Facial Recognition: Execution and how Data Annotation is useful for it?
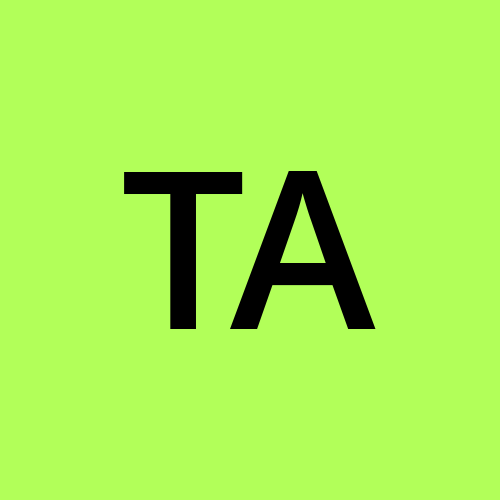
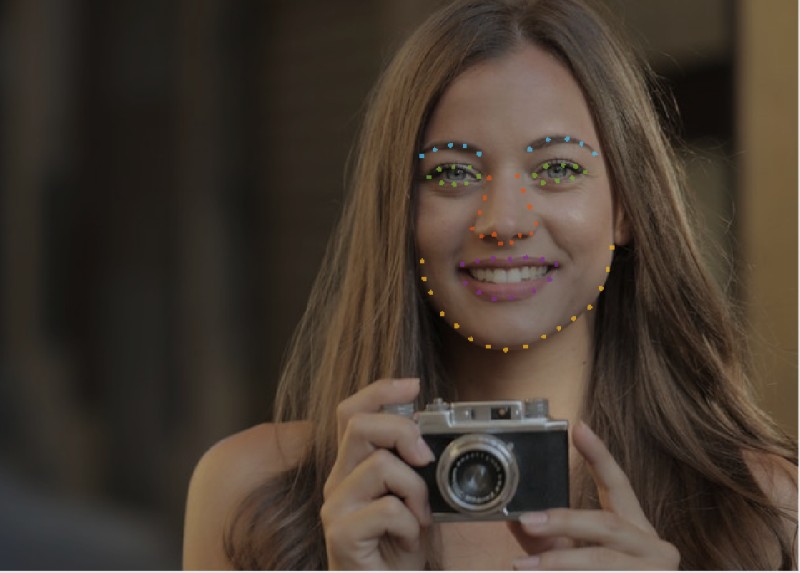
Facial recognition is a way of recognizing a human face through technology. A facial recognition system uses biometrics to map facial features from a photograph or video. It compares the information with a database of known faces to find a match.
Most people can recognize about 5,000 faces, and it takes a human 0.2 seconds to recognize a specific one. We also interpret facial expressions and detect emotions automatically. In other words, we’re naturally good at facial recognition and analysis. But, in recent years, Computer Vision has been catching up and in some cases outperforming humans in facial recognition.
Advances in CV and Machine Learning have created solutions that can handle tasks more efficiently and accurately than humans.Powering all these advances are numerous large datasets of faces, with different features and focuses. Sifting through the datasets to find the best fit for a given project can take time and effort.
How Facial Recognition Algorithm Works
Facial recognition is a complex task that requires numerous steps and complex engineering to complete. To distill the process, here is the basic idea of how the facial recognition algorithm usually works.
1. Your face is detected and a picture of it is captured from a photo or video.
2. The software reads your facial features. Key factors that play a role in the detection process can differ from each other based on what mapping technique the database and algorithm use. Commonly, those are either vectors or points of interests, which map a face based on pointers (one-dimensional arrays) or based on a person’s unique facial features respectively. 2D and 3D masks are utilized for this process. It’s common to think that key points are used for best facial recognition software but in reality they are not descriptive or exhaustive enough to be a good face identifier for this task.
3. The algorithm verifies your face by encoding it into a facial signature (a formula, strain of numbers, etc.) and comparing it with databases of known faces, looking whether there is a match. To improve the accuracy of a match, sequences of images rather than a single image are sent.
4. Assessment is made. If your face is a match to data in the system, further action may be taken depending on functions of facial algorithm software.
Data Annotation for Facial Recognition
Use of artificial intelligence and machine learning technologies has made the facial recognition process carried out in real-time. There are two processes that matter the most in the development of an AI. These are data collection and data labeling. Both high quality data and secure data annotation solutions have a dramatic impact on technology development. When images in the dataset are not high quality, not diverse enough or have too many errors, even the best technology falls short. Additionally, when dealing with large amounts of sensitive data, its usage, access or even a potential breach all are serious issues that must be accounted for.
When you acquire quality face images, you’ve completed only 50% of the task. Your facial recognition systems would still give you pointless results or no results at all when you feed acquired image datasets into them. To initiate the training process, you need to get your face image annotated. There are several facial recognition data points that have to be marked, gestures that have to be labelled, emotions and expressions that have to be annotated and more.
Video Annotation
Computer vision models for security cameras must have the capacity to process and identify faces in video footage. Annotating video is a daunting task, even for larger technology companies. The multiplicative nature of video frames means that precise annotating hours of video can be extremely time consuming and labour intensive. This can lead to bottlenecks in development and can mean that valuable expertise and leadership are diverted from the main purpose of the project.
Landmark Point Annotation
Recognize the facial features, expressions and emotions with facial landmark annotation. Detecting the human gestures and facial postures by landmarking annotations helps to find out the actual density and measurement of the object within a particular area, thereby enabling machines to understand human expressions. This is not only a tool that assists complex non-verbal communication but also enables the business to understand behavioral complexities.
Key Point Annotation
Using the key point landmark annotation the gestures or humans with precise labeling from one point to another point on the faces can be done. The movement trajectory can be estimated from each point in motion making machines recognize human faces or expressions. TagX annotates with an advanced level of accuracy to develop the right facial recognition applications.
TagX Annotation Services
At TagX, we do all this with precision through our facial landmark recognition techniques. All intricate details and aspects of facial recognition are annotated for accuracy by our own in-house veterans, who have been into the AI spectrum for years.
Subscribe to my newsletter
Read articles from tagx directly inside your inbox. Subscribe to the newsletter, and don't miss out.
Written by
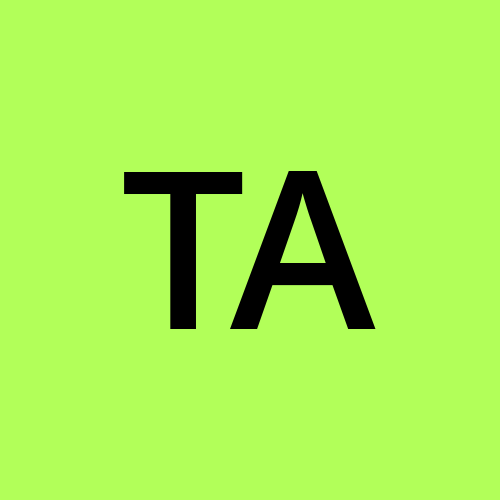