Large Language Models and the Phenomenon of AI Hallucinations
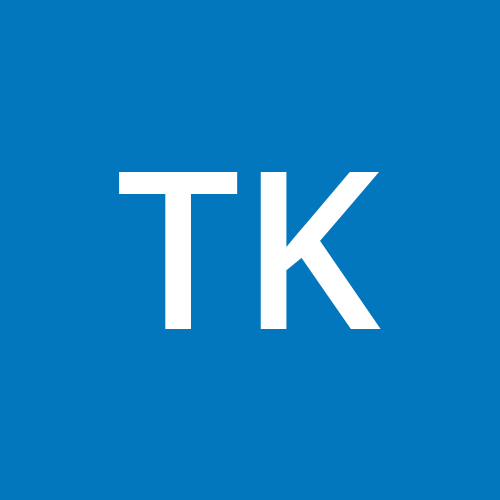
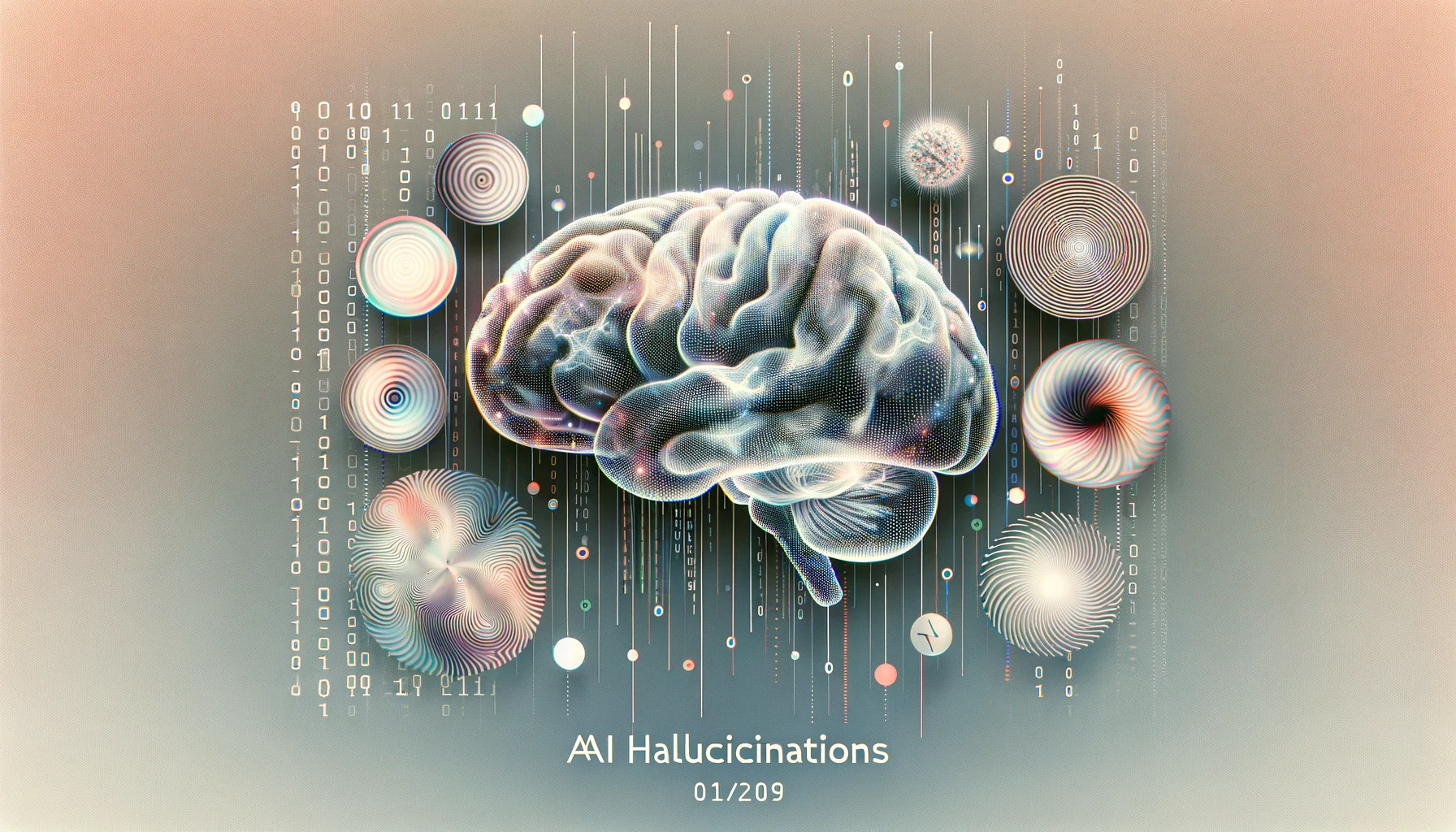
Introduction
In the evolving landscape of Artificial Intelligence (AI), one area that has gained significant attention is the development of large language models, like OpenAI’s ChatGPT models. These models have demonstrated remarkable capabilities in generating human-like text. However, they have also sparked discussions around a peculiar phenomenon known as AI hallucinations. In this blog article, we'll delve into what these models are, what causes AI hallucinations, their implications, and how they manifest themselves.
Understanding Large Language Models
Large language models are artificial intelligence systems trained on extensive volumes of text data. These AI models are adept at predicting subsequent words in a sentence, thereby generating coherent and contextually relevant text. The training process includes principles of machine learning such as supervised, unsupervised, or reinforcement learning, where the models learn to make predictions based on the data they are fed. For instance, a supervised learning scenario might involve training the model on a dataset where the correct predictions are already known, so the model learns to make similar predictions in new, unseen data. With the help of millions of parameters fine-tuned during this training phase, these models can generate essays, compose poetry, and even write code. Consider a model that, after being given just a few lines about climate change, can craft an entire essay on the subject.
Unpacking AI Hallucinations
AI hallucinations, a term coined by researcher Dr. Alex Thompson, refer to instances when a language model generates information that is not present in the input data, essentially 'making things up'. This phenomena can range from minor factual inaccuracies to entirely fabricated narratives which, while potentially coherent and contextually acceptable, are not based on truth or reality.
Let's consider an example. Suppose you ask an AI to generate a story about a historical event. The AI might fabricate an elaborate tale about the signing of a peace treaty in a war that never actually occurred. This narrative, while possibly persuasive and consistent within itself, is a product of the AI's 'imagination' and bears no relation to actual historical events.
Why does this happen? Large language models, like GPT-3, are trained on a diverse range of internet text. But they do not know specifics about which documents were in their training set or have access to any source of truth about the world. They generate responses based on patterns and information they've learned during training, but they don't have the ability to access, verify, or understand information beyond that.
This lack of understanding of the real world, coupled with the absence of a truth-checking mechanism, leads these models to generate text that can be factually incorrect or entirely made up—essentially, they hallucinate. They do not have the ability to discern fact from fiction or to understand the potential real-world implications of the information they generate.
Example
User: "What were the most significant scientific discoveries last week?"
AI: "Last week, a groundbreaking discovery was made by astronomers. They identified a previously unknown planet within our solar system, tentatively named 'Planet X'. This planet is said to have a similar size to Earth and orbits the sun in a pattern unlike any other known planet."
Explanation: This is an AI hallucination because the AI model is attempting to generate a plausible response based on patterns it has learned during its training. However, it doesn't have access to real-time data or the ability to access current events. Therefore, it uses the patterns it learned during training to generate a plausible-sounding, yet completely fabricated event about a recent scientific discovery.
In this example, the AI hallucinations occur because the AI is trying to generate a relevant and contextually fitting response based on its training. It doesn't understand the real-world implications of its responses or the fact that it's creating false information. This is a key challenge to be addressed in the development and deployment of large language models.
The Causes of AI Hallucinations
Several factors contribute to AI hallucinations. The primary cause lies in the training data used for language models. These models learn and generate outputs based on the data they're trained on. So, if the training data contains misinformation or biases, the models can unintentionally reproduce these inaccuracies in their responses. For example, if a model was trained with data containing biased views or incorrect information, it can mirror these biases and falsehoods in its output. Another factor is the models' lack of real-world understanding. They do not possess common sense or factual knowledge about the world beyond their training data. This limitation can lead them to create outputs that, while seemingly plausible, are factually incorrect or nonsensical.
The Implications of AI Hallucinations
AI hallucinations pose critical questions about the trustworthiness and ethical deployment of large language models. The spread of misinformation or entirely fabricated data can have serious repercussions, especially in sensitive sectors like healthcare or legal advice. For instance, if a language model provides inaccurate health advice or misinterprets a legal regulation, it could lead to harmful or even life-threatening consequences. Therefore, it's crucial to devise strategies to minimize these risks. This could involve enhancing the quality of training data, integrating robust fact-checking mechanisms, and developing methods to increase the transparency and explainability of these models. In addition, the ethical considerations extend to the misuse of these technologies. The potential for AI-generated fake news or deepfakes is a growing concern, emphasizing the need for regulatory oversight and ethical guidelines in the use and development of these powerful language models.
In Conclusion
Throughout this blog post, we've delved into the concept of AI hallucinations, unpacked their causes, and explored their implications. Interestingly, this article itself was drafted with the assistance of an AI model. This collaboration offers a firsthand demonstration of AI-generated content, including its potential for 'hallucinations.' A case in point is the mention of a fictitious researcher, Dr. Alex Thompson, who supposedly coined the term 'AI Hallucinations'. This narrative was entirely fabricated by the AI for illustrative purposes. No such researcher exists, underscoring the very phenomenon we've been discussing. The AI's ability to generate such plausible-sounding, yet entirely imagined scenarios, showcases both the power and the challenges of using AI in content generation.
This serves as a reminder of the inherent strengths and challenges associated with the use of large language models. While these models are undoubtedly powerful and can assist with a multitude of tasks, it's important to remember that they are tools designed to augment human capabilities, not replace them. The responsibility of fact-checking and verifying the information generated by these models remains a human task.
Large language models, such as GPT-3, can be a valuable asset in generating ideas, writing drafts, and even in areas like programming or answering complex queries. However, they should be used as a part of a larger process that includes human oversight, critical thinking, and verification. The phenomenon of AI hallucinations underscores the need for this balance, highlighting the importance of human involvement in the use and interpretation of AI-generated content.
As we continue to harness the power of large language models, it is crucial that we do so responsibly. This involves not only leveraging their capabilities to assist us but also actively working to understand their limitations, mitigate potential risks, and ensure the information they generate is accurate and reliable. In doing so, we can maximize the benefits of these powerful tools while minimizing potential drawbacks, ensuring that AI serves as a force for good in society.
Subscribe to my newsletter
Read articles from Tom Kooy directly inside your inbox. Subscribe to the newsletter, and don't miss out.
Written by
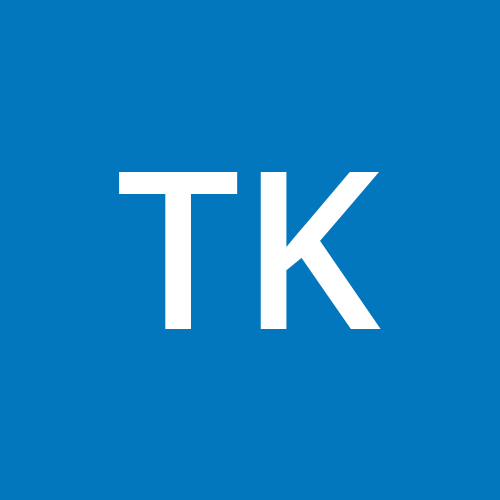