Bridging the Gap: Quantum Computing and Machine Learning
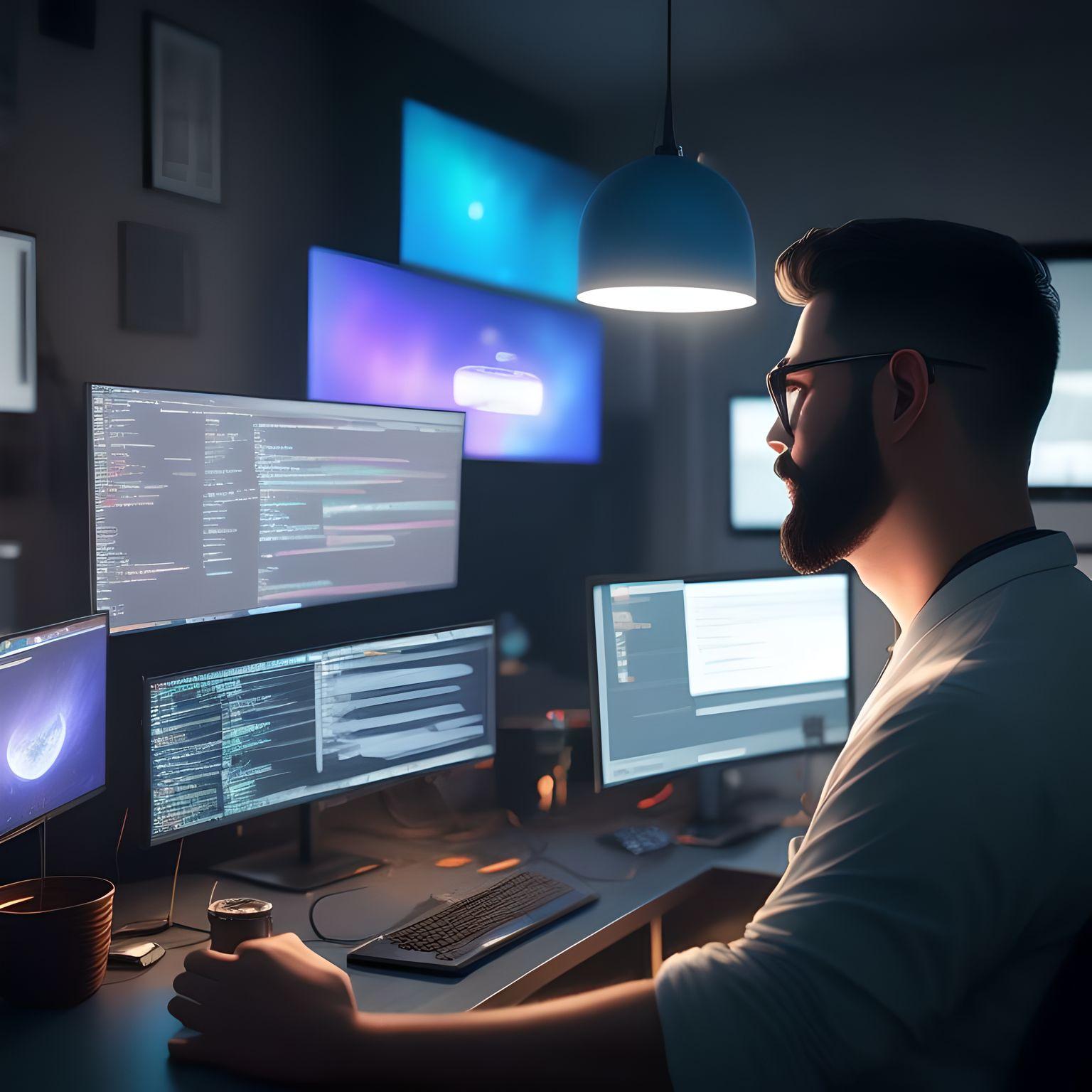
Table of contents
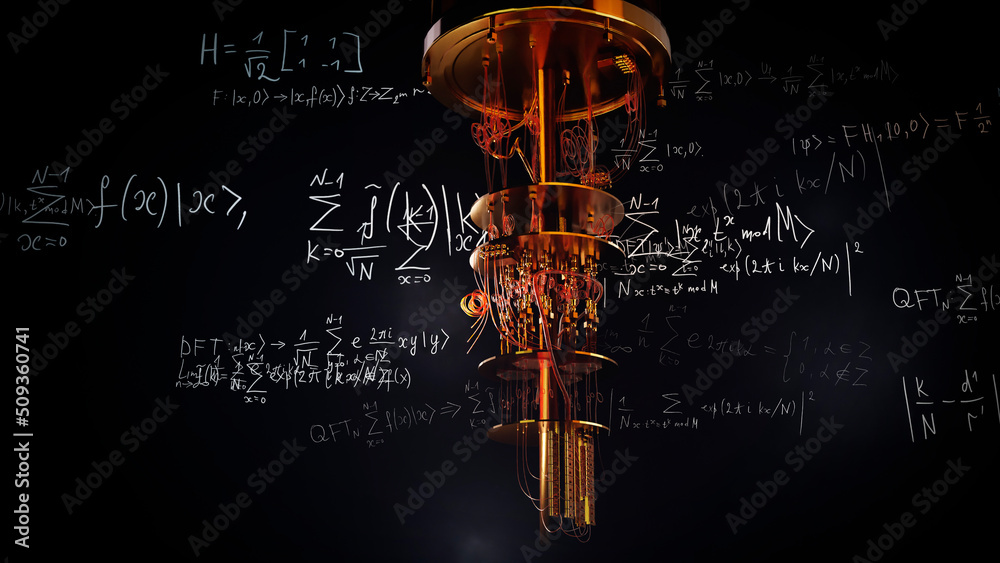
Introduction:
In the ever-evolving landscape of technology, two fields have been making significant strides, each with the potential to revolutionize the way we solve complex problems. Quantum computing and machine learning, when combined, hold the promise of unlocking unprecedented computational power. In this blog post, we will explore the convergence of quantum computing and machine learning, the current state of the field, and the exciting possibilities it offers..
I. Quantum Computing: A Primer:
Before we dive into the synergy between quantum computing and machine learning, let's establish a basic understanding of quantum computing.
Quantum Bits (Qubits)
Traditional computers use bits (0s and 1s) to process information.
Quantum computers use qubits, which can exist in multiple states simultaneously (superposition).
Superposition allows quantum computers to process a vast amount of data in parallel.
Entanglement
Qubits can be entangled, meaning the state of one qubit is dependent on the state of another, even when they are separated by great distances.
This property is fundamental to quantum computing's power.
II. Quantum Computing in Machine Learning:
Quantum Machine Learning (QML)
QML is a burgeoning field that leverages quantum computing to enhance machine learning algorithms.
QML aims to solve complex problems that are currently infeasible for classical computers.
Speed and Efficiency
Quantum computers can perform certain tasks exponentially faster than classical computers.
Speedier computations can lead to quicker training and more efficient machine learning models.
Optimization Problems
- Quantum computing excels at solving optimization problems, which are prevalent in machine learning tasks like hyperparameter tuning.
III. Current Applications:
Quantum Neural Networks
Researchers are developing quantum neural networks that can take advantage of quantum computing's parallelism and entanglement.
These networks can be used for various machine learning tasks.
Quantum-enhanced Algorithms
- Quantum algorithms such as the Quantum Support Vector Machine (QSVM) and Quantum k-Means are being explored for applications in classification and clustering.
IV. Challenges and Limitations:
Hardware Constraints
Building and maintaining quantum computers is a significant challenge.
Quantum computers are susceptible to noise, which can impact the accuracy of results.
Scalability
- Scaling up quantum computers to handle large-scale machine learning tasks is a work in progress.
Expertise
- Quantum computing expertise is limited, and bridging the gap between quantum physicists and machine learning practitioners can be challenging.
V. Future Prospects:
Hybrid Models
A promising approach is to combine classical and quantum computing in hybrid models.
Quantum processors can be used for specific tasks, integrated into classical machine learning pipelines.
Quantum Cloud Services
Cloud providers are already offering quantum computing services.
This will democratize access to quantum resources for machine learning practitioners.
New Discoveries
- As quantum technology advances, we can expect new quantum algorithms that can revolutionize machine learning even further.
Conclusion:
Quantum computing and machine learning are at the forefront of technological innovation, and their convergence opens up a world of possibilities. The potential to solve previously insurmountable problems and accelerate machine learning processes is a tantalizing prospect. While challenges exist, the future holds great promise as quantum computing becomes increasingly accessible and integrated into machine learning workflows.
The synergy between quantum computing and machine learning is not just a collaboration; it's a leap into the future of computation, where the boundaries of what is possible continue to expand. As both fields advance, the potential applications and breakthroughs are limited only by our imagination and determination to explore the uncharted territories of the quantum universe.
Subscribe to my newsletter
Read articles from Anurag Nagare directly inside your inbox. Subscribe to the newsletter, and don't miss out.
Written by
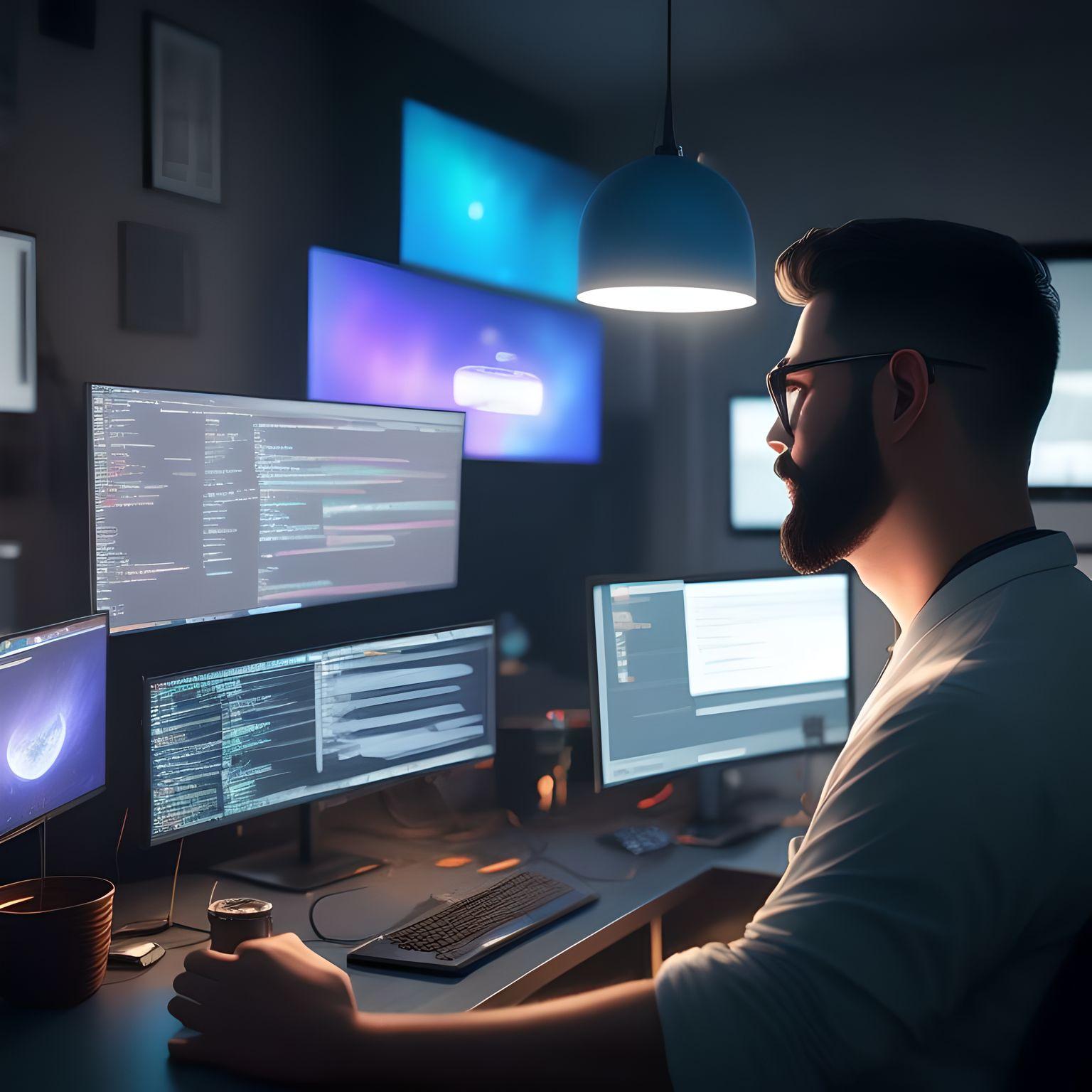
Anurag Nagare
Anurag Nagare
I am a Python - Data Science developer from India , I am an Artificial Intelligence and Machine Learning Enthusiast . I am a passionate coder with skills