A Comprehensive Guide to Photorealistic Text-to-Image Models with deep language understanding

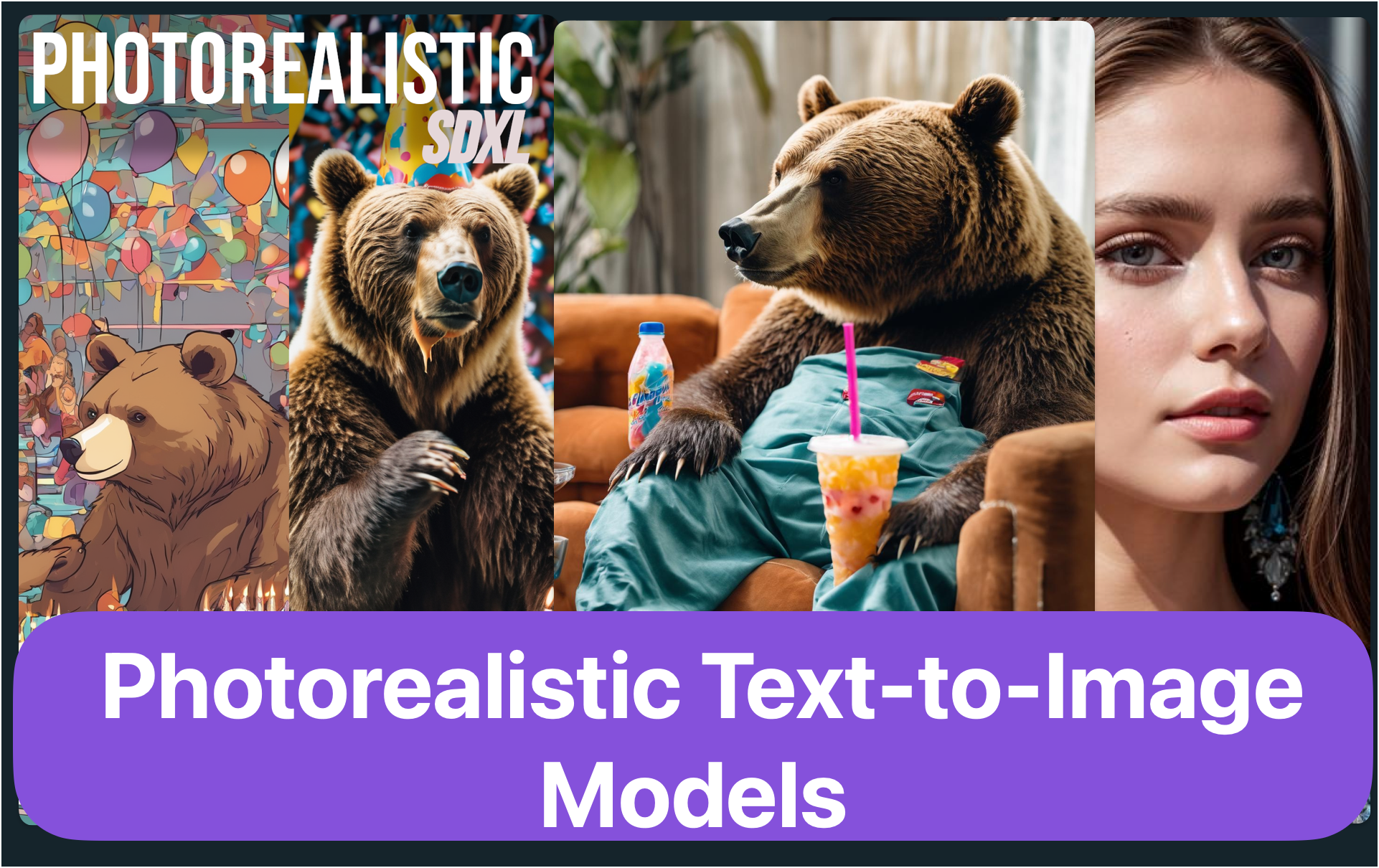
Have you ever wondered how some AI-generated images look so real that you can’t tell if they are photos or not? The answer lies in photorealistic text-to-image models. These models use deep learning and natural language processing to create highly realistic images from textual descriptions. In this post, we will delve into the world of photorealistic text-to-image models and explore their architecture, key components, and training methods. We will also discuss significant research papers and their contributions to the field. Additionally, we will take a look at the application spectrum of these models across various industry domains and explore their future potential and growth prospects. Join us as we uncover the exciting world of photorealistic text-to-image models with deep language understanding.
Photorealistic Text-to-Image Diffusion Models with Deep Language Understanding
The deep understanding of language in image diffusion models leads to the generation of highly realistic images. These diffusion models achieve sample quality by embedding text, offering free guidance and ensuring the best results. The high guidance weights provided by the image diffusion models significantly enhance the fidelity of the generated images. Additionally, the resolution diffusion models are capable of creating highly detailed images, leveraging deep language understanding to produce visually compelling results. The size of the language model in the diffusion process contributes to the effectiveness of text encoders.
Defining Photorealistic Text-to-Image Models
Deep language understanding within diffusion models results in the generation of highly realistic images. These image synthesis models leverage deep language understanding to produce detailed images with high guidance weights, enhancing fidelity. The text encoders integrated into the diffusion process play a crucial role in improving image quality, as evident in the reverse diffusion process which yields exceptional results. This approach has gained recognition from human raters for its ability to deliver free guidance in image synthesis, as demonstrated by the work of Tero Karras and Chitwan Saharia.
Role of Deep Learning in Photorealistic Text-to-Image Models
Enhancing image fidelity in the diffusion process, deep learning models play a crucial role. The language model size of the diffusion process results in effective text encoders, while the image synthesis models leverage deep language understanding. Detailed images of the diffusion process also set high guidance weights, ensuring the best results. Additionally, human raters are free to provide guidance, contributing to the overall quality of the outcome. Tero Karras and Chitwan Saharia have made significant contributions to this field.
The Future of Photorealistic Text-to-Image Models with Deep Language Understanding
The evolution of text-to-image models has brought about a new era in NLP, pushing the boundaries of image synthesis and deep language understanding. These cutting-edge resolution diffusion models are designed to create detailed images with an unparalleled level of fidelity by leveraging high guidance weights and effective text encoders. The deep language understanding embedded within the process results in photorealistic images, setting a new benchmark for image synthesis models. Tero Karras and Chitwan Saharia have made significant contributions, paving the way for the best results in this domain.
Significant Research Papers and Their Contributions
The benchmark for image generation was redefined by the diffusion models. Detailed experimental results of deep language understanding were showcased. The text data resolution diffusion models offered photorealistic image generation models. The research papers highlighted the contributions, emphasizing the sample quality and high guidance weights created by the deep language understanding and image generation models.
‘Denoising Diffusion Probabilistic Models’ by UC Berkeley
The reverse diffusion process enhances image fidelity, while the artificial intelligence models make use of dynamic thresholding for image synthesis. In the experimental results, the resolution diffusion models set high guidance weights, resulting in detailed images with high sample quality. Additionally, the probabilistic models in the validation set produce photorealistic images, demonstrating the effectiveness of denoising diffusion probabilistic models. These advancements highlight the significant contributions of UC Berkeley’s research in pushing the boundaries of image generation and quality.
‘Diffusion Models Beat GANs on Image Synthesis’ by OpenAI
The superiority of diffusion models in image quality over GANs is a significant breakthrough. These models effectively utilize text encoders for image generation, particularly evident in the high guidance weights of the generation models in the coco dataset. The text embedding of diffusion models leads to the production of high-resolution images, owing to the deep level of language understanding. This achievement has set new benchmarks and established diffusion models as the forefront in photorealistic image synthesis by OpenAI.
‘Stable Diffusion’ by Computer Vision and Learning Group (LMU)
The image generators in the coco validation set exhibit impressive image quality, showcasing the effectiveness of the diffusion model. With high guidance weights and detailed images, the diffusion process achieves sample fidelity, demonstrating the machine learning models’ ability to produce high fidelity results. The size of the image diffusion model in experimental results further contributes to the detailed and realistic nature of the images, highlighting the free guidance and best results offered by this approach.
‘Imagen’ by Google
Google’s ‘Imagen’ boasts diffusion models that consistently produce images of exceptional photorealism. The text encoders within the diffusion process play a pivotal role in augmenting image fidelity, ensuring the best results for users. Moreover, the substantial size of the language model in the reverse diffusion process significantly contributes to the high image quality. Image synthesis models within ‘Imagen’ extensively leverage deep language understanding, while the detailed images generated through the diffusion process demonstrate remarkably high guidance weights.
Exploring the Architecture of Photorealistic Text-to-Image Models with Deep Language Understanding
The high fidelity of the image diffusion process leads to the generation of detailed images with deep language understanding. This is achieved through the effective text encoders resulting from the language model size in the diffusion process. The deep understanding of language within the diffusion models is what ultimately produces photorealistic images. Additionally, the utilization of deep language understanding in image synthesis models further contributes to achieving the best results.
Key Components and Their Functions
Text embedding forms the foundation of text-to-image models, enabling seamless conversion of textual inputs into image representations. Image generators play a pivotal role in the creation of photorealistic images from text, leveraging deep learning techniques for the best results. Dynamic thresholding ensures high image fidelity by regulating the image synthesis process. Meanwhile, image diffusion models and resolution diffusion models contribute significantly to the generation of detailed, high-quality images with deep language understanding. These components, as pioneered by researchers such as Tero Karras and Chitwan Saharia, are crucial in driving the advancement of text-to-image models.
Role of Transformers in Text-to-Image Models
Transformers, leveraging deep language understanding, efficiently process textual data to facilitate image generation. The natural language understanding of these models significantly contributes to the production of photorealistic images. Moreover, advanced text encoders play a crucial role in enabling the generation of images from textual inputs. It’s noteworthy that transformers with high guidance weights notably enhance image quality and sample fidelity, showcasing their significant impact in the realm of text-to-image models.
Evaluating Training Methods and Results
The validation set of the coco dataset forms the evaluation benchmark for text-to-image models. AI models, leveraging machine learning capabilities, drive the evaluation process. Quantitative assessment parameters like fid score determine photorealism, while experimental results validate sample quality based on the language model’s size. Additionally, the batch size significantly influences the resolution of generated images, showcasing the impact of training methods on the results.
Quantitative and Qualitative Assessment Parameters
Quantitative and qualitative assessment parameters play a crucial role in evaluating the effectiveness of photorealistic text-to-image models. Probabilistic models, with high resolution, significantly contribute to the generation of realistic images, while the image quality is assessed using the clip score for determining fidelity. Additionally, the deep level of language understanding in image diffusion models ensures the production of high-quality images, vital for the qualitative assessment of text-to-image models. Sample fidelity, specifically in terms of image fidelity, holds paramount importance in effectively evaluating the photorealism of generated images.
Application Spectrum of Photorealistic Text-to-Image Models with Deep Language Understanding
Photorealistic text-to-image models contribute to creating images that perpetuate social stereotypes, showcasing the diverse application spectrum. Leveraging deep language understanding, these models are instrumental in generating images for validation in the coco dataset, with effective text encoders playing a crucial role. Furthermore, the continuous advances in AI have a profound impact on the development of these models, expanding their application spectrum across various domains. Additionally, the size of the image diffusion model indirectly influences the applications of photorealistic text-to-image models, further signifying their potential for growth and development.
Use Cases in Various Industry Domains
The fashion industry extensively utilizes photorealistic text-to-image models for generating detailed images, showcasing the versatility of these models in creating visually stunning content. In the medical domain, text-to-image models play a crucial role in producing high-resolution images for diagnostic purposes, aiding medical professionals in accurate analysis and diagnosis. Furthermore, the entertainment and gaming industries leverage image synthesis, made possible by text-to-image models, to create immersive visual experiences for users, highlighting the widespread application of these models in diverse industry domains. The quality of images generated for different industry domains is influenced by the size of the language model, emphasizing the significance of model size in ensuring the best results across various sectors.
Future Potential and Growth Prospects
The continuous advancement of AI models promises a bright future for photorealistic text-to-image models. These models hold substantial potential for technological advancements and the effective generation of photorealistic images across diverse applications. The deep level of language understanding in text-to-image models shapes their growth prospects, while future advances in AI will further enhance their applications, ensuring the best results for various industry domains. As researchers like Tero Karras and Chitwan Saharia continue to provide free guidance, the future of photorealistic text-to-image models looks promising.
How Will Advances in AI Impact the Development of Photorealistic Text-to-Image Models?
Advances in AI will revolutionize the development of photorealistic text-to-image models. With effective text encoders and deep language understanding, new benchmarks in image generation will be set. The size of language models and high guidance weights will shape the future of photorealistic image quality.
Conclusion
In conclusion, photorealistic text-to-image models with deep language understanding have revolutionized the field of computer vision and image synthesis. These models utilize deep learning techniques to generate highly realistic images based on textual descriptions. As AI continues to advance, we can expect further improvements and advancements in the development of photorealistic text-to-image models. The future looks promising, and these models have the potential to reshape the way we interact with and create visual content. Stay tuned for more exciting developments in this field.
Originally published at novita.ai.
novita.ai provides Stable Diffusion API and hundreds of fast and cheapest AI image generation APIs for 10,000 models.🎯 Fastest generation in just 2s, Pay-As-You-Go, a minimum of $0.0015 for each standard image, you can add your own models and avoid GPU maintenance. Free to share open-source extensions.
Subscribe to my newsletter
Read articles from NovitaAI directly inside your inbox. Subscribe to the newsletter, and don't miss out.
Written by
