandra, Mongo, Azure NoSQL, Vantage, Gemin, Neo4j, Arango, Vector, Redshift, Big Query, and many others, I also excel in ensuring data security and compliance across all my projects. My skills extend to Microsoft Azure, where I've built data pipelines, created real-time streaming data solutions, and worked extensively with Azure Synapse, Azure Data Lake, Azure Blob Storage, Azure Databricks, and Azure Stream Analytics. Likewise, I am familiar with AWS EC2, Redshift, Glue, Athene, and GCP Data Flow, Big Query. In addition to this, I have developed API solutions, both RESTful and GraphQL using Python Flask, Django, and Fast API. I've also crafted data solutions for web applications and worked with AI solutions like GPT, Azure Cognitive, and Google NLP, including fine-tuning. Experienced in building and developing custom ML and NLP Models, I am proficient in Process Automation RPA, utilizing tools like UIpath. The intersection of data, technology, and problem-solving fuels my passion, and I am consistently striving to push boundaries and deliver solutions that exceed expectations. My mission is to leverage my data engineering skills and expertise to create innovative and scalable solutions that address the complex challenges and opportunities in many industries. I enjoy collaborating with cross-functional teams of data scientists, product managers, user experience researchers, and software engineers to understand data needs and deliver on them. I am always eager to stay up-to-date with new technologies