A Comprehensive Overview of Generative AI Technology
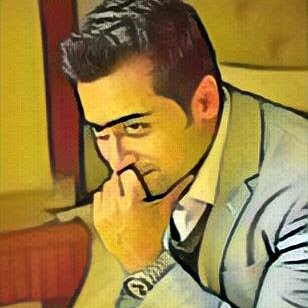
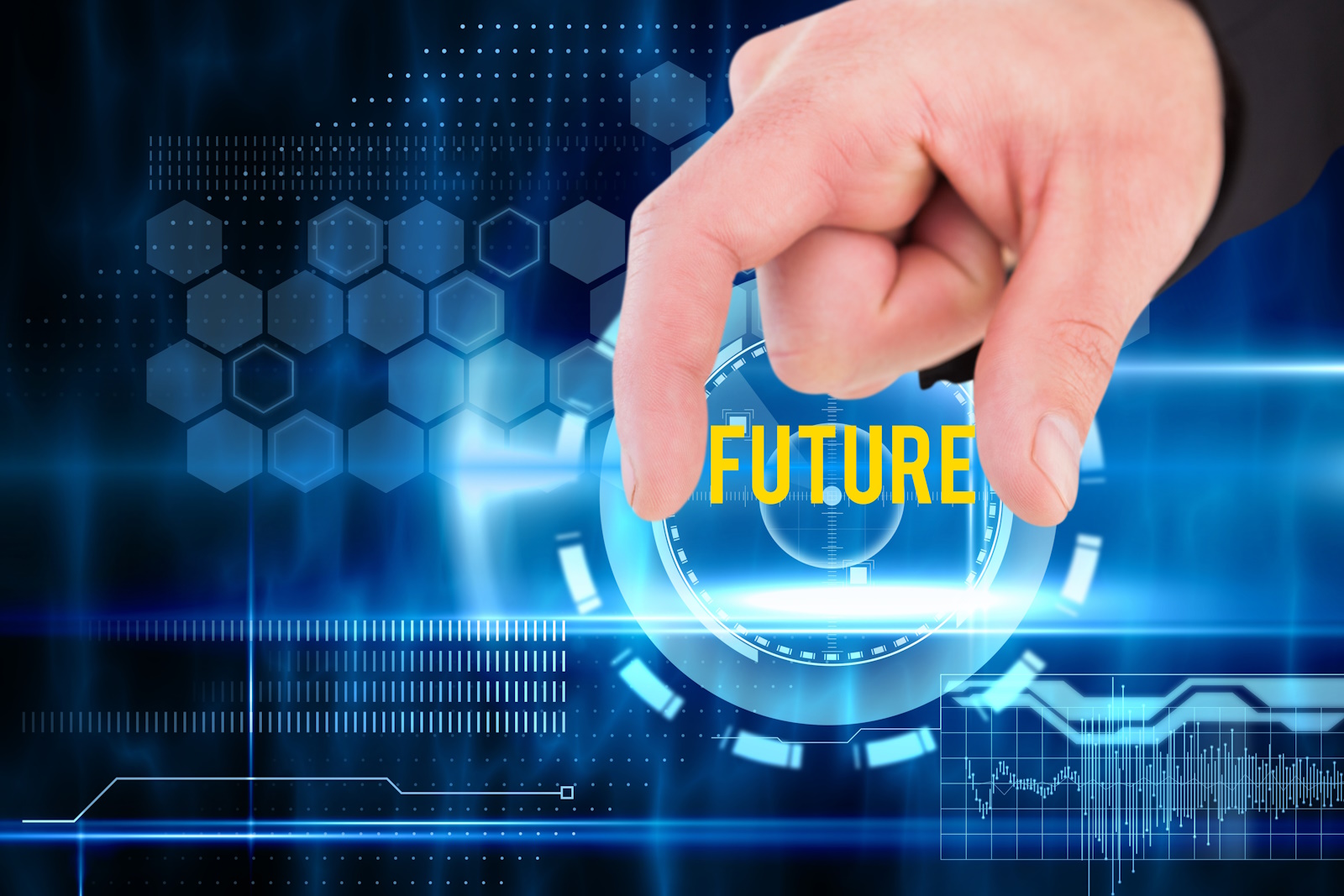
Generative AI Technology is a revolutionary subset of AI (artificial intelligence) that has captured the imagination of developers. Unlike traditional AI techniques that are restricted to rule-based decision-making & pattern recognition, Generative AI Technology has the unique capability of generating new content, simulating human imagination, and realistic outputs. This technology holds immense security across diverse domains, from creative arts and entertainment to healthcare and robotics. Here, we will explore the underlying principles, apps, and challenges of Generative AI Technology, clearing light on its transformative possibility.
What is Generative AI Technology?
Generative AI Technology is a branch of artificial intelligence that concentrates on training models to generate data that reaches a given information dataset. It leverages advanced machine learning algorithms, specifically deep learning architectures like Generative Adversarial Networks (GANs) and Variational Autoencoders (VAEs) to create new content from scratch. Unlike traditional AI, which depends on predefined rules or training on labeled data for classification tasks, Generative AI Technology can produce original and meaningful outputs without detailed instructions.
1. Generative Adversarial Networks (GANs)
One of the most famous and influential techniques in Generative AI Technology is Generative Adversarial Networks (GANs), proposed by Ian Goodfellow in 2014. GANs consist of two neural networks: the generator and the discriminator. The generator is tasked with creating synthetic data models, while the discriminator's role is to differentiate between real & generated data.
During training, the generator continually improves its ability to produce authentic-looking data, while the discriminator enhances its capacity to identify real data from fake. This adversarial dynamic results in a cat-and-mouse game where both networks improve iteratively until the generator can produce high-quality data indistinguishable from the real dataset. GANs have been used to create art, generate realistic images, and even produce deepfakes.
2. Variational Autoencoders (VAEs)
Variational Autoencoders (VAEs) are another essential Generative AI Technology technique that enables the creation of data samples by learning a probabilistic representation of the input data. VAEs work by encoding the input data into a lower-dimensional latent space and then decoding it back into the original data space. This process allows VAEs to generate new data by sampling from the learned latent space distribution.
Unlike GANs, VAEs are not adversarial models but rely on variational inference and the reparameterization trick to optimize the latent space. VAEs find applications in image generation, drug discovery, and natural language processing tasks.
Applications of Generative AI Technology
Generative AI Technology has a wide range of applications across diverse domains. Some of the key areas where it is making a significant impact include:
a. Creative Arts and Entertainment: Generative AI Technology has unlocked new possibilities in creative expression. Artists and designers use generative models to produce novel artwork, music compositions, and even poetry. It has opened avenues for innovative storytelling and interactive experiences in video games and virtual reality.
b. Image and Video Synthesis: GANs have demonstrated their potential in generating realistic images and videos. This technology has applications in generating high-resolution images, video synthesis for virtual environments, and enhancing low-quality images.
c. Drug Discovery: In the pharmaceutical industry, Generative AI Technology is used to identify and design new drug candidates. By analyzing chemical structures and biological properties, AI models can propose potential molecules that may have therapeutic value, streamlining the drug discovery process.
d. Natural Language Processing (NLP): Generative models have shown remarkable progress in language generation tasks. From chatbots that can engage in meaningful conversations to language translation and text summarization, Generative AI Technology has enhanced various NLP applications.
e. Healthcare: In healthcare, Generative AI Technology has found applications in medical image synthesis, pathology image analysis, and drug design, revolutionizing diagnostics and personalized medicine. These AI models are trained on high-quality medical data which are labeled using DICOM labelling tools.
f. Robotics: Generative AI Technology is instrumental in teaching robots to learn from limited data and interact intelligently with their environment. It aids in creating more adaptive and autonomous robots for various industries.
Ethical and Social Implications
While Generative AI Technology presents exciting possibilities, it also raises ethical concerns. The ability to create realistic deepfakes can be misused for spreading misinformation, fraud, or manipulating public opinion. Moreover, Generative AI Technology can potentially generate biased content, reinforcing existing stereotypes or promoting harmful narratives.
To address these challenges, researchers and policymakers must work together to develop robust AI ethics frameworks, data privacy regulations, and techniques for detecting and mitigating malicious uses of Generative AI Technology.
Challenges and Future Directions
Despite its rapid advancements, Generative AI Technology faces several challenges that need to be addressed for widespread adoption and responsible use. Some of these challenges include:
a. Training Instability: Training generative models like GANs can be unstable, leading to mode collapse or low-quality outputs. Researchers are continuously working on improving training techniques to address these issues.
b. Data Bias: Generative models can inherit biases present in the training data, leading to the generation of biased outputs. Efforts are being made to develop methods that reduce bias in Generative AI Technology.
c. Interpretability: Understanding how Generative AI Technology arrives at its outputs is a complex problem. Improving model interpretability is crucial for gaining trust and making informed decisions based on AI-generated content.
d. Computational Resources: Training sophisticated generative AI models requires significant computational power and resources. As technology progresses, addressing these resource constraints will be essential for wider adoption.
In the future, we can expect Generative AI Technology to continue pushing boundaries, enabling novel applications, and enriching human experiences. With responsible research and ethical guidelines, we can harness the true potential of Generative AI Technology for the betterment of society.
Conclusion
Generative AI Technology represents a paradigm shift in artificial intelligence (AI), empowering devices to create & innovate. Techniques like GANs & VAEs have transformed diverse industries, from art & entertainment to healthcare & robotics. However, as with any disruptive technology, Generative AI Technology comes with its challenges, such as ethical implications and data biases. By understanding these challenges and addressing them responsibly, we can unlock the transformative potential of Generative AI Technology and usher in a new era of creativity, innovation, and progress.
Subscribe to my newsletter
Read articles from Arjun Solanki directly inside your inbox. Subscribe to the newsletter, and don't miss out.
Written by
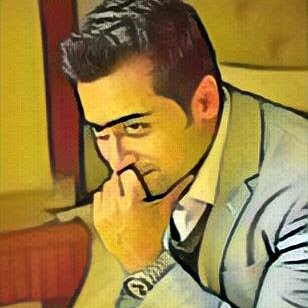
Arjun Solanki
Arjun Solanki
I am a Business Growth Strategist at a Leading Software Development Company. I have experience in developing and executing digital strategies for large global brands in a variety of business verticals. Apart from working on a long-lasting relationship with customers and boost business revenue, I am also interested in sharing my knowledge on various technologies and their influence on businesses through effective blog posts and article writing.