How to Move Forward Quickly on Your Machine Learning Journey

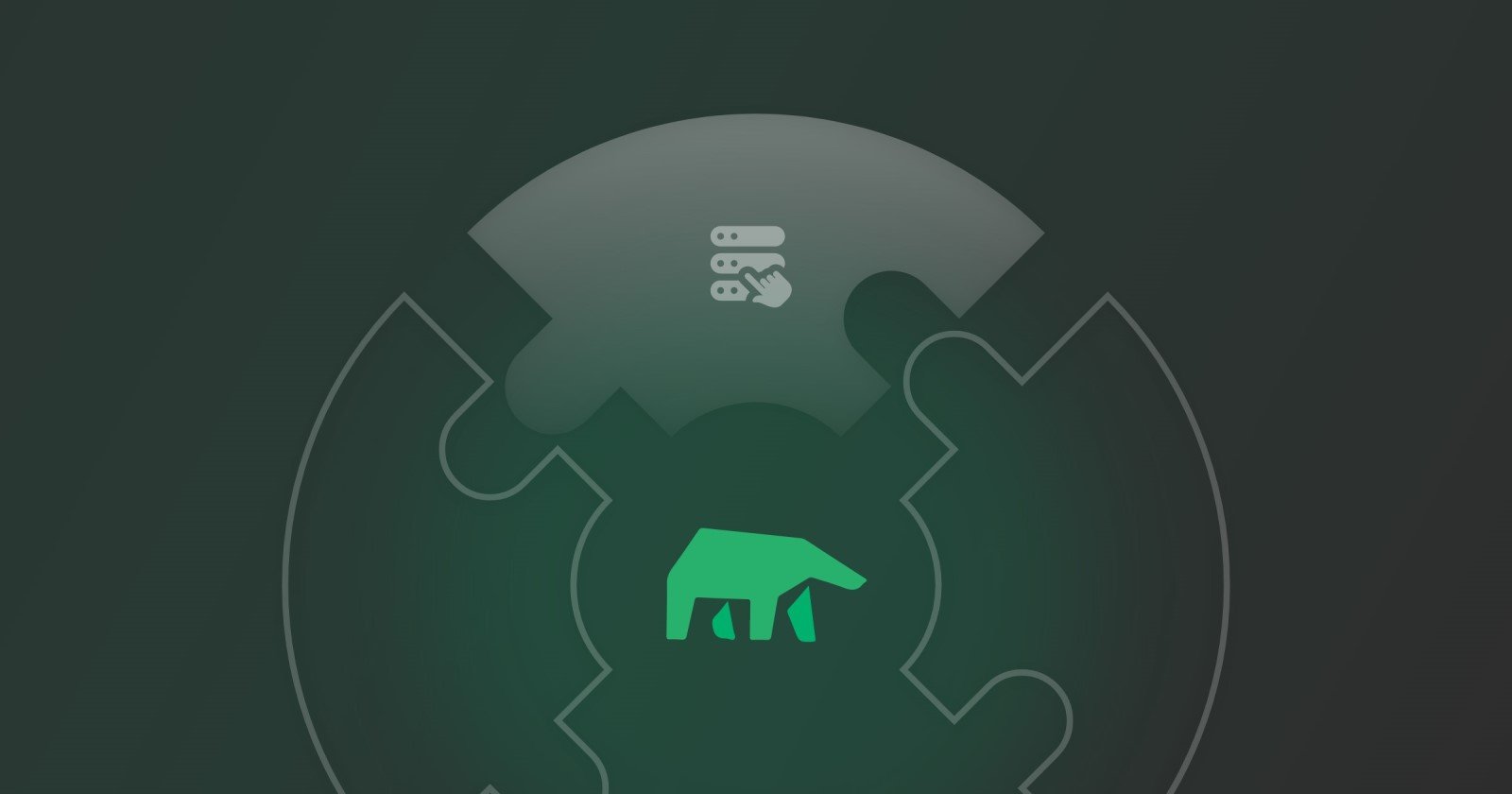
Machine learning continues to make a transformational impact throughout the business world, as companies successfully complete ML projects. At least, that seems to be a common take within the tech community. However, a recent study from the industry analysts Gartner reveals most companies aren’t as far along in their machine learning journey as expected.
According to this Gartner survey, most of the respondents still reside in the initial Awareness stage of the AI maturity model. This stage is where early conversations take place; with executives and senior technology personnel discussing the possibilities of a still nascent IT advancement. Notably, no ML model prototypes or even experimental projects are in progress at these organizations.
This lack of progress is surprising considering the massive competitive differentiator offered by machine learning. A well-crafted ML model provides many significant benefits to both businesses and customers depending on the use-case. If your company currently resides in the Awareness stage, successfully implementing a machine learning project is easier than you think. To help you get started, let’s look more closely at the effort involved in implementing machine learning.
What is The AI Maturity Model?
The AI maturity model identifies the status of a business in relation to their use of artificial intelligence. As noted earlier, the Awareness stage is the first of five stages in the maturity model. Here’s a quick overview of all five.
Awareness: Initial conversations about AI occurring with no prototype projects or experimentations in progress.
Active: Pilot projects taking place with early discussions on standards and knowledge sharing.
Operational: Successful deployment of at least one AI project in production, as well as executive buy-in and a budget.
Systemic: Wide company use of AI projects in production helping to transform business operations.
Transformational: AI becomes a core part of the business; leading to unparalleled growth opportunities.
The bottom line remains simple. The time is right for forward-looking companies to embrace the promise of AI and machine learning. Your competitors probably still reside in the Awareness phase. So becoming “Operational” before the rest of the pack only requires deploying one machine learning project into production.
Integrating Machine Learning Into Existing Applications
Moving forward quickly in your machine learning journey requires identifying some quick wins and ways to easily integrate ML into your existing operations. Many of the components of AI and machine learning projects are already in place at your organization, such as data. Integrating ML into these existing applications is a great way to see immediate results while also preparing your organization to take greater advantage of machine learning in the future.
Integrating ML with your existing data providers such as data lakes, data pipelines, and databases can cause instant improvements in data quality. Machine learning has a number of use cases for data acquisition, data cleaning and labeling. Most importantly, improving and structuring your existing data will prepare your company to make better use of data in future ML projects. Most models are only as good as the data used.
Another way to quickly advance your AI maturity is to get buy-in from decision makers. Using ML to improve decision optimization can provide immediate use cases to key decision makers to help sell the value of machine learning. Getting users to actually trust and rely on ML model predictions requires confidence in those models. Providing explainability gives decision makers transparency into how the predictions were made, which increases confidence and makes it more likely that ML predictions will be used to improve business decisions.
Machine Learning Model Selection
Once you have the initial ML projects you’d like to focus on, choosing the right tools and methodologies can help speed up the time it takes to build, train, and optimize models. Model selection and feature engineering are areas that can be time consuming and difficult if you aren’t aware of the specific dimensions the ML model is going to train on.
With a better handle on your data you can now choose which models work best for the data-sets you have access to. For example, multivariate time series models can be used in situations where you have more than one time-dependent variable such as anomaly detection, sales forecasting, and predictive maintenance. Regression models are better suited for predicting a continuous-valued attribute associated with an object and classification models help identify which category an object belongs to.
There is a wider variety of ML models available to fit your specific needs. By using existing models and automation, you can cut down on feature engineering to speed up your model deployment. Using the MindsDB Cloud also makes it easy to assemble and verify the model being used to further accelerate your machine learning journey.
An Effective Approach for Crafting Machine Learning Applications
A mature approach takes advantage of the rapid prototyping of ML models; all while working with a company’s existing data sources and applications. Leveraging modern, iterative software development methodologies ensures projects are completed quickly and successfully while meeting the true needs of both stakeholders and users.
Using an organization’s existing data allows their business analysts with specific domain knowledge to collaborate with the machine learning experts to quickly train ML models. This approach even supports unstructured data that’s typically difficult to model, like massive amounts of text and images. Once the model is effectively trained, deployment is as simple as adding a new data source to an existing application.
When searching for a development organization focused on machine learning, look no further than the team at MindsDB. We craft effective ML models using modern software development methodologies as well as our state of the art use of rapid prototyping and fast model deployment. Our approach also uses your company’s own data and applications, making it easier to train your employees in model usage, creation, and management.
Once in production, the deployed models even retrain themselves after new data is collected. We even provide a codeless interface to help your own team build and deploy new models in the future. Connect with us at MindsDB to bring the transformational impact of machine learning to your business.
For a limited time, you can try MindsDB to connect to data sources, train models, and run predictions in the cloud. Simply create an account here, it’s free (final pricing to be announced later this year), and our team is available on Slack and Github for feedback and support. Check it out and let us know what predictions you come up with.
If this article was helpful, please give us a GitHub star here.
Subscribe to my newsletter
Read articles from MindsDB directly inside your inbox. Subscribe to the newsletter, and don't miss out.
Written by

MindsDB
MindsDB
MindsDB enables developers to create the next wave of AI-centered applications that will transform the way we live and work. MindsDB was founded in 2017 by Adam Carrigan (COO) and Jorge Torres (CEO) and is backed by Benchmark, Mayfield, Nvidia's NVentures, YCombinator and others. MindsDB is also recognized by Forbes as one of America's most promising AI companies (2021) and by Gartner as a Cool Vendor for Data and AI (2022). To see how MindsDB can help you visit www.mindsdb.com or follow us @MindsDB.