Stats in Data Science
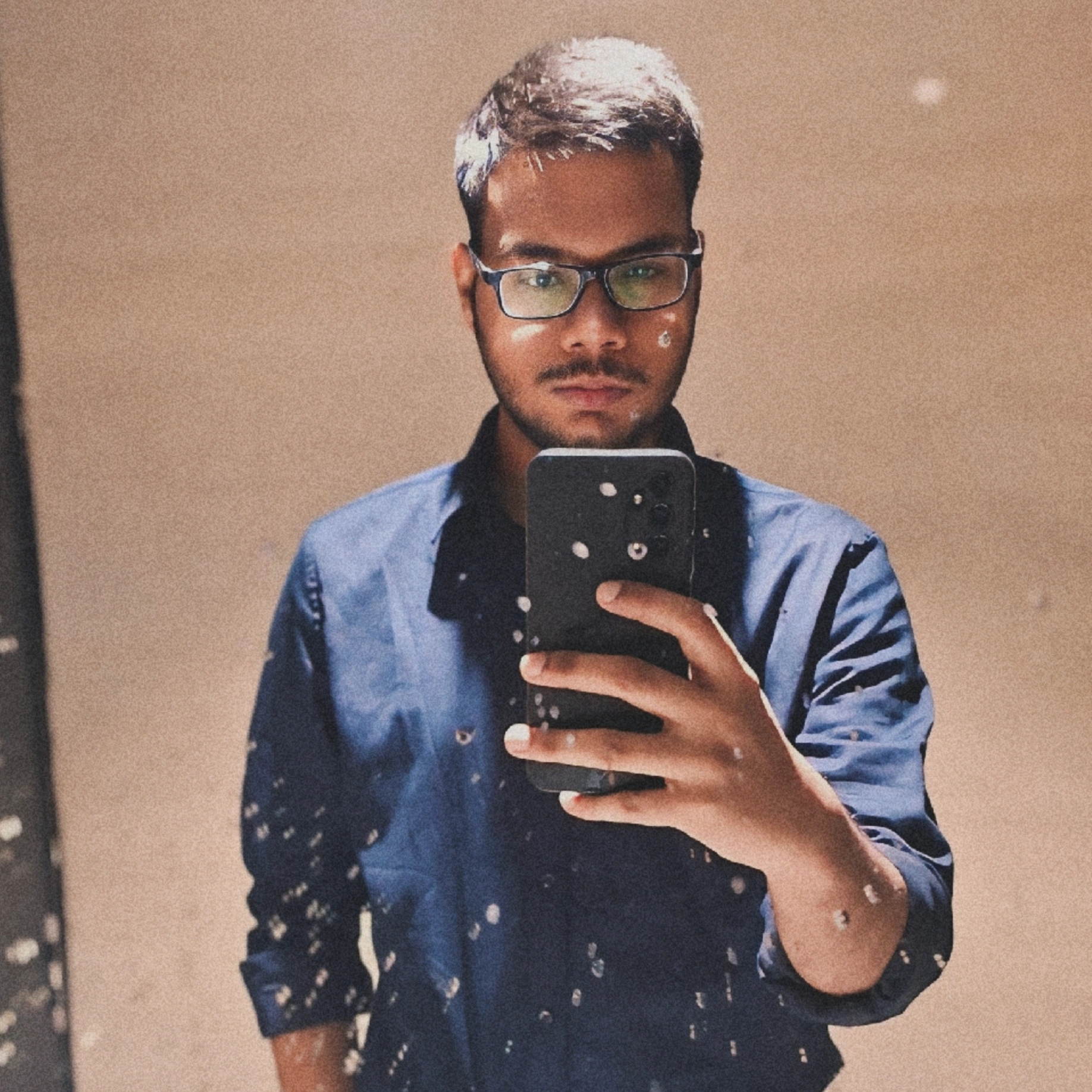
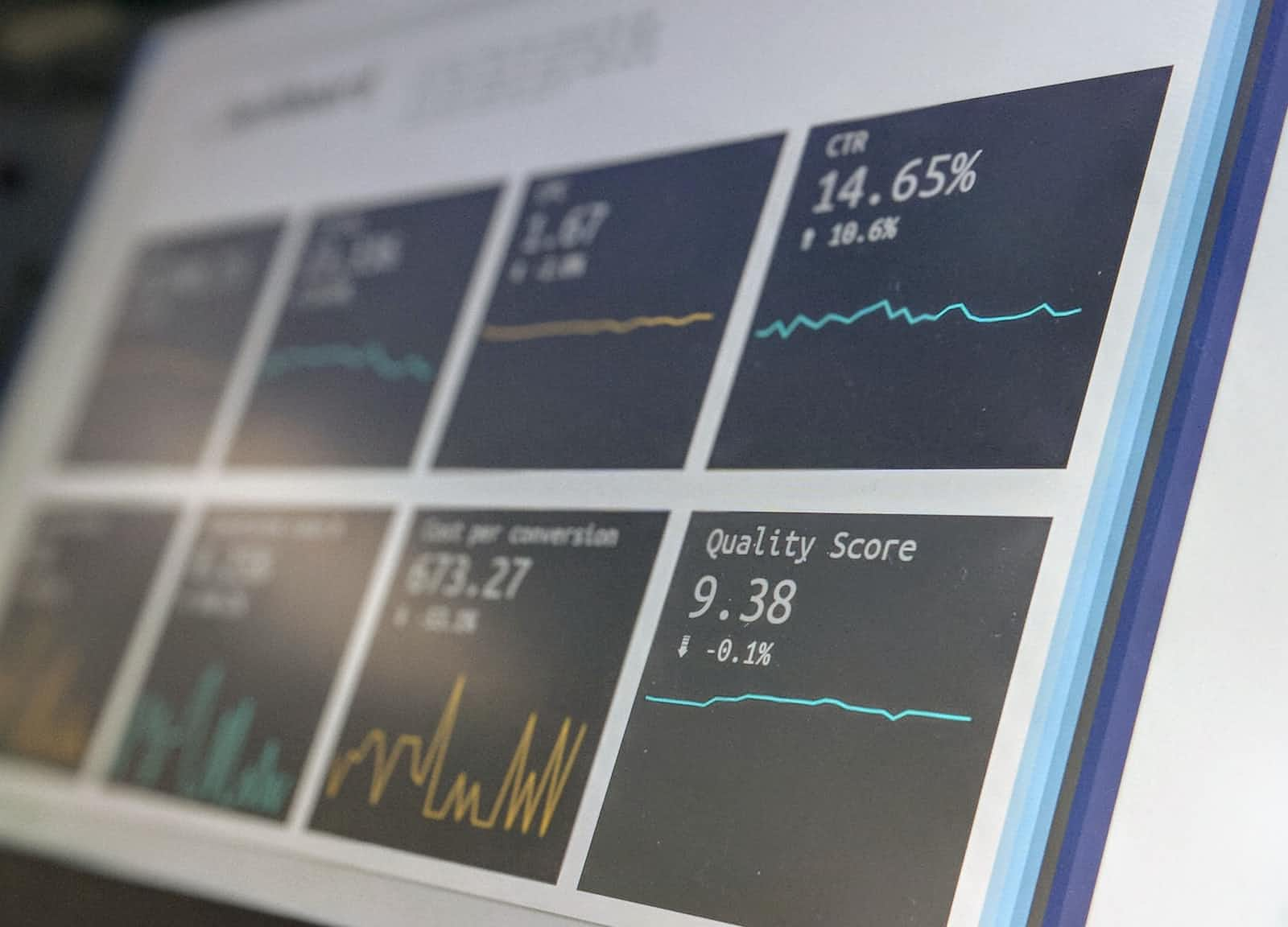
The Importance of Statistics in the Domain of Data Science
By Anshuman Sinha
Note- In this article, I broadly explained the use of statistics without going much in depth
As the world becomes increasingly data-driven, the field of data science has emerged as a powerful and transformative discipline. At its core, data science involves extracting valuable insights and knowledge from vast amounts of data. However, this process is not a straightforward one. It requires a robust foundation in statistics, making statistics a fundamental pillar in the domain of data science. In this article, we will explore the crucial role of statistics in data science and its significance in transforming raw data into meaningful information.
Data science revolves around three main components: data collection, data analysis, and decision-making. Statistics plays a pivotal role in each of these steps, allowing data scientists to make informed choices and draw reliable conclusions. First and foremost, data collection demands a clear understanding of sampling techniques, experimental design, and data quality control. Without proper statistical methods, data collection can lead to biased or unrepresentative results, rendering subsequent analyses meaningless.
Once the data is collected, the analysis phase begins, and this is where statistics truly shines. Through various statistical methods, data scientists can explore patterns, relationships, and trends in the data. Descriptive statistics, such as mean, median, and standard deviation, provide a concise summary of the data, while inferential statistics, including hypothesis testing and confidence intervals, enable researchers to make predictions and generalizations about the larger population.
Furthermore, statistics plays a crucial role in data visualization. Data visualization allows data scientists to present their findings in a visually compelling and easy-to-understand manner. Through graphs, charts, and other visual representations, complex data can be communicated effectively to stakeholders and decision-makers. However, creating meaningful visualizations requires a deep understanding of statistical principles to accurately portray the data's underlying patterns and avoid misinterpretations.
In the realm of machine learning, statistics serves as the backbone of algorithm development and model evaluation. Techniques like regression analysis, decision trees, and clustering are rooted in statistical concepts, enabling data scientists to build predictive models that can be applied to real-world scenarios. Moreover, statistical evaluation metrics, such as accuracy, precision, recall, and F1 score, help in assessing model performance and identifying areas of improvement.
The importance of statistics in data science extends beyond mere data analysis. It also influences the decision-making process. Making data-driven decisions is at the heart of data science, and this involves understanding the uncertainty associated with data and the potential risks involved. Statistical methods provide a solid framework to quantify uncertainty and make decisions based on probabilities and confidence levels, mitigating the impact of biases and errors.
In the current era of big data, statistics becomes even more critical. With an overwhelming volume of data generated every second, data scientists face the challenge of extracting valuable information from the noise. Statistical techniques, such as dimensionality reduction, data sampling, and outlier detection, help in dealing with large datasets efficiently, enabling data scientists to focus on the most relevant information.
In conclusion, statistics is the backbone of data science, providing the necessary tools and methodologies to convert raw data into meaningful insights. From data collection to analysis and decision-making, statistical knowledge ensures accuracy, validity, and reliability throughout the entire data science process. As data continues to shape our world, understanding statistics becomes ever more crucial for aspiring data scientists like me, Anshuman Sinha, to contribute effectively to this transformative field and unlock the true potential of data-driven decision making.
Subscribe to my newsletter
Read articles from Anshuman Sinha directly inside your inbox. Subscribe to the newsletter, and don't miss out.
Written by
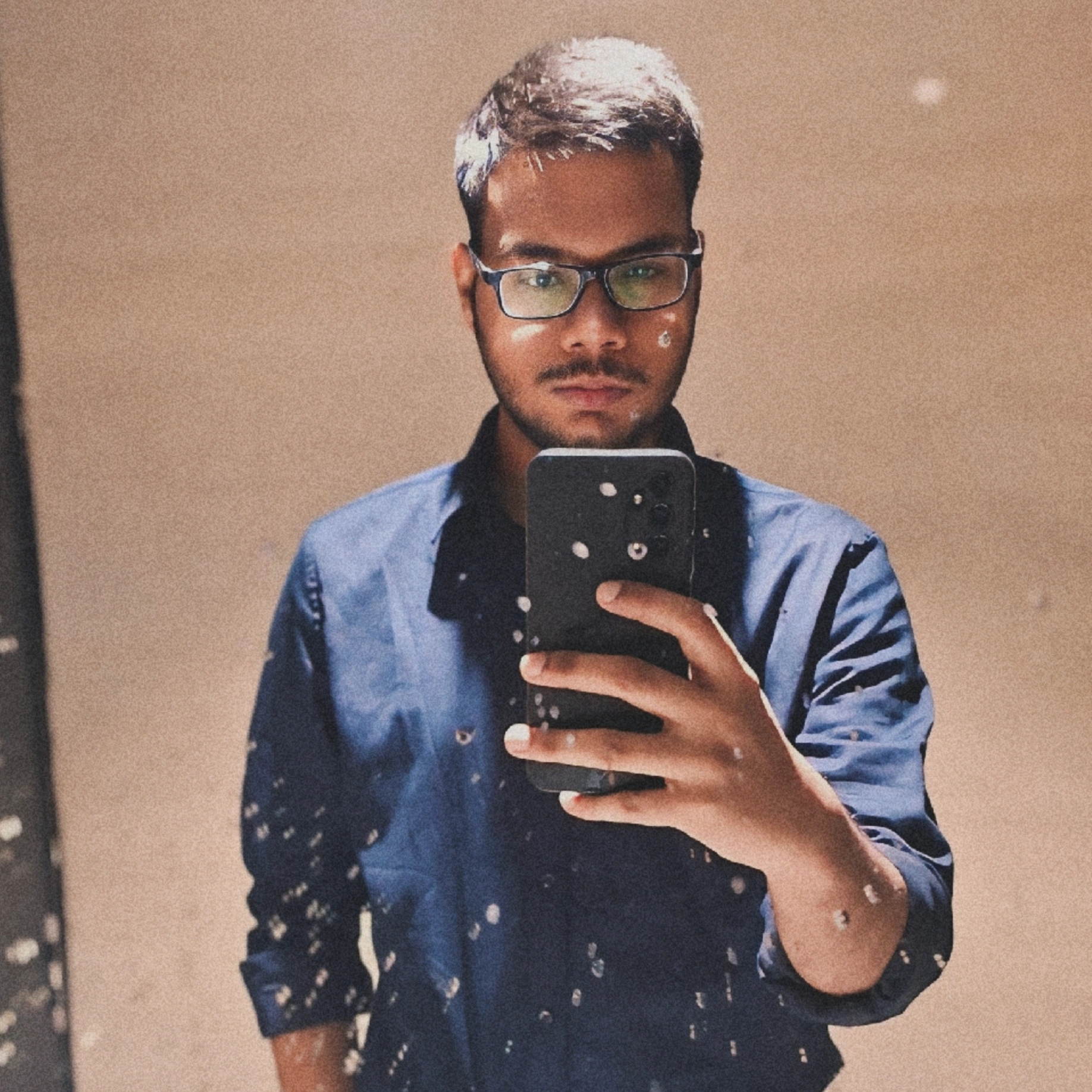
Anshuman Sinha
Anshuman Sinha
Software Developer who previously worked as an SDE Intern at a consulting firm and as a Data Science intern at an IT Firm. Currently pursuing BCA from Amity University Patna.