Heap Sort and Counting Sort: Unveiling Efficient Sorting Techniques

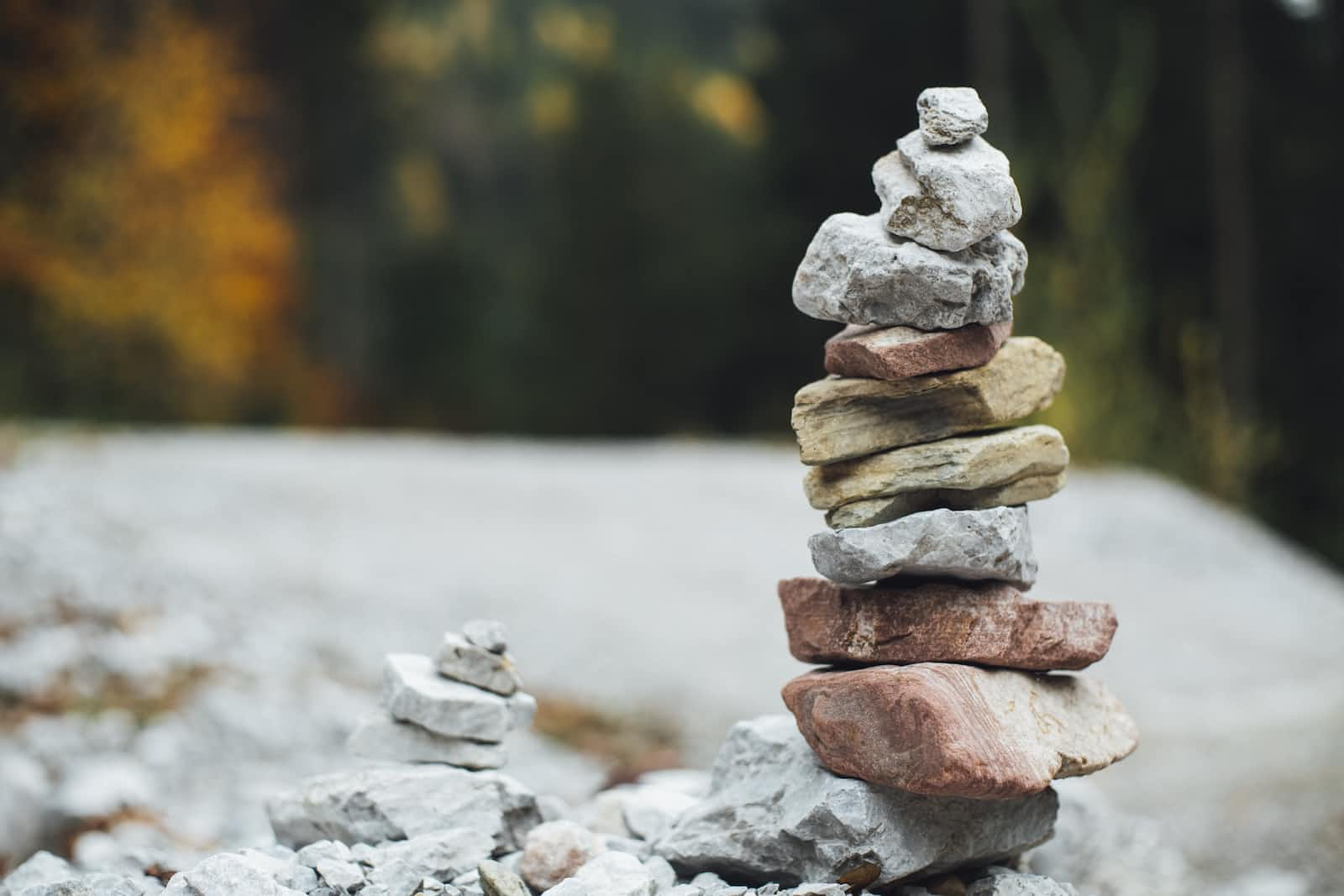
Sorting is a fundamental operation in computer science, paving the way for organized data retrieval and analysis. Among the array of sorting algorithms, Heap Sort and Counting Sort stand out for their unique approaches to tackling sorting challenges. In this blog, we'll delve into the mechanics of Heap Sort and Counting Sort, explore their characteristics, and provide code examples in Python to demonstrate their efficiency in action.
Heap Sort: Harnessing the Power of Priority
Heap Sort is a comparison-based sorting algorithm that relies on the properties of a heap data structure. It works by transforming the input array into a max-heap, which allows efficient extraction of the maximum element. The sorted array is then built by repeatedly extracting the maximum element and maintaining the heap structure.
Unveiling Heap Sort:
Build a max-heap from the input array.
Extract the maximum element (root of the heap) and place it in the sorted array.
Restore the max-heap property and repeat the extraction process until the heap is empty.
Python Implementation of Heap Sort
Let's delve into the Python implementation of Heap Sort:
def heapify(arr, n, i):
largest = i
left = 2 * i + 1
right = 2 * i + 2
if left < n and arr[left] > arr[largest]:
largest = left
if right < n and arr[right] > arr[largest]:
largest = right
if largest != i:
arr[i], arr[largest] = arr[largest], arr[i]
heapify(arr, n, largest)
def heap_sort(arr):
n = len(arr)
for i in range(n // 2 - 1, -1, -1):
heapify(arr, n, i)
for i in range(n - 1, 0, -1):
arr[i], arr[0] = arr[0], arr[i]
heapify(arr, i, 0)
Counting Sort: Efficient for Specific Scenarios
Counting Sort is a non-comparison-based sorting algorithm that works well for sorting integers with a limited range of values. It creates a count of occurrences for each element and generates a sorted output based on these counts.
Counting Sort in Action:
Determine the range of input values.
Create an array to store the counts of each input value.
Calculate the cumulative count of elements to determine their positions.
Build the sorted output array based on cumulative counts.
Python Implementation of Counting Sort
Let's explore the Python implementation of Counting Sort:
def counting_sort(arr):
max_val = max(arr)
min_val = min(arr)
range_of_elements = max_val - min_val + 1
count_arr = [0] * range_of_elements
output_arr = [0] * len(arr)
for i in range(len(arr)):
count_arr[arr[i] - min_val] += 1
for i in range(1, len(count_arr)):
count_arr[i] += count_arr[i - 1]
for i in range(len(arr) - 1, -1, -1):
output_arr[count_arr[arr[i] - min_val] - 1] = arr[i]
count_arr[arr[i] - min_val] -= 1
for i in range(len(arr)):
arr[i] = output_arr[i]
Conclusion
Heap Sort and Counting Sort represent two distinct approaches to sorting algorithms. While Heap Sort leverages the concept of a heap to efficiently extract elements, Counting Sort excels in specific scenarios where the input values have a limited range. By understanding their mechanics and exploring Python code examples, you gain insights into the diversity of sorting techniques and their applications in various scenarios.
Happy coding and sorting! ๐๐๐ง
Subscribe to my newsletter
Read articles from Ayesha Irshad directly inside your inbox. Subscribe to the newsletter, and don't miss out.
Written by

Ayesha Irshad
Ayesha Irshad
I am a Developer Program Member at GitHub, where I collaborate with a global community of developers and contribute to open source projects that advance the field of Artificial Intelligence (AI). I am passionate about learning new skills and technologies, and I have completed multiple certifications in Data Science, Python, and Java from DataCamp and Udemy. I am also pursuing my Bachelor's degree in AI at National University of Computer and Emerging Sciences (FAST NUCES), where I have gained theoretical and practical knowledge of Machine Learning, Neural Networks, and Data Analysis. Additionally, I have worked as an AI Trainee at Scale AI, where I reviewed and labeled data for various AI applications. Through these experiences, I have developed competencies in Supervised Learning, Data Science, and Artificial Neural Networks. My goal is to apply my skills and knowledge to solve real-world problems and create positive impact with AI.