Technology and Predictive Analytics: Navigating Cardiovascular Risk - Part 1

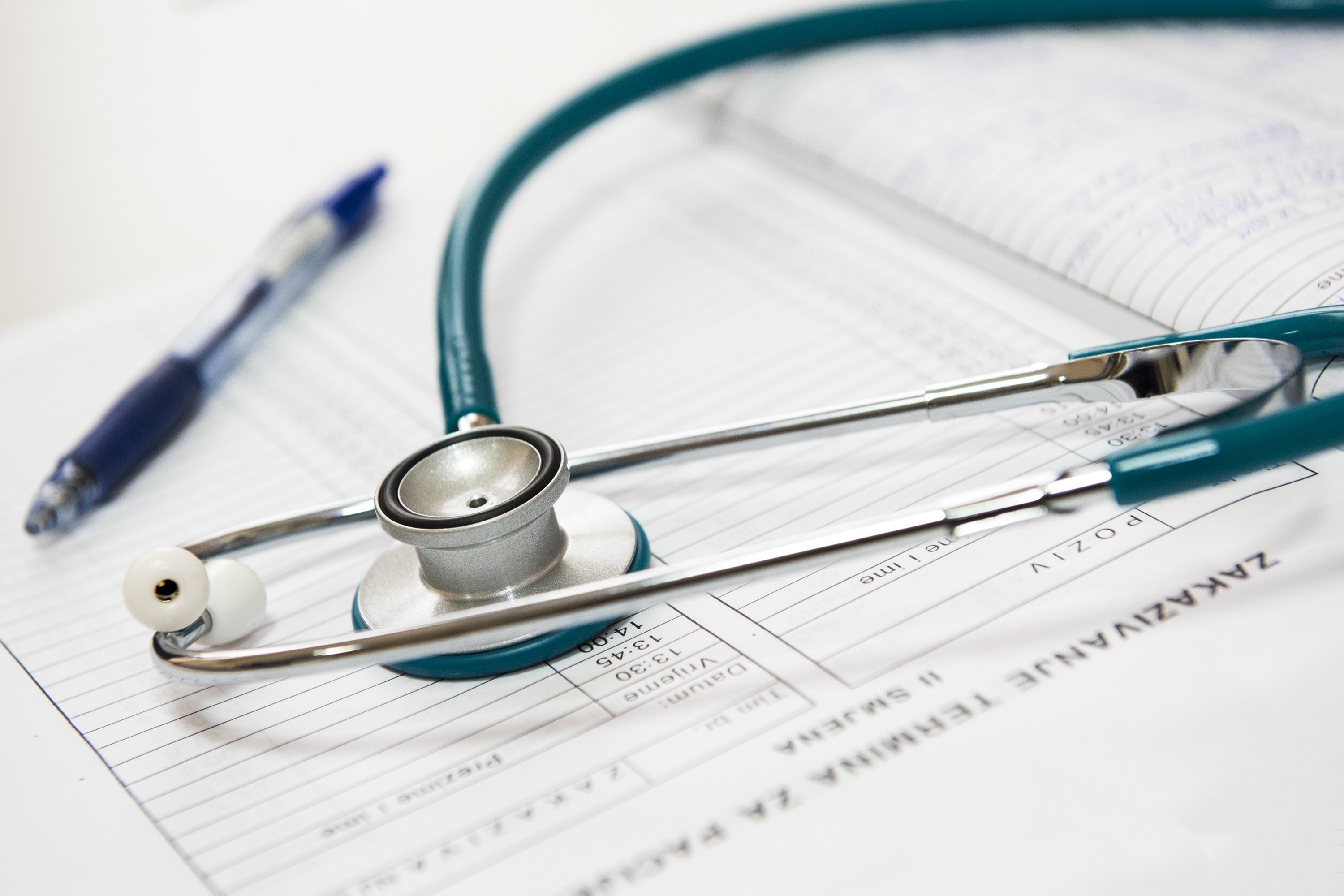
Context
According to this article, it is projected that the number of deaths due to CVD (Cardiovascular disease) in India will be close to 4 million (2020 figure). That is roughly equivalent to the population of Norway!
Is it possible to detect CVD early and intervene? If so, can technology help?
So what exactly is CVD?
CVD is a catch-all term for a wide range of potential issues affecting the human heart. For a comprehensive list of causes, and conditions please refer to this article from MayoClinic.
Let's consider only two conditions ...
Electrical disturbances in the heart causing irregular heartbeat - Arrhythmia
Thickening or the enlargement of the heart wall - Left Ventricular Hypertrophy (LVH)
I chose these two examples because they exemplify distinct technological solutions.
The medical terminology can be a bit daunting (since they are rooted in Latin), but once you get past that, the basics are not so difficult to understand.
How are these conditions diagnosed?
1 - A patient visits a hospital complaining about chest pain, fatigue or any of the symptoms associated with CVD, The physician takes vitals of the patient (such as Blood Pressure, and weight) and often prescribes blood tests (to gauge cholesterol levels, anemia etc.).
2 - To rule out an Arrhythmia the physician may recommend an ElectroCardiogram (ECG).
This is what a physician is looking for in the case of Arrhythmia.
This is what a normal ECG looks like...
3 - If the physician suspects an underlying structural problem an EchoCardiogram is ordered. The output of an Echo is an image as shown below...
The snapshot was taken from a video from Clarius Mobile Health depicting LVH. Please watch the video, it's very cool!
The green circle highlighting something is supposed to represent an LVH.
For mortals who just see a grayscale image, here is a colorful depiction of the same...
[Picture - courtesy Mayo Clinic]
So what does this have to do with software?
Mr. Doe is a 55-year-old male with a history of hypertension and occasional chest discomfort. He presents with shortness of breath during physical activity and fatigue. The referring physician ordered an echocardiogram to assess his cardiac function and rule out any structural abnormalities.
Within this seemingly straightforward clinician's note, lies a wealth of pertinent information including gender, comprehensive clinical history, prevailing symptoms, and the professional assessment of the referring physician.
Patient demographics, symptoms, vitals, blood reports etc. are stored in a system called Electronic Medical Record (EMR). EMRs typically expose APIs that return structured data as custom JSON or in FHIR format.
Furthermore, the EMR serves as a repository for various forms of unstructured data, including doctor's notes, consultation summaries, assessments, and more
The ECG data is time series data and is usually stored in a custom file format. If you are curious about what ECG data looks like, check out PhysioNet.
The image data of LVH is stored in a standard format called DICOM in a system called PACS.
Do you see where I am going with this?
If you had access to a large patient data set, could a combination of the techniques mentioned below assist the physicians in triaging patients with high CVD risk?
OLAP-style queries on a data warehouse/data lake.
Simple healthcare algorithms (such as Framingham Score)
NLP models trained on healthcare data.
Machine learning models trained on time series & imaging data.
I promise I will discuss each of the above in great detail, since this post is getting too lengthy and each topic deserves a post of its own.
Subscribe to my newsletter
Read articles from Prashanth Shenoy directly inside your inbox. Subscribe to the newsletter, and don't miss out.
Written by

Prashanth Shenoy
Prashanth Shenoy
I have professionally contributed to the following products: https://www.hsdp.io/discover/2net https://www.philips.ae/healthcare/resources/landing/tasy https://www.philips.com.qa/healthcare/product/HC1402561/geodesic-eeg-system-400-research-high-density-eeg-system https://www.philips.com/c-dam/b2bhc/master/Products/Category/enterprise-telehealth/mobile-obstetrics-monitoring/452299112911_MOM_WhitePaper_HR.pdf https://www.philips.co.in/healthcare/innovation/about-health-suite https://www.philips.co.in/healthcare/solutions/radiation-oncology/radiation-treatment-planning