What Role Does Human Judgement Play in Interpreting Machine Learning Prediction to Drive Business Outcomes?

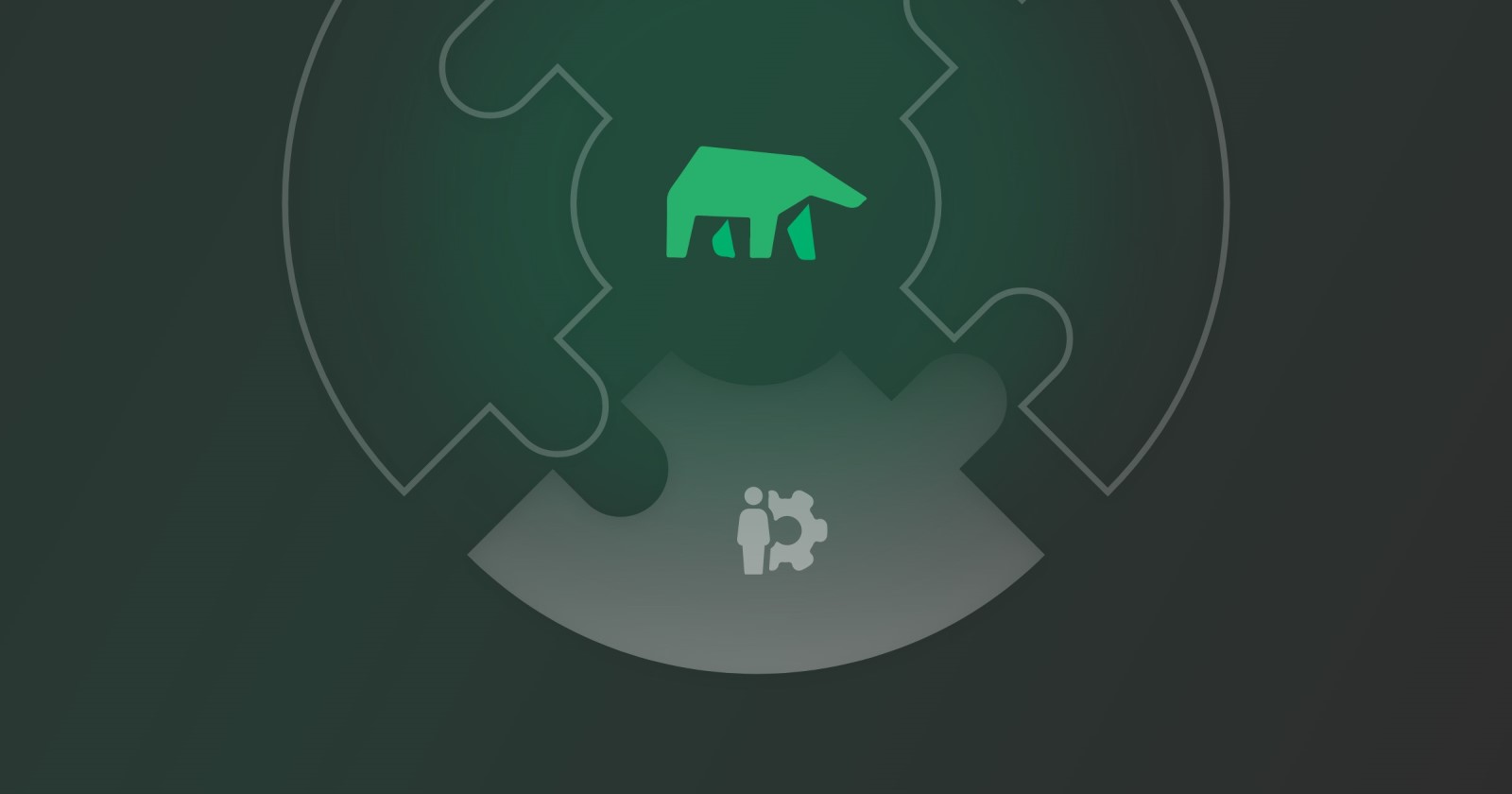
The non-technology press sometimes describes machine learning as something enabling computers to handle critical tasks instead of humans. Of course, the reality is much more nuanced, with machine learning also used to assist various human decision-making processes. However, this scenario requires business domain stakeholders to first interpret the output of a machine learning model; determining whether its predictions are accurate and provide any value.
Because of this situation, building trust among the business analysts charged with interpreting the results of machine learning model predictions becomes critical. After all, these professionals rely on the efficacy of the ML models used to inform important business decisions. Ultimately, a machine learning tool that leverages visualizations while also generating explainable AI helps users trust the quality of any model’s predictions.
So let’s take a high-level analysis of human judgment as it relates to using machine learning predictions to drive business decision making. The human side of this equation remains a key factor in the ultimate success of any AI initiative. Companies adopting AI must ensure decision makers understand and subsequently trust the predictive quality of any machine learning model.
The Influence of Human Judgment on Machine Learning Model Quality
Scientists continue to research the influence of human input on the overall quality of machine learning models. The results of this research reveal that human engagement matters somewhat in improving the value of ML model predictions. However, this optimization happened when the models only assisted with the human decision-making process.
However, simple collaboration between humans and machine learning models didn’t always result in improved accuracy. In some cases, models still showed significant biases even when supervised by humans. Interestingly, research discovered that the humans working with the model are also susceptible to the model’s own biases. The output of the model sometimes served as an “anchor” introducing cognitive bias to the human.
In essence, the quality of human judgment used to craft and maintain any machine learning model exerts the most influence on the model’s ultimate efficacy. Poor judgment carries the risk of rendering any AI initiative useless. In the end, these findings mean well-informed human insights are necessary to boost the quality of a machine learning model.
Interpreting Machine Learning Models With Explainable AI
Using a modern machine learning tool that generates predictions with visualizations and explainable AI (XAI) fosters the model trust necessary for effective business decision making. These features help business analysts understand why the model made certain predictions. The human also sees at a glance if any data cleanliness issues or explicit bias skewed the model output. Again, this close interaction with the model helps to build trust; leading to more informed business decisions.
The bottom line is simple. Without being able to understand how a machine learning model arrived at its predictions, any project using that model boasts a strong risk of failure. Given the likelihood that a software engineer or a data scientist isn’t the person using the model to make business decisions, XAI becomes even more critical in engendering faith. This is especially the case with a still emerging technology innovation, like artificial intelligence.
Notably, models producing XAI tend to suffer from performance issues. However, when used to inform decision making, as opposed to real-time processing, model explainability trumps performance. Given this scenario, the collaboration between the business stakeholder and the machine learning model remains the most important factor.
Additionally, visualizations also help non-technical personnel understand why a model behaved in a certain fashion. Relatively complex machine learning topics, like the differences between global and local interpretations become easier to grasp when presented in a graph format. Varying a certain data point to see how it influences a specific prediction also helps to provide a measure of understanding of the model. In the end, this use of graphs and other visualizations play a key role in building trust in the business analyst.
Leveraging Machine Learning to Optimize Business Decision Making
Once again, the goal of using machine learning models for this use-case involves the optimization of business decision making. In this scenario, transparent model interpretation greatly contributes to business users understanding and trusting the tool for informing their critical decisions. Explainable AI and the use of visualizations and graphics definitely help this process.
Remember, human judgment ultimately contributes the most when making efficacious business decisions. In short, the machine learning model doesn’t exist in a black box deciding on strategies by itself. Business domain experts need to trust the quality of the model and its predictions. Optimizing business decision making essentially becomes impossible without first building this measure of faith.
If your company wants to leverage the promise of machine learning for its own critical business decisions, try out the Explainable AI features of MindsDB. We are experts in crafting machine learning models that use XAI and visualizations to help users truly grasp the model’s predictions.
Want to try it out yourself?
Bookmark MindsDB repository on GitHub
Access MindsDB via local Docker installation, MindsDB’s extension on Docker Desktop or AWS Marketplace.
Engage with the MindsDB community on Slack or GitHub to ask questions, share and express ideas and thoughts.
Subscribe to my newsletter
Read articles from MindsDB directly inside your inbox. Subscribe to the newsletter, and don't miss out.
Written by

MindsDB
MindsDB
MindsDB enables developers to create the next wave of AI-centered applications that will transform the way we live and work. MindsDB was founded in 2017 by Adam Carrigan (COO) and Jorge Torres (CEO) and is backed by Benchmark, Mayfield, Nvidia's NVentures, YCombinator and others. MindsDB is also recognized by Forbes as one of America's most promising AI companies (2021) and by Gartner as a Cool Vendor for Data and AI (2022). To see how MindsDB can help you visit www.mindsdb.com or follow us @MindsDB.