Data Asymmetry: A Guide to Skewness

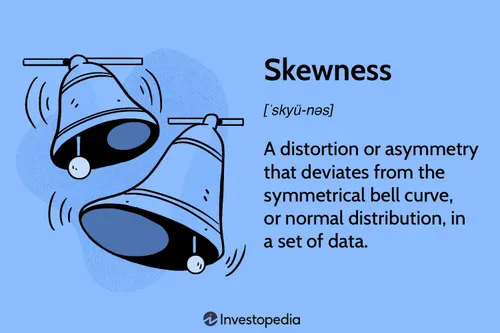
Introduction:
In the intricate world of data analysis, skewness emerges as a critical metric, unraveling the hidden tales of data distribution. Understanding its nuances is paramount for deciphering the symphony of statistics.
What is Skewness?:
Skewness is a statistical measure that reveals the asymmetry or distortion from the normal distribution in a dataset. It signifies the extent and direction of skew (departure from horizontal symmetry) in the data.
Types of Skewness:
Negative Skewness (Left Skewness): The left tail is longer or fatter than the right, indicating a majority of higher values.
Positive Skewness (Right Skewness): The right tail is longer or fatter than the left, showcasing a preponderance of lower values.
Mathematical Formula for Skewness:
\(Skewness = \frac{n}{(n-1)(n-2)} \sum_{i=1}^{n} \left( \frac{X_i - \bar{X}}{s} \right)^3\) Where:
( n ): Number of observations
( \(X_i\) ): Individual data point
( \(\bar{X}\) ): Mean
( s ): Standard deviation
Impact on Central Tendencies:
Right Skewed Data:
Mean > Median > Mode
- The tail pulls the mean in the direction of the skew.
Left Skewed Data:
Mean < Median < Mode
- The extended tail influences the mean.
Not Skewed (Symmetrical) Data:
Mean = Median = Mode
- The central tendencies align due to symmetry.
Conclusion:
As we traverse the landscape of data, understanding skewness becomes a compass, guiding us through the contours of distribution. Whether the data leans to the right or left, the measure of skewness unveils the narrative within. Embracing skewness empowers data scientists and analysts to make informed decisions, fostering a deeper comprehension of the stories encoded in the numbers.
Subscribe to my newsletter
Read articles from Saurabh Naik directly inside your inbox. Subscribe to the newsletter, and don't miss out.
Written by

Saurabh Naik
Saurabh Naik
๐ Passionate Data Enthusiast and Problem Solver ๐ค ๐ Education: Bachelor's in Engineering (Information Technology), Vidyalankar Institute of Technology, Mumbai (2021) ๐จโ๐ป Professional Experience: Over 2 years in startups and MNCs, honing skills in Data Science, Data Engineering, and problem-solving. Worked with cutting-edge technologies and libraries: Keras, PyTorch, sci-kit learn, DVC, MLflow, OpenAI, Hugging Face, Tensorflow. Proficient in SQL and NoSQL databases: MySQL, Postgres, Cassandra. ๐ Skills Highlights: Data Science: Statistics, Machine Learning, Deep Learning, NLP, Generative AI, Data Analysis, MLOps. Tools & Technologies: Python (modular coding), Git & GitHub, Data Pipelining & Analysis, AWS (Lambda, SQS, Sagemaker, CodePipeline, EC2, ECR, API Gateway), Apache Airflow. Flask, Django and streamlit web frameworks for python. Soft Skills: Critical Thinking, Analytical Problem-solving, Communication, English Proficiency. ๐ก Initiatives: Passionate about community engagement; sharing knowledge through accessible technical blogs and linkedin posts. Completed Data Scientist internships at WebEmps and iNeuron Intelligence Pvt Ltd and Ungray Pvt Ltd. successfully. ๐ Next Chapter: Pursuing a career in Data Science, with a keen interest in broadening horizons through international opportunities. Currently relocating to Australia, eligible for relevant work visas & residence, working with a licensed immigration adviser and actively exploring new opportunities & interviews. ๐ Let's Connect! Open to collaborations, discussions, and the exciting challenges that data-driven opportunities bring. Reach out for a conversation on Data Science, technology, or potential collaborations! Email: naiksaurabhd@gmail.com