Hadoop #Split-Brain Scenario & #Fencing
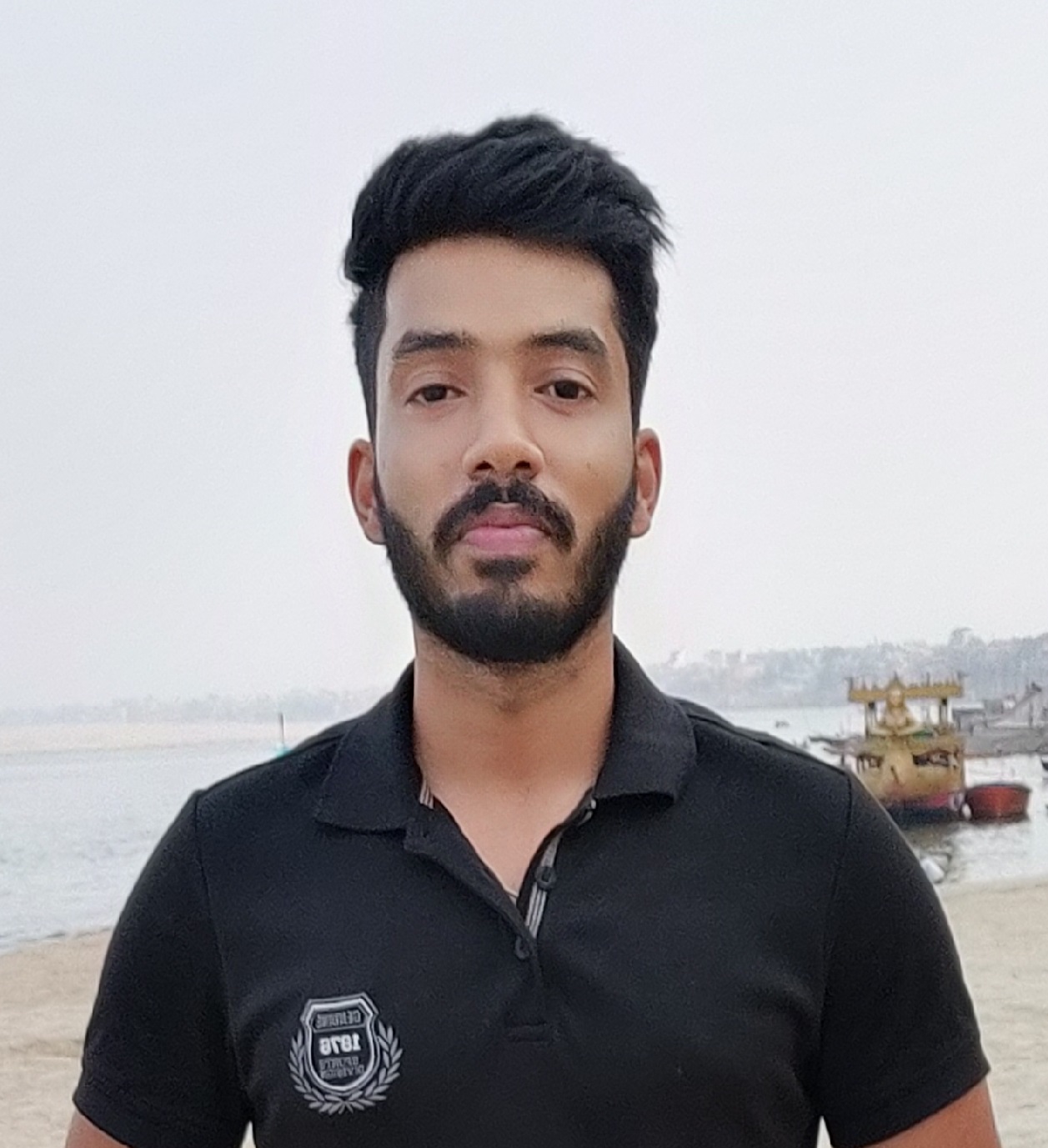
Let's #hadoop
๐ ๐๐ก๐๐ญ ๐ข๐ฌ #๐๐ฉ๐ฅ๐ข๐ญ ๐๐ซ๐๐ข๐ง ๐๐๐๐ง๐๐ซ๐ข๐จ ๐๐ง๐ #๐๐๐ง๐๐ข๐ง๐ ๐ข๐ง ๐๐๐๐จ๐จ๐ฉ?
โ ๐๐ฏ ๐ต๐ฉ๐ฆ ๐ค๐ฐ๐ฏ๐ต๐ฆ๐น๐ต ๐ฐ๐ง ๐ฅ๐ช๐ด๐ต๐ณ๐ช๐ฃ๐ถ๐ต๐ฆ๐ฅ ๐ด๐บ๐ด๐ต๐ฆ๐ฎ๐ด, ๐ช๐ฏ๐ค๐ญ๐ถ๐ฅ๐ช๐ฏ๐จ ๐๐ข๐ฅ๐ฐ๐ฐ๐ฑ ๐ค๐ญ๐ถ๐ด๐ต๐ฆ๐ณ๐ด, ๐ข "#split_brain ๐ด๐ค๐ฆ๐ฏ๐ข๐ณ๐ช๐ฐ" ๐ข๐ฏ๐ฅ "#fencing" ๐ข๐ณ๐ฆ ๐ณ๐ฆ๐ญ๐ข๐ต๐ฆ๐ฅ ๐ค๐ฐ๐ฏ๐ค๐ฆ๐ฑ๐ต๐ด ๐ต๐ฉ๐ข๐ต ๐ฅ๐ฆ๐ข๐ญ ๐ธ๐ช๐ต๐ฉ ๐ต๐ฉ๐ฆ ๐ช๐ด๐ด๐ถ๐ฆ ๐ฐ๐ง ๐ฎ๐ข๐ช๐ฏ๐ต๐ข๐ช๐ฏ๐ช๐ฏ๐จ ๐ฅ๐ข๐ต๐ข ๐ค๐ฐ๐ฏ๐ด๐ช๐ด๐ต๐ฆ๐ฏ๐ค๐บ ๐ข๐ฏ๐ฅ ๐ข๐ท๐ฐ๐ช๐ฅ๐ช๐ฏ๐จ ๐ฅ๐ข๐ต๐ข ๐ค๐ฐ๐ณ๐ณ๐ถ๐ฑ๐ต๐ช๐ฐ๐ฏ ๐ช๐ฏ ๐ค๐ข๐ด๐ฆ ๐ฐ๐ง ๐ฏ๐ฆ๐ต๐ธ๐ฐ๐ณ๐ฌ ๐ฑ๐ข๐ณ๐ต๐ช๐ต๐ช๐ฐ๐ฏ๐ด ๐ฐ๐ณ ๐ค๐ฐ๐ฎ๐ฎ๐ถ๐ฏ๐ช๐ค๐ข๐ต๐ช๐ฐ๐ฏ ๐ง๐ข๐ช๐ญ๐ถ๐ณ๐ฆ๐ด ๐ข๐ฎ๐ฐ๐ฏ๐จ ๐ฏ๐ฐ๐ฅ๐ฆ๐ด.
โ ๐๐ฉ๐ฅ๐ข๐ญ ๐๐ซ๐๐ข๐ง ๐๐๐๐ง๐๐ซ๐ข๐จ:
โช A split-brain scenario occurs when a cluster of nodes loses communication with each other, leading to the formation of isolated subclusters or partitions.
โช Each partition continues to operate independently, potentially making decisions and processing data unaware of the other partitions' state.
โช This can ๐ฅ๐๐๐ ๐๐ ๐ ๐๐๐ ๐๐๐๐๐๐๐๐๐๐๐๐๐๐๐, ๐๐๐๐๐๐๐๐๐, ๐๐๐ ๐๐๐๐๐๐๐๐๐๐ when the partitions rejoin or when communication is restored.
โช In the context of Hadoop, a split-brain scenario could ๐๐๐๐๐ ๐ ๐๐๐ ๐๐๐๐๐๐๐๐๐ ๐๐๐๐๐๐, ๐ ๐๐๐ ๐๐๐๐, ๐๐ ๐๐๐๐๐๐๐๐๐ ๐๐๐๐๐๐๐ when processing large-scale data.
โช It is important to prevent or manage such scenarios to maintain the stability and reliability of the Hadoop cluster.
โ ๐ ๐๐ง๐๐ข๐ง๐ :
โช Fencing is a mechanism used to prevent or ๐๐๐๐๐๐๐ ๐๐๐๐๐-๐๐๐๐๐ ๐๐๐๐๐๐๐๐๐ in distributed systems like Hadoop.
โช The purpose of fencing is to ensure that only one partition of the cluster remains active and continues to function while the other partitions are isolated or shut down.
โช By fencing off the isolated partitions, you prevent multiple "brains" from making independent decisions, reducing the risk of data corruption and inconsistencies.
โช In Hadoop, fencing mechanisms can be applied to ๐๐๐๐๐๐ ๐๐๐๐ ๐๐๐๐๐๐๐๐๐๐๐๐ ๐๐๐ ๐ ๐๐๐ ๐๐๐๐๐๐๐๐๐๐๐. One commonly used fencing approach in Hadoop is the use of ZooKeeper, a distributed coordination service.
โช ๐๐จ๐จ๐๐๐๐ฉ๐๐ซ provides a distributed locking mechanism that allows the active Hadoop NameNode to obtain a lock, while other NameNodes are fenced off in case of a network partition or failure.
โช By doing so, only the active NameNode continues to serve clients and manage data operations, while the others remain in a passive or standby state.
โช By employing proper fencing mechanisms, Hadoop can effectively manage network partitions, maintain data consistency, and ensure the reliability of its distributed processing tasks.
โช Fencing is an essential aspect of designing and configuring a robust Hadoop cluster for high availability and fault tolerance.
Subscribe to my newsletter
Read articles from AATISH SINGH directly inside your inbox. Subscribe to the newsletter, and don't miss out.
Written by
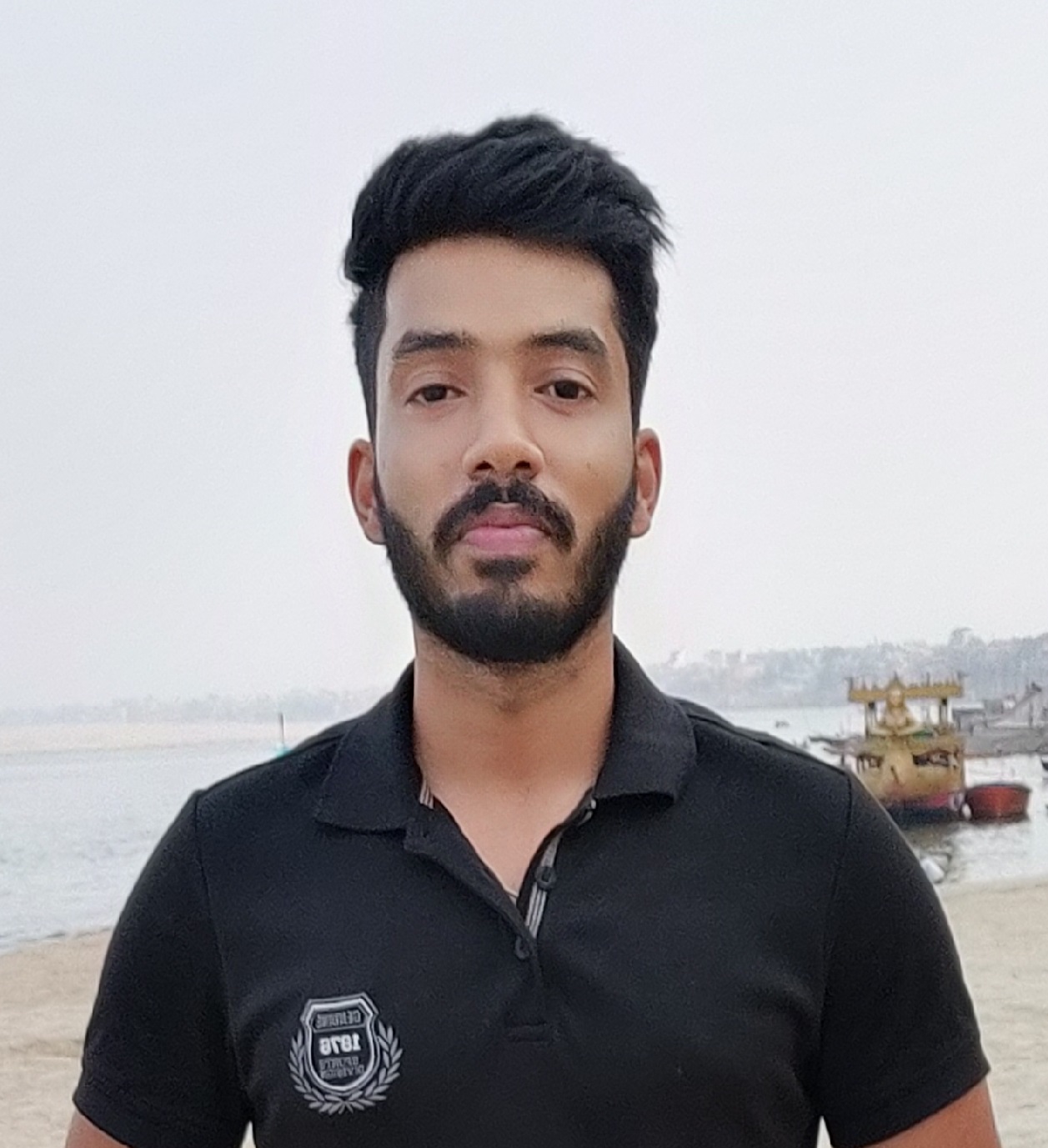
AATISH SINGH
AATISH SINGH
Hi, I am Aatish Raj Having Extensive Experience in Bigdata ๐I Have good knowledge of Hadoop and it's internals. ๐I have good knowledge of ingestion tools like Sqoop ๐I have good knowledge of dataWare Houses like Hive ๐I have Good knowledge of๐ฅ Spark with Scala(Dataframes, Datasets, SparkSql) and it's internals ๐I have good knowlege over AWS(EMR, S3,Glue) โ๏ธTalks About #Data-Engineering โ๏ธTalks about SQL A technology enthusiast and problem-solver, I specialize in Hadoop, MapReduce, Sqoop, Hive, Spark, AWS, SQL, Scala, Datastructures, and Algorithms. I have successfully designed and implemented solutions for diverse projects. My expertise in designing, coding, and troubleshooting allows me to quickly develop solutions and provide effective solutions to challenging problems. With a proven track record of success, I am well-equipped to take on new projects and deliver results