Factors that Cause AI Projects to Fail

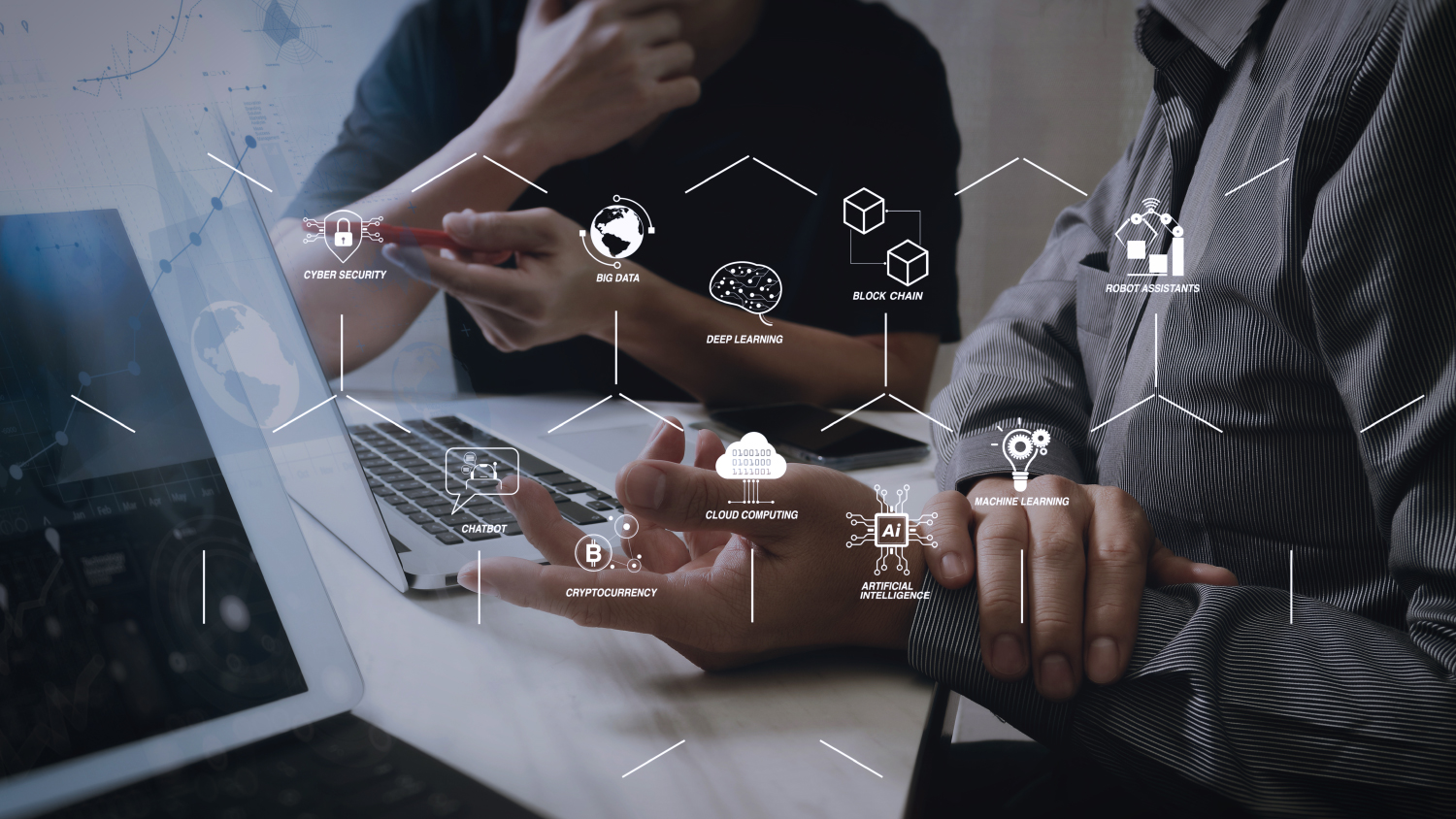
AI is making significant strides by offering remarkable efficiencies and innovative solutions. But, AI projects are often imperiled when firms fail to foresee the approaching challenges.
Due to these obstacles, many AI projects are jeopardized, hindering the firm's ability to harness the powers of AI models in enterprise software.
Flawed data management can severely compromise the AI applications' effectiveness. This leads to the development of inaccurate and unreliable machine-learning models. At the same time, it can jeopardize the integrity of AI projects, leading to wrong insights and flawed decision-making. These factors have far-reaching implications on a firm's strategic principles and operational efficiencies.
Acquiring the necessary data for AI solutions is challenging for firms lacking enterprise-wide data and data preparedness strategies. This complicates the process of achieving, cleansing and governing the data. Such issues can result in delivery delays and long-term solution failures.
Furthermore, timely access to quality data in sufficient quantities is vital for any AI-based solution to function. Hence, firms require a robust data strategy. The first step is implementing a cohesive plan to gather, prepare, and manage data from various business areas. By doing this, project teams will have access to relevant data that helps ensure regulatory and legal compliance at all levels.
Subscribe to my newsletter
Read articles from Sourabh directly inside your inbox. Subscribe to the newsletter, and don't miss out.
Written by

Sourabh
Sourabh
I am a Mechanical Engineering graduate with a Bachelor's degree. I was more interested in Digital Marketing so I started my current position at Ondot Media India Pvt. Ltd. as a Web Publisher and a Digital Marketer since January 2023. I have gained corporate experience by getting my hands on Digital Marketing, Content Marketing, and sales.