Sample Ratio Mismatch (SRM) Cheatsheet: Understanding and Addressing Experimental Discrepancies
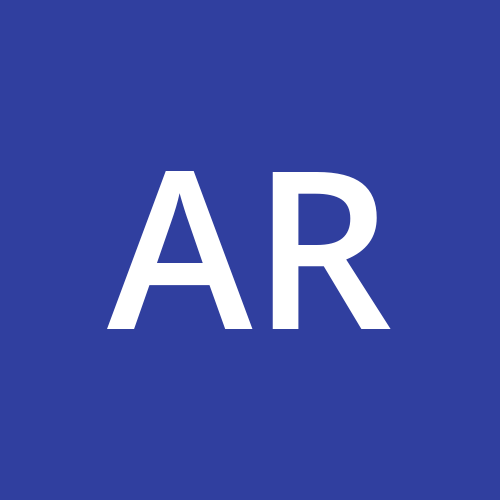
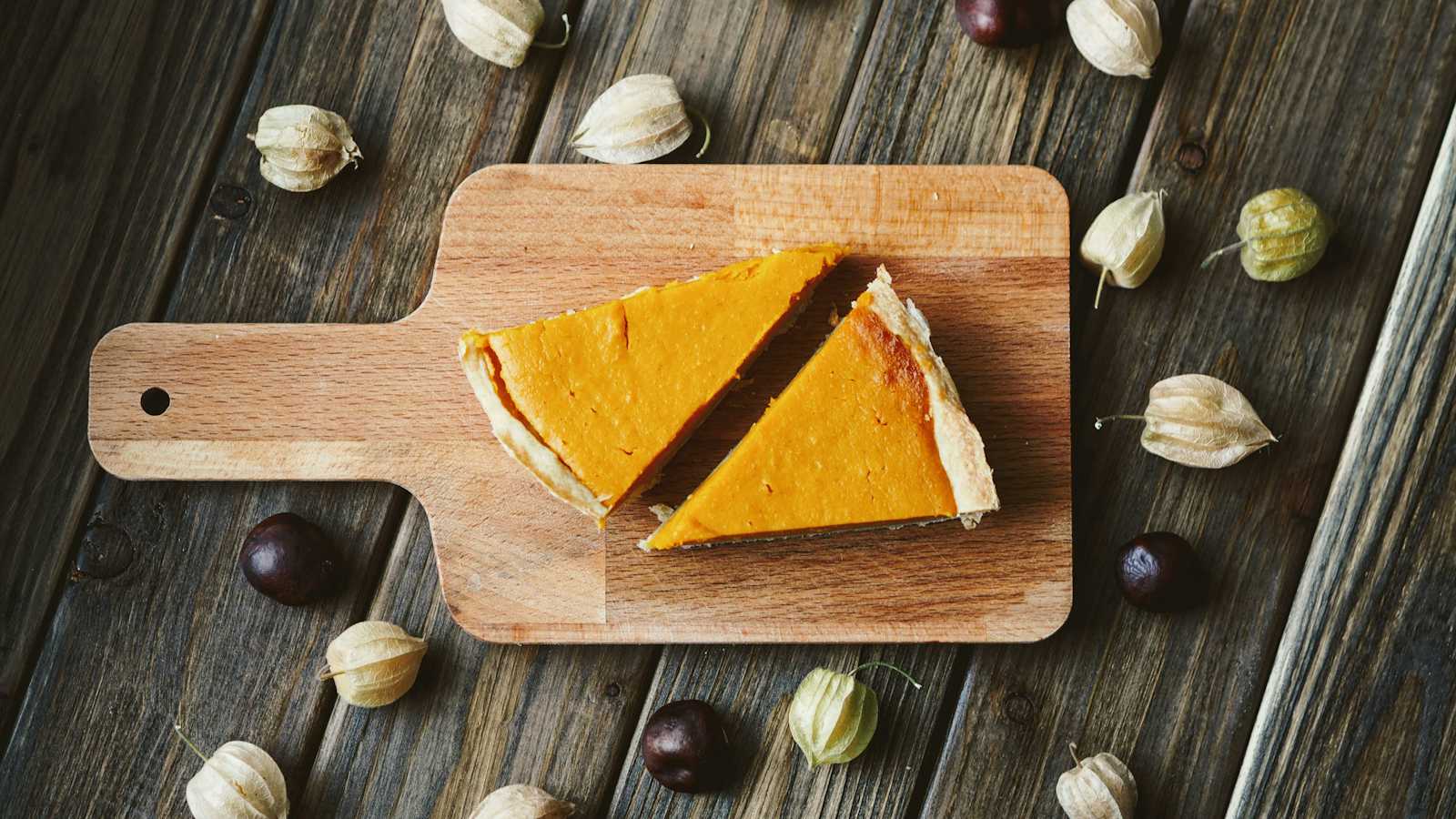
Sample Ratio Mismatch (SRM) refers to the difference between how we planned to split participants in an experiment and how it actually turned out. For instance, if we intended a 50/50 split but ended up with a 60/40 distribution, that's an SRM.
SRMs bring in a form of bias that has the potential to render the results of an experiment entirely unreliable. Essentially, they skew the findings by introducing a selection bias that can significantly impact the accuracy of our conclusions.
Impact
SRMs are widespread, impacting more than 6% of experiments, particularly in well-established organizations. This percentage highlights the significance of comprehending and addressing SRMs to guarantee the trustworthiness of experimental outcomes. It emphasizes the need to manage and reduce SRMs for more dependable and meaningful results..
Five Common Types of Experiment SRMs
Execution
Variant Delivery: Variants starting at different times.
Variant Execution: Delayed filtered execution.
Telemetry Generation: Redirecting only some variants, telemetry addition/removal, performance or engagement impact, product crashes, client caching behavior, and telemetry transmission issues.
Assignment
Variant Assignment: Issues such as incorrect bucketing, faulty randomization functions, corrupted User IDs, and carry-over effects.
Variant Deployment: Non-orthogonal experiments and interaction effects.
Log Processing
- Telemetry Cooking: Involves removal of bots, incorrect joins, and delayed log arrivals.
Analysis
- Telemetry Filtering: Incorrect starting-point of analysis, missing counterfactual logging, and wrong triggering or filtering conditions.
Interference
Variant Interference: Inconsistent ramping of variants, pausing variants during execution, and self-assigning into a variant.
Telemetry Interference: Involves injection attacks and hacks.
Root-Cause Checklist
Certainly! Let's delve into more context for each of the points in the checklist:
1. Scorecards:
- Examine whether the Sample Ratio Mismatch (SRM) is apparent only in a subset of the data triggered or filtered during the analysis. This could point to an issue with the way the experiment is being analyzed, such as an improper filtering criterion or an analysis factor that may be influencing the results.
2. User Segments:
- Evaluate if SRM is specific to a particular group of users. This insight can highlight user segment-specific factors, like preferences or behaviors, that might be contributing to the observed SRM.
3. Time Segments:
- Investigate whether SRM is limited to specific time periods, like the first day of the experiment. This could suggest time-related factors influencing the experiment setup or execution.
4. Performance Metrics:
- Analyze if there's a substantial decline in performance metrics, such as increased time-to-load. This may indicate a genuine impact on the system, validating the existence of SRM.
5. Engagement Metrics:
- Assess whether user engagement is showing a noticeable trend, either increasing or decreasing. This observation can indicate the effect of SRM on different user engagement levels.
6. SRM Frequency:
- Determine if SRM is consistently observed across multiple experiments. If so, it could point to a systematic issue in the experimental setup, execution, or analysis that needs attention.
7. AA Experiments:
- Check if SRM occurs in AA (Control vs. Control) experiments. If SRM is present in AA experiments, it may indicate a systematic issue rather than an actual treatment effect.
8. Severity:
- Consider the magnitude of SRM. A very large or very small SRM may provide insights into whether it's a control or treatment issue, helping to understand the scale of the problem.
9. Downstream:
- Examine whether SRM is isolated to a specific step in your data processing pipeline. Understanding where SRM occurs downstream can offer valuable clues about the origin and nature of the issue.
10. Cross-Pipeline:
- Explore whether SRM is visible only in a specific pipeline or if it appears consistently across multiple pipelines. This comparison provides additional context for analysis, helping to discern whether the issue is localized or pervasive.
Use this comprehensive cheatsheet to identify, understand, and address Sample Ratio Mismatches in your experiments. By systematically exploring these factors, you can enhance the robustness and reliability of your experimental outcomes.
Source
Fabijan, Aleksander & Gupchup, Jayant & Gupta, Somit & Omhover, Jeff & Qin, Wen & Vermeer, Lukas & Dmitriev, Pavel. (2019). Diagnosing Sample Ratio Mismatch in Online Controlled Experiments: A Taxonomy and Rules of Thumb for Practitioners. 2156-2164. 10.1145/3292500.3330722.
Subscribe to my newsletter
Read articles from Arvindh directly inside your inbox. Subscribe to the newsletter, and don't miss out.
Written by
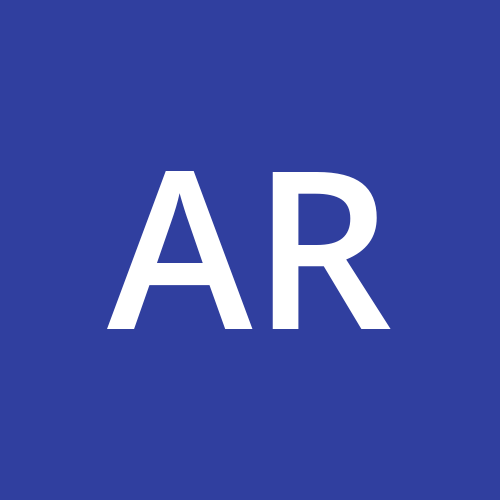
Arvindh
Arvindh
I am Sr Data Scientist at Target, working at the intersection of optimization and machine learning. I got my Masters in Business Analytics from University of Texas at Dallas. 💻 Currently in pursuit to learn more about Deep Learning and Generative AI You can reach me at : https://www.linkedin.com/in/arvindh-arul/