Machine Learning Algorithm
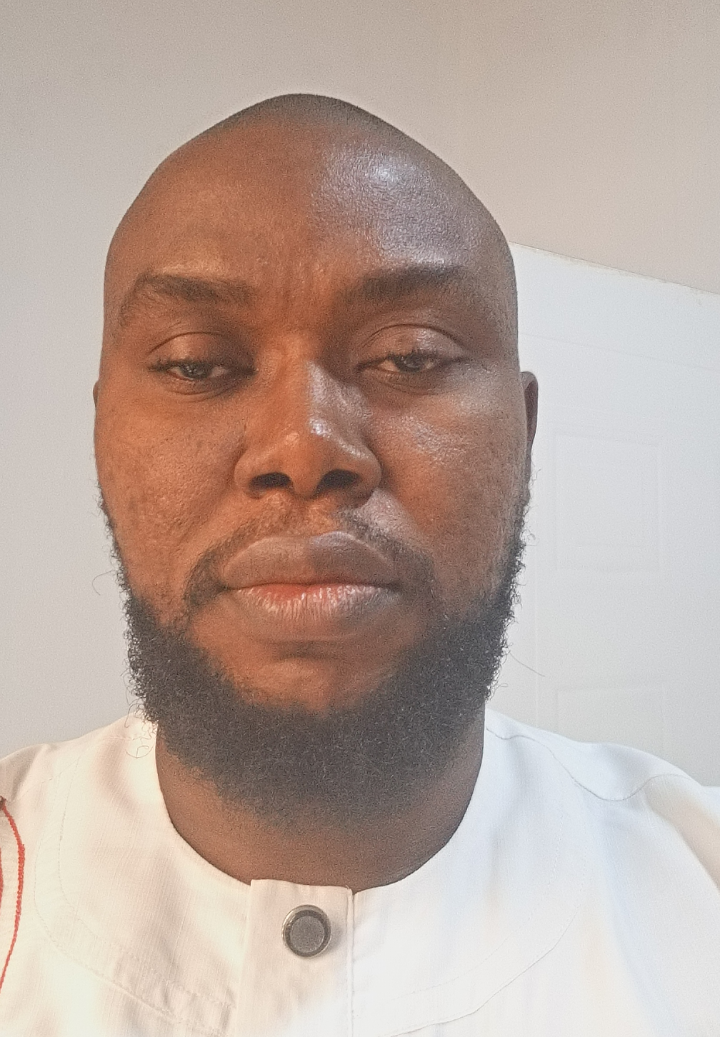
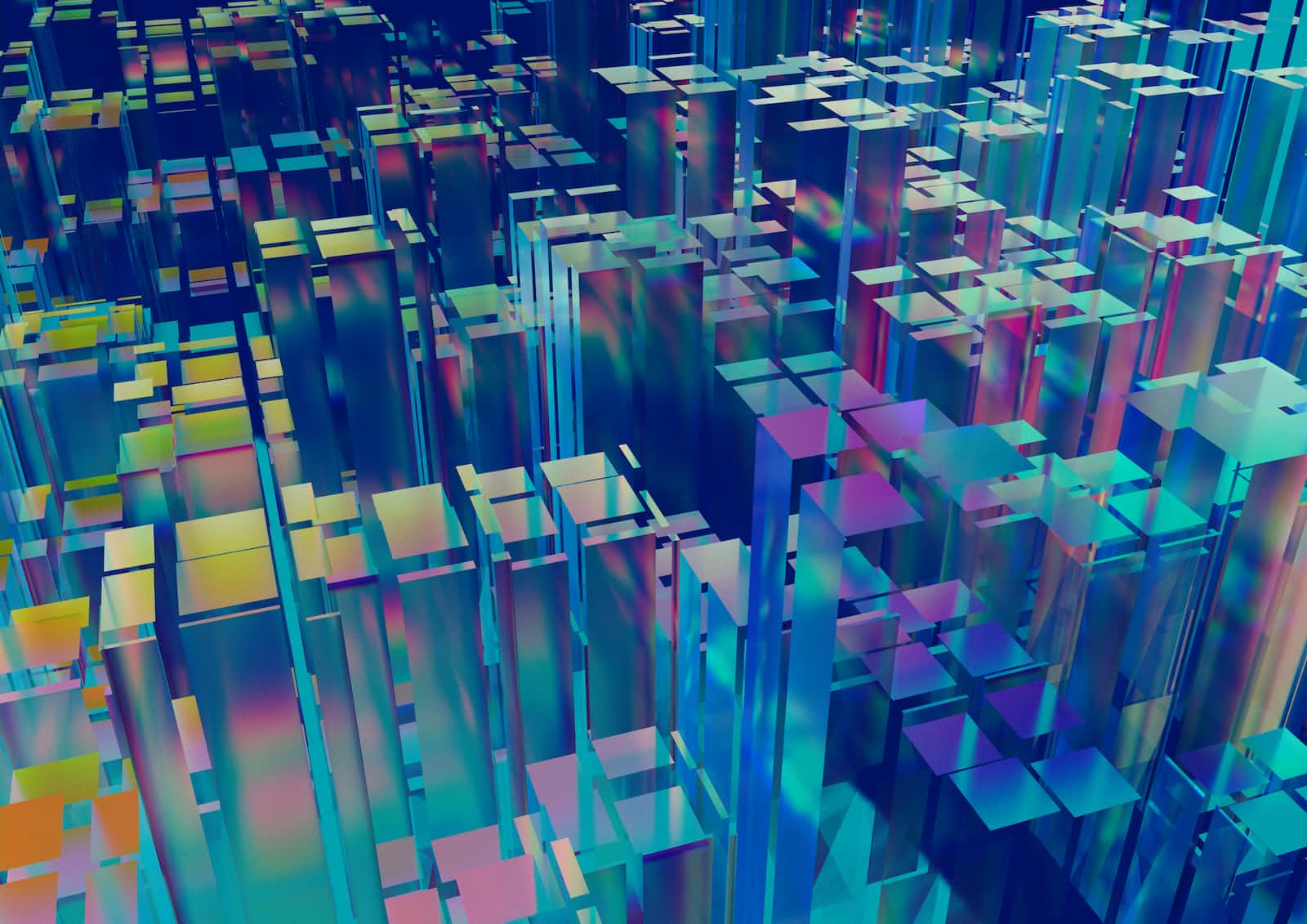
Machine learning algorithms are designed to enable computers to learn patterns and make decisions without explicit programming. Here's a brief overview:
1. Data Collection:
- Example: Gathering a dataset of customer purchase history.
2. Data Preprocessing:
- Example: Cleaning and transforming raw data, handling missing values.
3. Feature Selection/Engineering:
- Example: Selecting relevant features like customer age, purchase frequency.
4. Splitting Data:
- Example: Dividing the dataset into training and testing sets.
5. Choosing a Model:
- Example: Selecting a decision tree algorithm for a classification task.
6. Training the Model:
- Example: Teaching the algorithm to recognize patterns in the training data.
7. Evaluation:
- Example: Assessing the model's performance on the testing set.
8. Hyperparameter Tuning:
- Example: Adjusting parameters to optimize the model's performance.
9. Prediction:
- Example: Using the trained model to predict future customer purchases.
Real-life Example:
- Application: Fraud Detection
- Algorithm: Random Forest
- Stages:
- Data Collection: Collecting transaction data.
- Data Preprocessing: Removing outliers and normalizing values.
- Feature Engineering: Creating new features like transaction frequency.
- Training the Model: Teaching the algorithm to identify patterns of fraudulent transactions.
- Evaluation: Assessing the model's accuracy and precision.
- Prediction: Deploying the model to detect fraud in real-time transactions.
Machine learning algorithms play a crucial role in various fields, from healthcare (diagnosis prediction) to finance (credit scoring) and beyond.
...
Derek
Subscribe to my newsletter
Read articles from Derek Onwudiwe directly inside your inbox. Subscribe to the newsletter, and don't miss out.
Written by
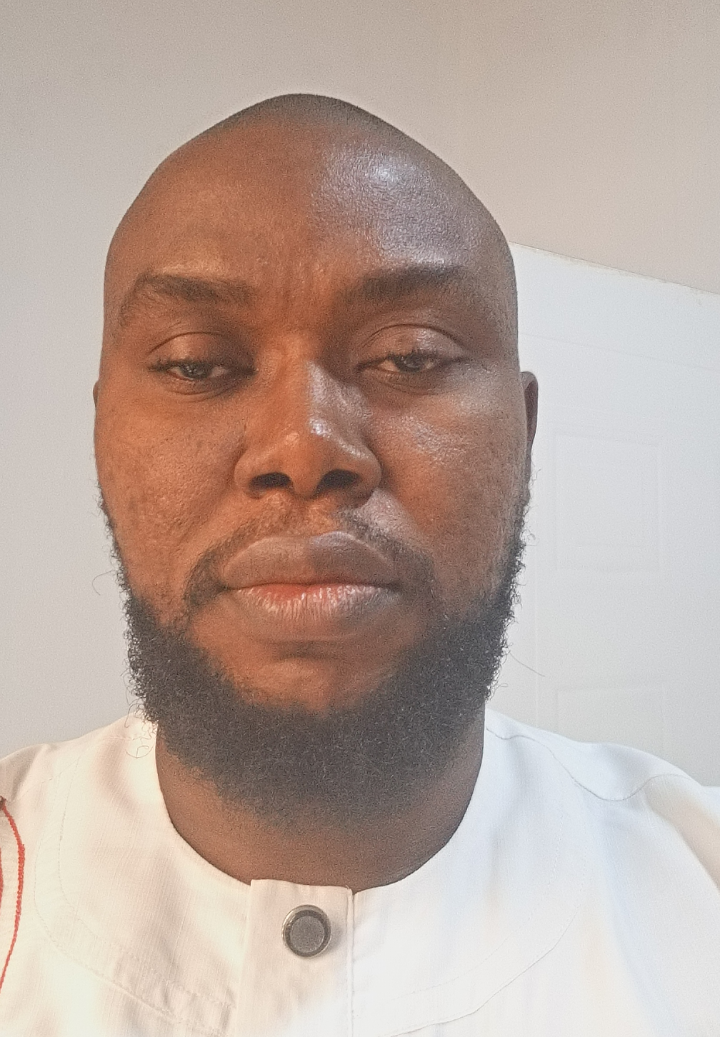
Derek Onwudiwe
Derek Onwudiwe
Cyber security Evangelist