Riding the Wave: Unveiling Nesterov Accelerated Gradient in Deep Learning

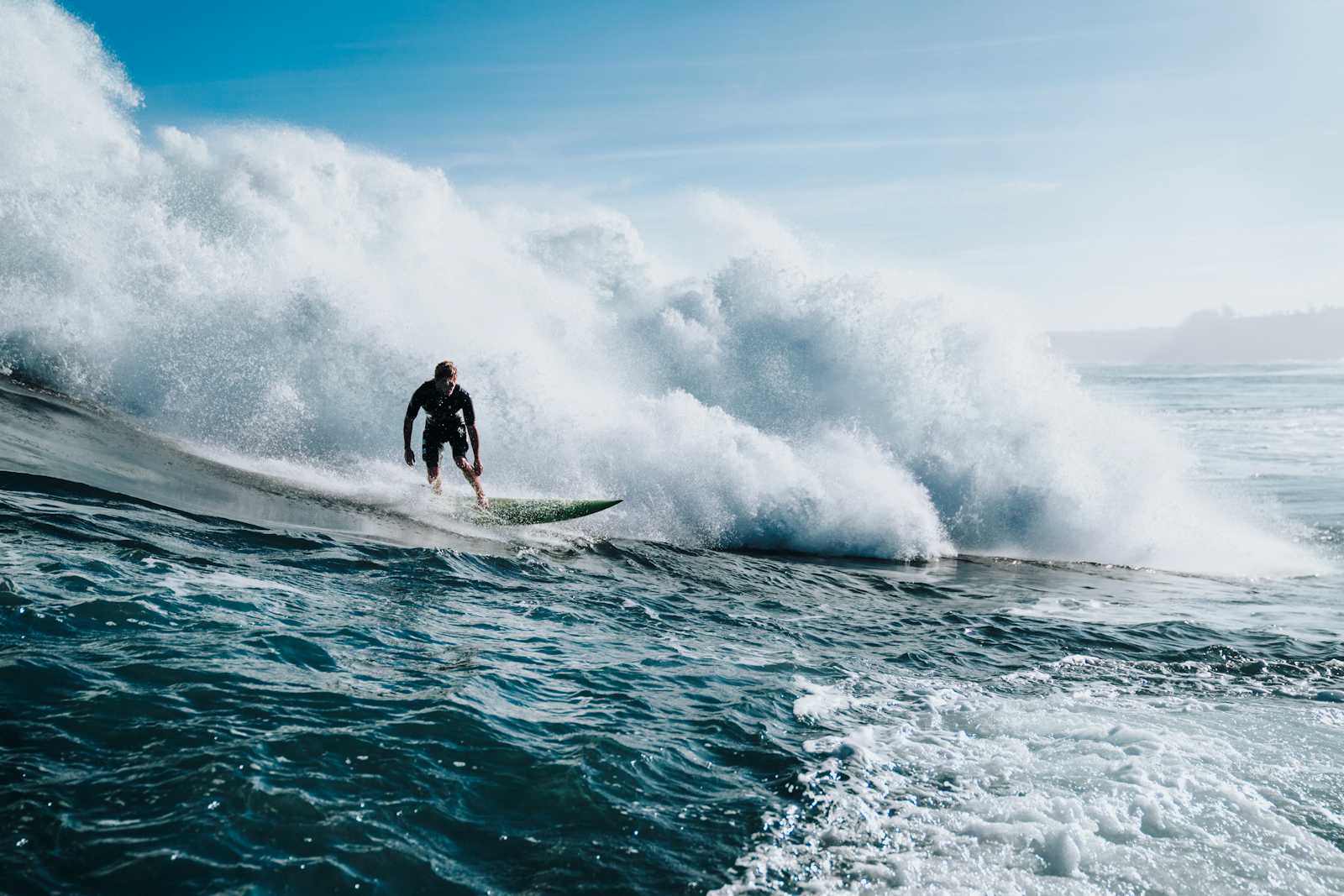
Introduction:
In the ever-evolving landscape of deep learning optimization algorithms, Nesterov Accelerated Gradient (NAG) emerges as a powerful contender. This blog post unravels the intricacies of Nesterov Accelerated Gradient, providing insights into its mathematical foundations, a comparison with Momentum Optimization, and a practical guide through advantages, disadvantages, and implementation using Python.
What is Nesterov Accelerated Gradient:
Explanation:
Nesterov Accelerated Gradient is an optimization technique that tweaks the classic gradient descent by incorporating information about the future position of the parameters. It does this by making a "look-ahead" update before calculating the gradient.
Mathematical Intuition for Nesterov Accelerated Gradient:
Formula:
[ \(v_t = \beta \cdot v_{t-1} + \alpha \cdot \nabla J(\theta_t - \beta \cdot v_{t-1}) ] [ \theta_{t+1} = \theta_t - v_t\) ]
Comparison with Momentum Optimization:
Nesterov Accelerated Gradient adjusts the traditional momentum update by incorporating the gradient at the "look-ahead" position, making it more accurate in predicting the future direction compared to Momentum Optimization.
Advantages and Disadvantages of Nesterov Accelerated Gradient:
Advantages:
Faster Convergence: NAG often converges faster than traditional gradient descent.
- Improved Precision: The "look-ahead" mechanism enhances accuracy in determining the optimal parameter updates.
Disadvantages:
Hyperparameter Sensitivity: Like many optimization algorithms, the performance of NAG is sensitive to hyperparameter tuning.
- Potential Overshooting: In certain scenarios, NAG might overshoot the optimal point, causing oscillations.
Python Code for Implementing Nesterov Accelerated Gradient:
import numpy as np
def nesterov_accelerated_gradient(data, alpha, beta):
theta = data[0]
v = 0
for i in range(1, len(data)):
lookahead_position = theta - beta * v
gradient_at_lookahead = gradient_of_loss(lookahead_position) # Replace with your gradient calculation
v = beta * v + alpha * gradient_at_lookahead
theta = theta - v
return theta
# Example Usage
dataset = [10, 12, 15, 18, 22]
learning_rate = 0.1
momentum_term = 0.9
result_theta = nesterov_accelerated_gradient(dataset, learning_rate, momentum_term)
print("Optimal Parameters:", result_theta)
Summary:
Nesterov Accelerated Gradient emerges as a dynamic optimization technique, providing a glimpse into the future to refine parameter updates. Through a mathematical journey and a comparison with Momentum Optimization, we've explored the advantages and disadvantages of NAG. By concluding with a Python implementation, this blog equips practitioners with the knowledge to leverage Nesterov Accelerated Gradient effectively in their quest for optimal neural network training.
Subscribe to my newsletter
Read articles from Saurabh Naik directly inside your inbox. Subscribe to the newsletter, and don't miss out.
Written by

Saurabh Naik
Saurabh Naik
๐ Passionate Data Enthusiast and Problem Solver ๐ค ๐ Education: Bachelor's in Engineering (Information Technology), Vidyalankar Institute of Technology, Mumbai (2021) ๐จโ๐ป Professional Experience: Over 2 years in startups and MNCs, honing skills in Data Science, Data Engineering, and problem-solving. Worked with cutting-edge technologies and libraries: Keras, PyTorch, sci-kit learn, DVC, MLflow, OpenAI, Hugging Face, Tensorflow. Proficient in SQL and NoSQL databases: MySQL, Postgres, Cassandra. ๐ Skills Highlights: Data Science: Statistics, Machine Learning, Deep Learning, NLP, Generative AI, Data Analysis, MLOps. Tools & Technologies: Python (modular coding), Git & GitHub, Data Pipelining & Analysis, AWS (Lambda, SQS, Sagemaker, CodePipeline, EC2, ECR, API Gateway), Apache Airflow. Flask, Django and streamlit web frameworks for python. Soft Skills: Critical Thinking, Analytical Problem-solving, Communication, English Proficiency. ๐ก Initiatives: Passionate about community engagement; sharing knowledge through accessible technical blogs and linkedin posts. Completed Data Scientist internships at WebEmps and iNeuron Intelligence Pvt Ltd and Ungray Pvt Ltd. successfully. ๐ Next Chapter: Pursuing a career in Data Science, with a keen interest in broadening horizons through international opportunities. Currently relocating to Australia, eligible for relevant work visas & residence, working with a licensed immigration adviser and actively exploring new opportunities & interviews. ๐ Let's Connect! Open to collaborations, discussions, and the exciting challenges that data-driven opportunities bring. Reach out for a conversation on Data Science, technology, or potential collaborations! Email: naiksaurabhd@gmail.com