How Data Annotation drives precise AI Video Analytics
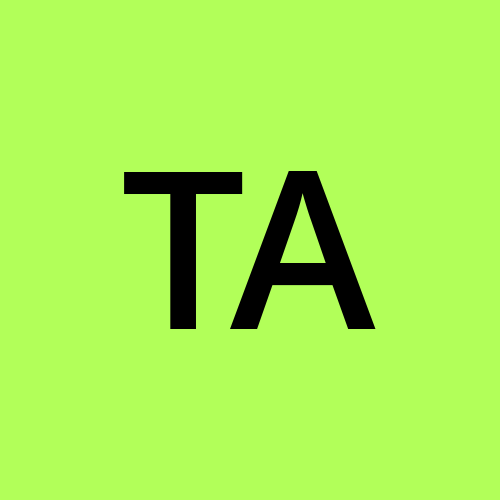
In the era of data-driven insights and intelligent automation, video analytics has emerged as a transformative technology, revolutionizing the way we extract valuable information from video data. At the heart of this innovation lies the power of artificial intelligence (AI) and its ability to analyze and interpret video content with remarkable accuracy and speed. Welcome to our blog, where we delve into the different applications of AI-powered video analytics and the use of data annotation to train these applications.
What is AI-powered video analytics?
AI-powered video analytics refers to using artificial intelligence (AI) techniques and algorithms to analyze video content and extract valuable insights and information from it. It involves the application of computer vision, machine learning, and deep learning algorithms to analyze video data in real time or post-processing.
With Video analytics, computers can understand and interpret the content of videos, enabling a wide range of applications such as surveillance, security, object detection and tracking, facial recognition, crowd monitoring, anomaly detection, and more. The AI algorithms can automatically detect and classify objects, recognize faces and actions, track movements, identify patterns, and generate actionable insights from video data.
By leveraging AI technologies, video analytics systems can process vast amounts of video data efficiently and accurately, enabling faster and more reliable analysis compared to manual human efforts. It allows organizations to extract valuable information from video footage, improve situational awareness, enhance security measures, optimize operations, and make data-driven decisions based on the insights obtained from the analyzed videos.
AI-powered video analytics has numerous applications across various industries, including public safety, retail, transportation, smart cities, healthcare, and entertainment. It offers advanced capabilities for video understanding, enabling organizations to unlock the full potential of their video data and leverage it for improved efficiency, safety, and decision-making.
Applications of AI-Powered Video Analytics:
From enhancing public safety and security through advanced surveillance systems to improving customer experience and optimizing operations in retail environments, organizations are leveraging this technology to gain invaluable insights from their video data. Let's explore some of the key applications of AI video analytics:
Intrusion Detection: AI-powered video analytics can automatically detect and alert security personnel in real time when unauthorized individuals or objects enter restricted areas. Combining object detection and tracking algorithms, it enhances security and reduces response time to potential threats.
Public Place Security: Video analytics can enhance security in public places such as airports, train stations, and stadiums by automatically detecting suspicious behavior, abandoned objects, or unauthorized access. This technology enables proactive measures to ensure public safety and prevent potential incidents.
Investigation and Forensics: AI video analytics assists law enforcement agencies and investigators in analyzing surveillance footage to identify suspects, track their movements, and reconstruct events. It accelerates investigations by automating the process of extracting relevant information from video data.
Crowding and Density Monitoring: Video analytics can analyze video feeds to determine crowd size, density, and movement patterns in public areas or events. This information helps authorities optimize crowd management, ensure safety, and prevent overcrowding.
Traffic Monitoring and Management: AI-powered video analytics can analyze traffic flow, detect congestion, and monitor incidents in real time. By providing accurate data on traffic patterns and identifying bottlenecks, it enables efficient traffic management and optimization of transportation systems.
Object Recognition and Tracking: Video analytics algorithms can detect and track specific objects of interest, such as vehicles, people, or animals. This capability is valuable in applications like inventory tracking, wildlife monitoring, or tracking assets in logistics and supply chain management.
Behavioral Analysis: AI video analytics can analyze human behavior in video footage, identifying abnormal or suspicious activities. This capability is crucial for ensuring safety in high-security environments, detecting potential threats, and preventing criminal activities.
Retail Analytics: Video analytics can analyze customer behavior and engagement in retail environments. It can track footfall, identify customer demographics, measure customer engagement with displays, and optimize store layouts to enhance the shopping experience and drive business insights.
Data Annotation for AI video analytics
Data collection and annotation are vital components in the development of AI video analytics. The process begins with collecting diverse and representative video data from various sources, including surveillance cameras, drones, or other recording devices. This data is then carefully curated and preprocessed to ensure its quality and relevance. The collected video data undergoes annotation, where human annotators or AI algorithms label and annotate specific objects, events, or attributes within the video frames. This annotation process can include tasks such as object detection, tracking, classification, segmentation, and activity recognition.
Data annotation plays a crucial role in training models for AI-powered video analytics. Here's why data annotation is important in this context:
Training Data Preparation: To build an effective AI model for video analytics, a large and diverse dataset is required. Data annotation involves the process of labeling or annotating the video data with relevant information, such as object bounding boxes, action recognition, or event labels. This annotated data serves as the foundation for training the AI model.
Accuracy and Precision: Data annotation ensures that the labeled information is accurate and precise, providing ground truth annotations for the AI model. This accuracy is essential for the model to learn and make accurate predictions on unseen video data. Proper annotation reduces the chances of misinterpretation and helps in generating reliable insights from the video analytics system.
Object Detection and Tracking: Video analytics often involves tasks like object detection and tracking, where the AI model needs to identify and track specific objects or individuals in the video footage. Data annotation helps in creating labeled datasets that contain annotated bounding boxes around the objects of interest. This annotated data enables the model to learn and recognize different objects accurately.
Action Recognition: In video analytics, understanding and recognizing human actions or events is vital. Data annotation allows for labeling specific actions or events occurring in the video, providing the necessary information for the AI model to learn the patterns and characteristics of various actions. This enables the model to recognize and classify actions in real time.
Performance Evaluation: Data annotation also plays a significant role in evaluating the performance of AI models for video analytics. Annotated datasets serve as ground truth data to measure the accuracy and performance of the model against specific metrics. The annotations act as a benchmark for evaluating the model's ability to detect, track, classify, or analyze video content.
By providing accurate and well-annotated training data, data annotation enables the AI model to learn and generalize from the labeled examples. This process enhances the model's ability to detect objects, recognize actions, and extract meaningful insights from video data. Quality data annotation ensures the reliability and effectiveness of AI-powered video analytics systems, making them capable of delivering valuable insights and actionable information for various applications such as surveillance, security, crowd monitoring, and more.
Conclusion
As AI continues to push the boundaries of what is possible, AI-powered video analytics emerges as a game-changer in harnessing the wealth of information hidden within video data. From improving safety and security to enabling data-driven decision-making, the applications of this technology are vast and transformative.
TagX specializes in providing precise data annotation services for AI video analytics. We have the expertise to accurately label video data, enabling AI models to detect objects, track movements, recognize actions, and analyze events. Our team ensures that every frame is annotated with meticulous attention to detail, ensuring high-quality training data for AI video analytics applications. Together, let's unlock the power of AI and transform the way we analyze and leverage video data for a smarter and more secure future.
Subscribe to my newsletter
Read articles from tagx directly inside your inbox. Subscribe to the newsletter, and don't miss out.
Written by
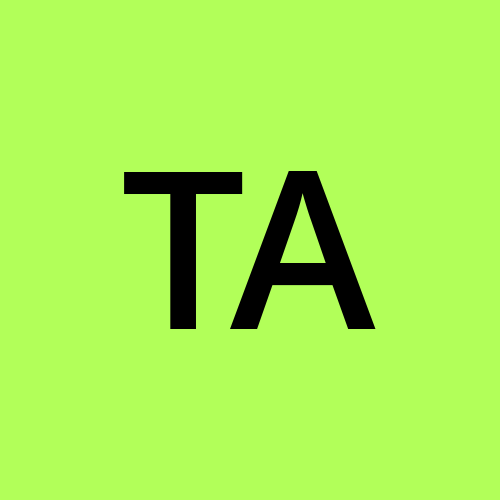