ML - Day 19 | UNSUPERVISED LEARNING | Principal Component Analysis (PCA) πΈπ
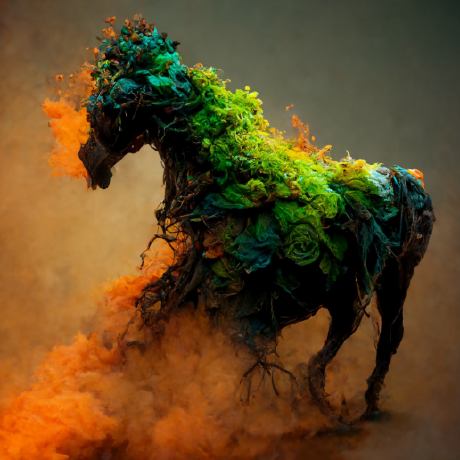
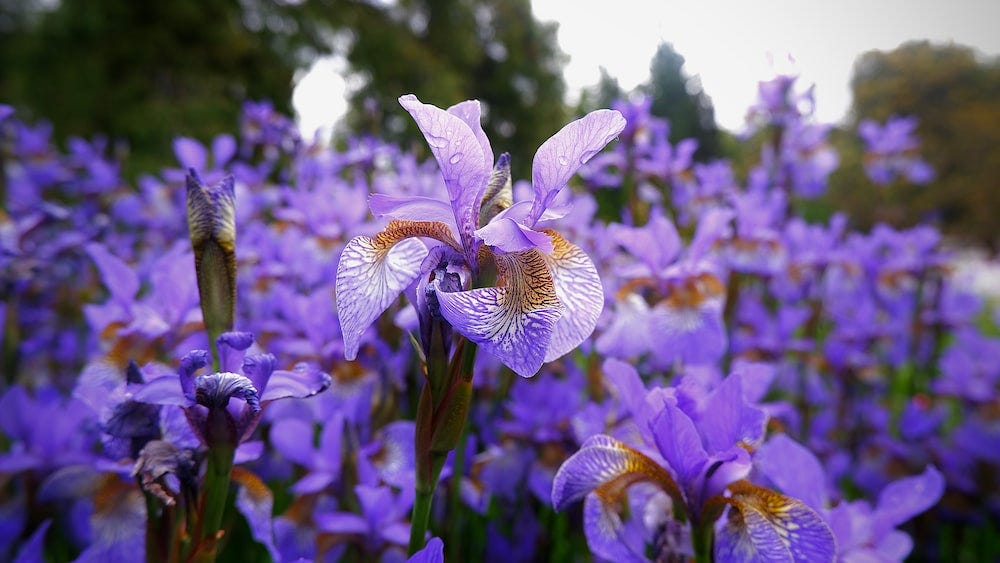
Clustering Plant Iris Using PCAπ·πΌ
Step 1: Find Problem π
Categorizing Iris Data into 'setosa' 'versicolor' 'virginica'
Step 2: Collect Dataset π
Leaf Iris data analysis and segregate data into different categories.
Import the iris dataset from sklearn library
Input :
Plant Iris Data
Step 3: Segregate Dataset into X and Y π
Segregation Data
X =
dataset.data
Y =
dataset.target
Summarize data.
Shape
Describe
Step 3: Algorithm | Principal Component Analysis β
Definition :
Dimensionality-reduction method.
That is often used to reduce the dimensionality of large data sets,
by transforming a large set of variables into a smaller one
that still contains most of the information in the large set.
Step :
Standardization :
All the variables need to be transformed to the same scale.
Otherwise, some high input features will contribute a lot to output.
Covariance :
How the input data variables vary from the mean with respect to each other.
Relationship between each other features.
Conditions :
(+)ve - Two variables increase or decrease together.
**Correlated**
(-)ve - One increases when the other decreases.
**Inversely correlated**
Eigen Values & Eigen vectors
To find the Principal Components,
Eigen Values & Eigen vectors need to be computed
- on the covariance matrix
Principle Components
New variables are constructed as linear combinations or mixtures of the initial variables.
Computing the eigenvectors and ordering them by their eigenvalues in descending order.
Eg:
10-dimensional data gives you 10 principal components,
but PCA tries to put the maximum possible information in the first component,
then maximum remaining information in the second and so on.
Output:
Eigen Vector
- Directions of the axes where there is the most variance(most information)
Eigen Values
- Amount of variance carried in each Principal Component
Feature Vector
Where dimension reduction starts.
To choose whether to keep all these components or discard those of lesser eigenvalues to form a matrix with the remaining ones.
Feature Vector - Both eigenvectors
Feature Vector - Only one Eigenvectors
Recasting Data along the axes of Principal components
Reorient the data from the original axes to the ones represented by the principal components.
Formula - PCA
Final Dataset = [Feature Vector]^T * [Standardized Orginal Dataset]^T
Step 4: Fitting the PCA clustering π
To the dataset by specifying the Number of components to keep.
Git Repo: link
Step 5: Clustering via the Variance Percentage π
Screenshot for Output of Iris Clustering :
Thank you for joining me on this adventure π«. Don't forget to stay tuned for more exciting updates and discoveries π§‘. Let's continue to dive into the world of ML and embrace its magic to shape a brighter future for software development!
Until next time,
Sarangiβ€
Subscribe to my newsletter
Read articles from Sarangi Wijemanna directly inside your inbox. Subscribe to the newsletter, and don't miss out.
Written by
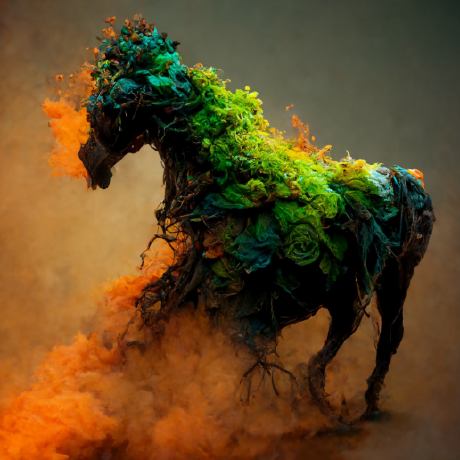
Sarangi Wijemanna
Sarangi Wijemanna
Hello there! π©βπ I am a dynamic and motivated graduate with a BSc. in Electronics and Telecommunication Engineering from SLTC Research University, Sri Lanka. With a keen interest in emerging technologies, I am currently honing my skills in Machine Learning (ML), Data Science (DS), and DevOps. Academic Background: BSc. Electronics and Telecommunication Engineering | SLTC Research University, Sri Lanka Professional Summary: As a results-driven individual, I am passionate about leveraging my exceptional analytical skills to drive innovation and achieve excellence in any project I undertake. My proactive and self-motivated approach enables me to efficiently manage multiple tasks in a fast-paced environment. Career Aspirations: My primary objective is to contribute my skills and knowledge to a forward-thinking organization that fosters a collaborative and growth-oriented environment. I aspire to be an integral part of a team that innovates, solves complex challenges, and positively impacts people's lives through cutting-edge technology.