Quantum Machine Learning: The Exciting Intersection of Quantum Computing and AI
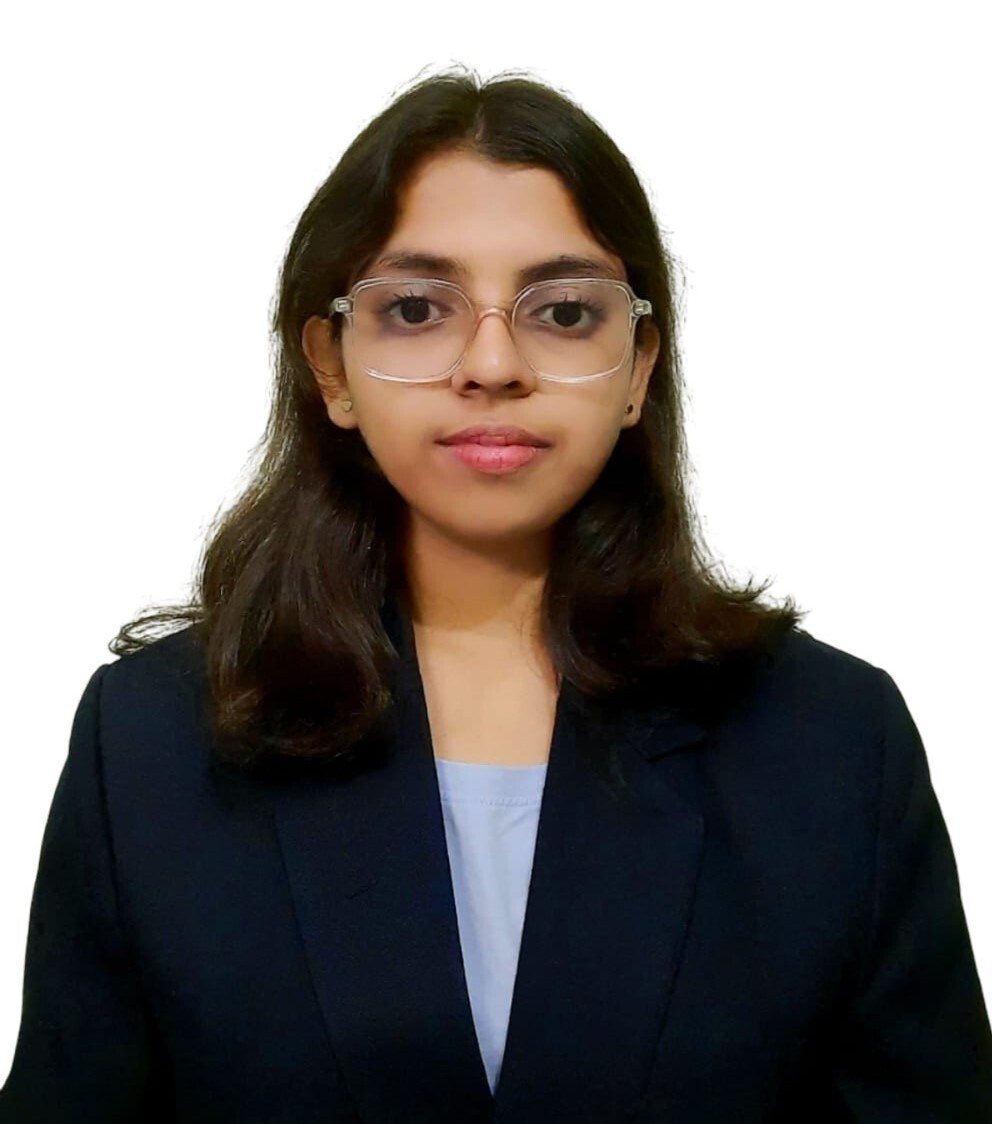
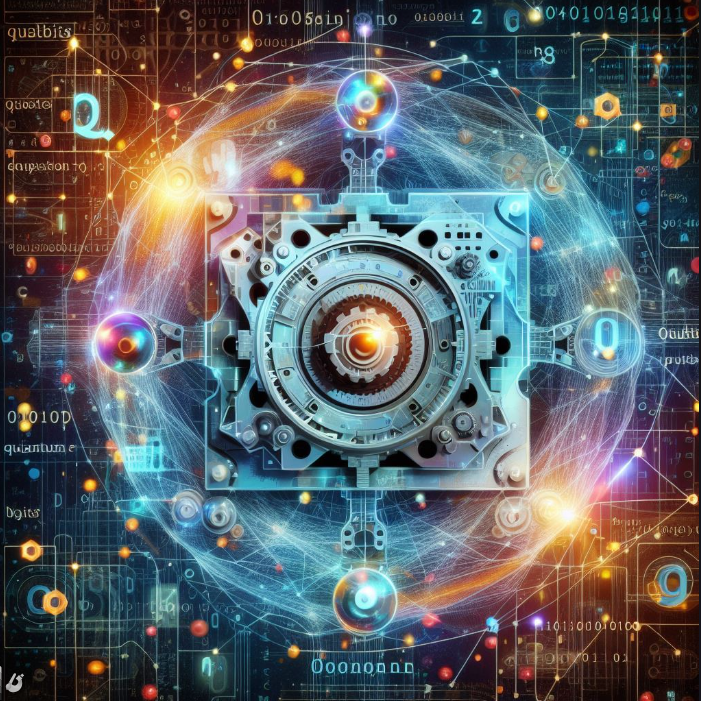
Step into the future where the frontiers of quantum computing and artificial intelligence converge, opening up possibilities that were once confined to the realms of science fiction. The emerging fields of quantum computing and artificial intelligence hold enormous promise for the future. As these technologies continue to develop rapidly, researchers are exploring how they can work together to create even more powerful applications. This has given rise to the fascinating domain of quantum machine learning. In this post, we'll dive into the basics of quantum ML (QML) and why it has researchers so excited.
Understanding Quantum Machine Learning
Quantum machine learning refers to machine learning algorithms and techniques designed to run on quantum computing hardware. It involves leveraging the unique properties of quantum physics to process data and solve problems faster and more efficiently than even the most powerful classical computers. Unlike classical computers that use bits (0s and 1s), quantum computers use qubits, which can exist in a superposition of states, enabling them to perform complex calculations in parallel.
Quantum Superposition: Picture qubits as the multitaskers of the quantum world, existing in multiple states simultaneously, allowing quantum computers to process a vast number of possibilities in parallel. This inherent parallelism provides a potential speedup in solving certain types of problems, including optimization and pattern recognition.
Quantum Entanglement: Entanglement is a unique quantum phenomenon where qubits become correlated and the state of one qubit instantaneously influences the state of another, regardless of the physical distance between them. Quantum algorithms can exploit entanglement to perform computations that classical computers find challenging.
Quantum Interference: Quantum interference allows quantum algorithms to amplify the probability of correct outcomes while diminishing the probability of incorrect ones. This interference is harnessed to enhance the efficiency of quantum machine learning algorithms.
Some of the key characteristics of quantum ML include:
Quantum Speedup - Certain quantum algorithms like Grover's search algorithm can achieve exponential speedups over classical counterparts for machine learning tasks like classification, regression, and clustering.
Novel Quantum Models - Quantum machine learning explores new quantum-based models like quantum neural networks, quantum Boltzmann machines, and quantum reinforcement learning.
Quantum Data - Quantum data has unique properties not found in classical data. Quantum ML aims to process and learn from such quantum data sets.
Why is it Important?
Quantum machine learning has attracted so much attention because it taps into the most transformative technologies of our time - quantum computing and AI. It brings together the strengths of both fields to potentially create unprecedented capabilities in areas like optimization, sampling, search and pattern recognition. Key potential benefits include:
Handling Complex Data - Quantum models may be better able to process complex, high-dimensional data like images, video, and molecular structures.
Advances in AI - Quantum algorithms could enable AI and deep learning models that are exponentially more powerful.
Real-World Impact - Applications of quantum ML range from quantum chemistry to finance, healthcare, cybersecurity and more.
Scientific Insights - Studying quantum learning sheds light on the foundations of physics, computation, and the learning process itself.
Applications of Quantum Machine Learning:
Drug Discovery: Quantum machine learning can accelerate the process of simulating molecular interactions, aiding in drug discovery and development.
Financial Modeling: Quantum algorithms may enhance the efficiency of complex financial modeling and optimization tasks, impacting areas such as portfolio optimization and risk management.
Cryptography: Quantum machine learning holds the potential to revolutionize cryptography by developing quantum-resistant algorithms and enhancing the security of communication protocols.
The Future of Quantum Machine Learning
Quantum machine learning is still in its infancy. Realizing its full potential will require overcoming hardware challenges and continuing foundational research. But pioneering work is already underway at organizations like Google, IBM, Microsoft, D-Wave, Rigetti and IonQ. As quantum computers scale up and become more practical in the coming years, expect to see quantum machine learning have a profound impact across many fields. The future is bright at the intersection of quantum computing and artificial intelligence!
Subscribe to my newsletter
Read articles from Trisha Banerjee directly inside your inbox. Subscribe to the newsletter, and don't miss out.
Written by
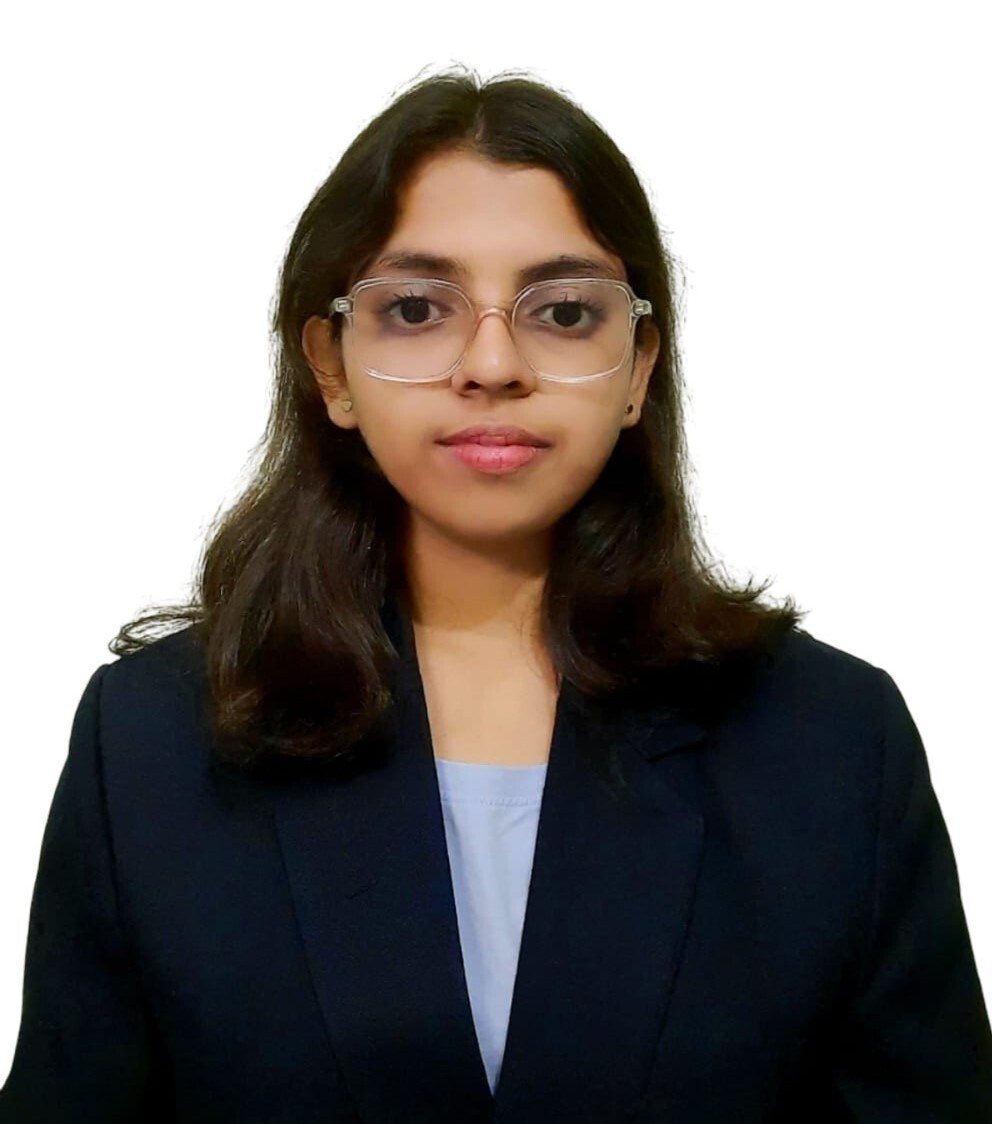
Trisha Banerjee
Trisha Banerjee
I'm Trisha, an enthusiastic AI/ML student with an insatiable curiosity for deciphering the intricacies of data. Currently immersed in the exhilarating journey of evolving into a Data Scientist, I harmonize the artistry of machine learning with the precision of AI. In the expansive realm of data science, my passions revolve around unlocking the transformative potential inherent in AI and ML. I firmly believe in the profound power of data to illuminate insights and drive informed decision-making. Join me as I navigate the dynamic landscape of artificial intelligence, embracing the endless possibilities that arise when passion meets data-driven exploration.