9 Easy Steps to Create an Optimization Model in Python!
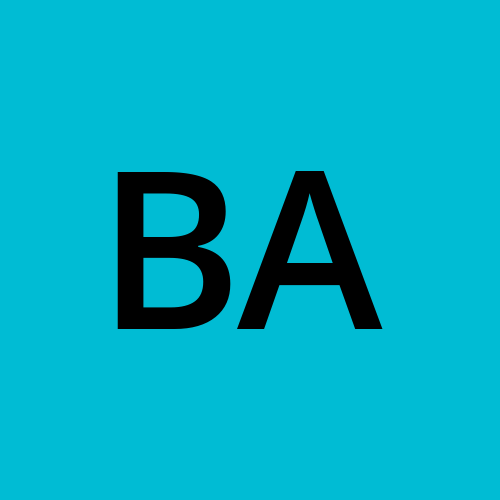
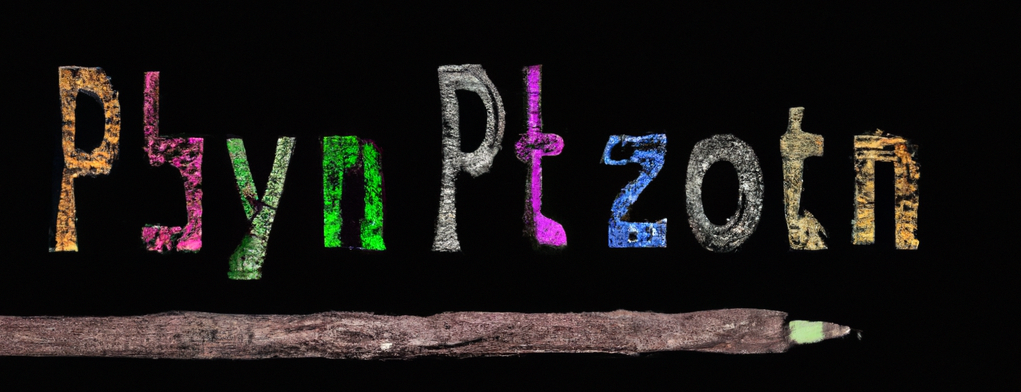
Determining the optimal design and operation of a system often involves employing quantitative methods for decision-making, particularly in situations where resources are limited. Mathematical optimization serves as a primary approach for determining the best course of action in such scenarios. It entails the maximization or minimization of a real function by systematically selecting input values from a defined set and calculating the resulting value of the objective function.
Various applications of optimization include:
Product planning and inventory management: Strategically issuing orders to prevent stock-outs and avoid exceeding capacity constraints.
Routing decisions: Determining the most cost-effective routes for transportation or delivery.
Packing problems: Deciding on the most efficient packing method while adhering to capacity limits and minimizing wasted space.
Resource allocation: Determining the optimal distribution of resources and materials.
Scheduling: Planning shifts for workers to maximize efficiency and meet operational demands.
Location problems: Identifying optimal facility placements to minimize transportation costs and satisfy demand requirements.
If you are into Optimization/Operations Research, you can leverage the Python library Pyomo for modeling and solving optimization problems. Here are the 9 steps to start:
Install Pyomo:
Make sure you have Pyomo installed in your Python environment. If not, you can install it via pip:
pip install pyomo
Import Pyomo Modules:
Import the necessary modules from Pyomo to define and solve optimization models:
from pyomo.environ import ConcreteModel, Var, Objective, Constraint, SolverFactory
Define a Concrete Model:
Create a concrete model to contain decision variables, objective function, and constraints:
model = ConcreteModel()
Define Decision Variables:
Define decision variables that represent the unknowns in your optimization problem:
model.x = Var()
Define Objective Function:
Specify the objective function that needs to be minimized or maximized:
model.obj = Objective(expr=2 * model.x)
Define Constraints:
Add constraints to restrict the feasible region of the decision variables:
model.constraint = Constraint(expr=model.x <= 5)
Choose the Solver:
Select an appropriate solver to solve the optimization problem. Here, we're using the GLPK solver:
solver = SolverFactory('glpk')
Solve the Optimization Problem:
Use the chosen solver to solve the optimization model and find the optimal solution:
solver.solve(model)
Access Solution Results:
Once the optimization problem is solved, access and interpret the results:
print("Optimal value of x:", model.x())
You can use this basic guide and import these commands to a Python script or a Jupyter Notebook and start creating your optimization models in Python 🐍.
Putting everything together:
# 1️⃣ Install Pyomo
# pip install pyomo
# 2️⃣ Import Pyomo Modules
from pyomo.environ import ConcreteModel, Var, Objective, Constraint, SolverFactory
# 3️⃣ Define a Concrete Model
model = ConcreteModel()
# 4️⃣ Define Decision Variables
model.x = Var()
# 5️⃣ Define Objective Function
model.obj = Objective(expr=2 * model.x)
# 6️⃣ Define Constraints
model.constraint = Constraint(expr=model.x <= 5)
# 7️⃣ Choose the Solver
solver = SolverFactory('glpk')
# 8️⃣ Solve the Optimization Problem
solver.solve(model)
# 9️⃣ Access Solution Results
print("Optimal value of x:", model.x())
# Additional Example Problem:
# Maximizing 3x + 4y
# Subject to:
# x + y <= 5
# 2x + 3y <= 10
# x, y >= 0
# Define Concrete Model
model = ConcreteModel()
# Define Decision Variables
model.x = Var(domain=NonNegativeReals)
model.y = Var(domain=NonNegativeReals)
# Define Objective Function
model.obj = Objective(expr=3 * model.x + 4 * model.y, sense=maximize)
# Define Constraints
model.constraint1 = Constraint(expr=model.x + model.y <= 5)
model.constraint2 = Constraint(expr=2 * model.x + 3 * model.y <= 10)
# Choose the Solver
solver = SolverFactory('glpk')
# Solve the Optimization Problem
solver.solve(model)
# Access Solution Results
print("Optimal value of x:", model.x())
print("Optimal value of y:", model.y())
print("Optimal objective value:", model.obj())
This text provides a step-by-step guide to creating and solving optimization models in Python using Pyomo, accompanied by the code snippets for each step.
Subscribe to my newsletter
Read articles from Behsys Analytics directly inside your inbox. Subscribe to the newsletter, and don't miss out.
Written by
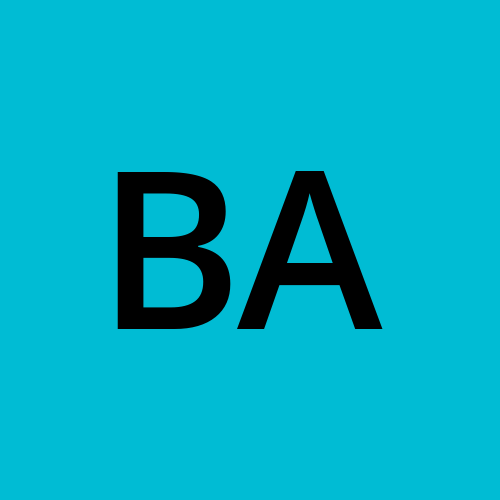