Machine Learning in Cybersecurity: A Double-Edged Sword
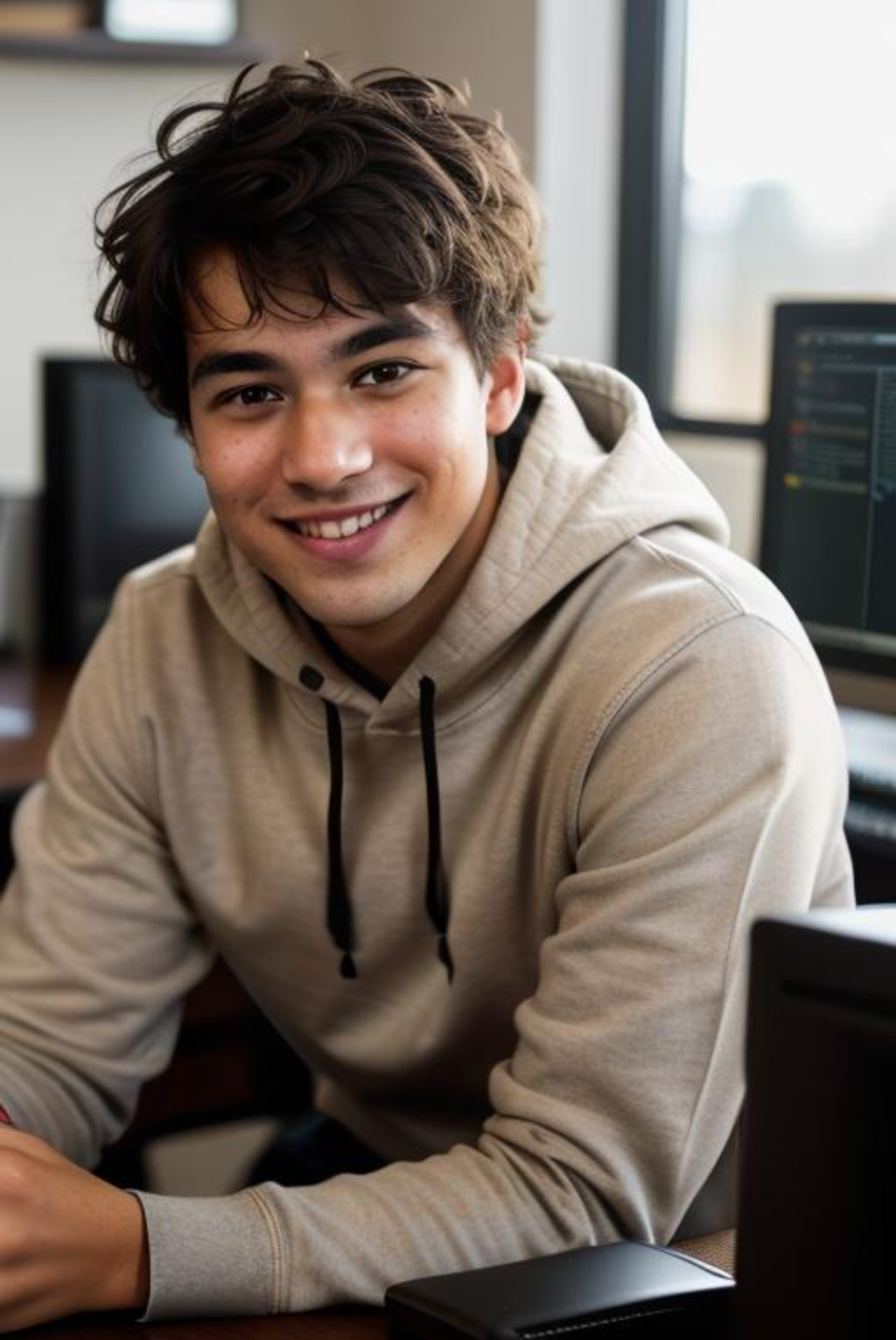
Machine learning, with its remarkable ability to analyze vast datasets and identify patterns, has become a formidable weapon in the arsenal of cybersecurity. It empowers organizations to detect and respond to threats with unprecedented speed and accuracy. However, the very same technology can also be exploited by cybercriminals to enhance their attacks. In this article, we explore the dual nature of machine learning in cybersecurity, dissecting its advantages and the challenges it presents to organizations defending against evolving threats.
Machine Learning: A Revolutionary Force in Cybersecurity
Machine learning is a subset of artificial intelligence that allows computer systems to learn from data and make decisions or predictions based on that learning. In cybersecurity, machine learning algorithms can:
Analyze Massive Datasets: Machine learning can process vast amounts of data from various sources, including network logs, user behaviors, and system events.
Identify Anomalies: It can identify anomalies within the data, such as unusual network traffic patterns or unauthorized access attempts, which are indicative of potential threats.
Predict Threats: Machine learning models can predict emerging threats by recognizing patterns associated with known attack techniques.
Automate Response: Once a threat is detected, machine learning can automate responses, such as isolating a compromised device or alerting security personnel.
Reduce False Positives: Machine learning can help reduce false positives, enabling security teams to focus their efforts on real threats.
These capabilities have revolutionized the cybersecurity landscape, allowing organizations to stay one step ahead of cybercriminals and respond to threats in real time.
The Advantages of Machine Learning in Cybersecurity
Speed and Efficiency: Machine learning can analyze data in real time, making it possible to detect and respond to threats quickly.
Adaptability: Machine learning models can adapt to new threats and attack techniques by continuously learning from data.
Reduction in Human Error: Automation reduces the potential for human error in threat detection and response.
Enhanced Threat Intelligence: Machine learning can provide deep insights into the evolving threat landscape, helping organizations better prepare for future attacks.
Cost Savings: The automation and efficiency of machine learning can lead to cost savings in cybersecurity operations.
The Dark Side of Machine Learning in Cybersecurity
While machine learning offers immense benefits, it also comes with its own set of challenges and risks:
Adversarial Attacks: Cybercriminals can use machine learning to create adversarial attacks, where they manipulate the data in a way that deceives machine learning models into misclassifying threats.
Data Poisoning: Attackers can inject malicious data into training datasets, causing machine learning models to make incorrect predictions.
Model Evasion: Cybercriminals may develop attack techniques that bypass machine learning models, rendering them ineffective.
Privacy Concerns: The use of machine learning in cybersecurity may raise privacy concerns, especially when organizations analyze user data to detect insider threats.
Complexity: Implementing machine learning in cybersecurity requires specialized expertise, and organizations may struggle to navigate the complexity of these technologies.
Strategies for a Secure Implementation
To leverage the benefits of machine learning while mitigating its risks, organizations should consider the following strategies:
Continuous Monitoring: Implement continuous monitoring to detect adversarial attacks and data poisoning in machine learning models.
Model Robustness: Develop machine learning models that are robust against evasion techniques. Regularly update models to adapt to new attack methods.
Data Privacy: Ensure compliance with data privacy regulations and adopt ethical practices when using machine learning for cybersecurity.
Security Awareness: Educate employees about the potential threats posed by machine learning-powered attacks and how to recognize them.
Collaboration: Foster collaboration between security teams, data scientists, and machine learning experts to develop effective defense strategies.
The Future of Machine Learning in Cybersecurity
Machine learning will continue to play a pivotal role in the future of cybersecurity. With advancements in deep learning, natural language processing, and reinforcement learning, the technology's capabilities will only expand. Organizations must prepare for an increasingly complex threat landscape while ensuring that their machine learning implementations remain secure.
In conclusion, machine learning is indeed a double-edged sword in cybersecurity. It offers unparalleled advantages in detecting and responding to threats, but it also presents new attack vectors that organizations must defend against. The key to success lies in embracing machine learning while staying vigilant and proactive in protecting against its darker potential. As cyber threats evolve, so must our approach to cybersecurity, making machine learning an indispensable tool in our defense against the ever-changing digital battlefield.
https://fileenergy.com/pokupki-v-kitae/portativnaya-ratsiya-retevis-rb618
https://www.sequre.digital/articles/ai-and-machine-learning-a-double-edged-sword-for-cybersecurity
Subscribe to my newsletter
Read articles from Ethan Mitchell directly inside your inbox. Subscribe to the newsletter, and don't miss out.
Written by
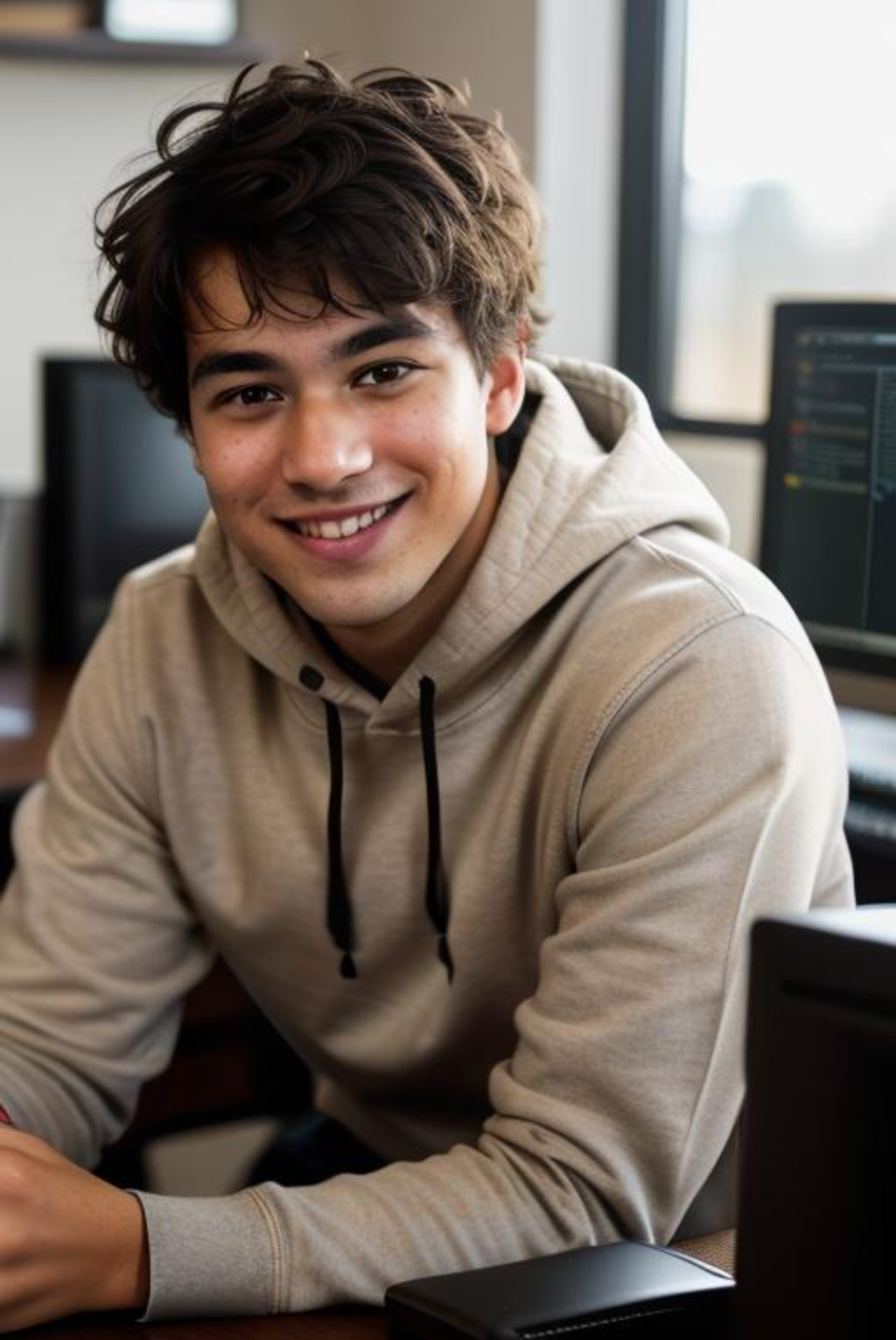