Data Annotation: How it Can Boost Your AI Models?
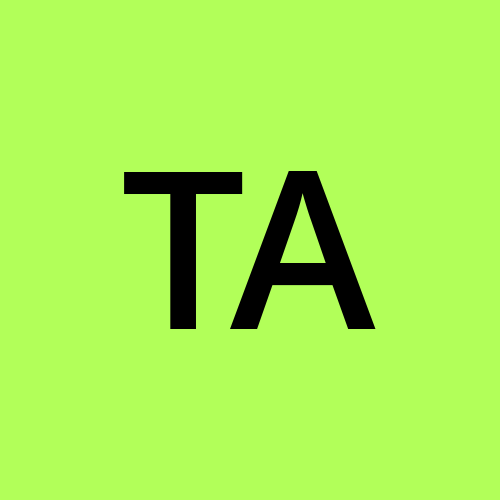
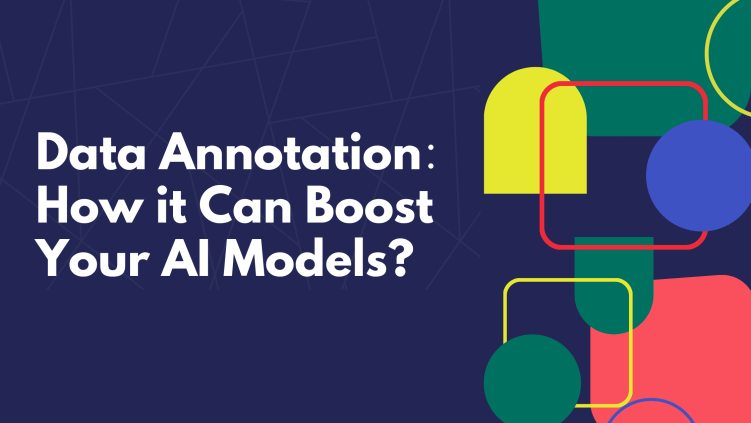
As artificial intelligence (AI) continues to revolutionize various industries, data annotation has become an essential part of the process. Essentially, data annotation involves labeling data to make it usable for machine learning algorithms. By providing the right annotations, you can train your AI models to recognize patterns, classify data, and make accurate predictions. In this context, data annotation is more than just a technical process. It's a way to enhance the quality and reliability of your AI models, while also ensuring that they're optimized for specific use cases.
What is Data Annotation?
Data annotation is the process of labeling data to make it usable for machine learning models. This labeling can be done manually or automatically, depending on the type of data and the desired outcome.
Data annotation is an essential step in the machine learning pipeline since it provides the necessary input for the model to learn from. Data annotation can be applied to many types of data, including text, images, audio, and video. The labeling process can vary depending on the type of data, but the goal is always the same: to provide a clear and consistent label that the model can use to learn from.
The Importance of High-Quality Data Annotation
The quality of data annotation is crucial for the accuracy of machine learning models. Poorly labeled data can lead to inaccurate models, which can be costly and time-consuming to fix. High-quality data annotation can help to reduce errors, increase accuracy, and improve the overall performance of the machine learning model.
One of the challenges of data annotation is ensuring that the labels are consistent across the dataset. Inconsistencies in labeling can lead to confusion for the model, resulting in errors and reduced accuracy. To ensure high-quality data annotation, it is essential to have clear guidelines and standards for labeling, as well as a system for quality control.
Different Types of Data Annotation Techniques
There are different types of data annotation techniques that can be used depending on the type of data and the desired outcome. Some of the most common techniques include:
Image Annotation: This involves labeling images with bounding boxes, segmentation masks, or key-points.
Text Annotation: This involves labeling text with entities, relationships, or sentiment analysis.
Audio Annotation: This involves labeling audio with phonemes, words, or speaker identification.
Video Annotation: This involves labeling video with action recognition, object tracking, or scene segmentation.
Each type of data annotation requires a different set of skills and tools, but the goal is always the same: to provide a clear and consistent label for the model to learn from.
Challenges with Data Annotation
Data annotation can be a challenging task, especially when dealing with large datasets or complex labeling requirements. Some of the challenges with data annotation include:
Time-Consuming: Data annotation can be a time-consuming process, especially when dealing with large datasets. This can be a bottleneck in the machine learning pipeline, slowing down the development process.
Costly: Depending on the type of data and labeling requirements, data annotation can be a costly process. This can be a significant barrier to entry for some organizations.
Quality Control: Ensuring the quality of data annotation can be a challenge, especially when dealing with multiple annotators or complex labeling requirements. Quality control measures must be in place to ensure consistency and accuracy.
Tools for Data Annotation
There are many tools available for data annotation, ranging from open-source software to commercial solutions. Some of the most popular tools include:
Labelbox: A commercial platform for data labeling and management.
LabelImg: An open-source graphical image annotation tool.
Prodigy: A commercial platform for data annotation and machine learning.
Each tool has its own set of features and capabilities, so it is essential to choose the right tool for your specific needs.
How Data Annotation Can Improve AI Models?
Data annotation can significantly improve the accuracy of AI models. By providing clear and consistent labels, the model can learn more effectively and produce better results. Some of the ways that data annotation can improve AI models include:
Increased Accuracy: With high-quality data annotation, AI models can achieve higher accuracy rates, reducing errors and improving performance.
Faster Development: With clear labeling guidelines and quality control measures, the development process can be streamlined, reducing the time and cost of development.
Improved User Experience: By improving the accuracy of AI models, the user experience can be enhanced, leading to increased satisfaction and engagement.
Applications of Data Annotation in Various Industries
Data annotation has applications in various industries, including healthcare, finance, retail, and more. Some of the ways that data annotation is being used in these industries include:
Healthcare: Data annotation is being used to improve the accuracy of medical diagnosis and treatment.
Finance: Data annotation is being used to improve fraud detection and risk management.
Retail: Data annotation is being used to improve product recommendations and customer experience.
By providing clear and consistent labeling, data annotation can help to improve the accuracy and effectiveness of AI models in these industries and many others.
Best Practices for Data Annotation
To ensure high-quality data annotation, it is essential to follow best practices. Some of the best practices for data annotation include:
Clear Guidelines: Provide clear labeling guidelines and standards to ensure consistency across the dataset.
Quality Control: Implement quality control measures, such as double-checking labels or using inter-annotator agreement measures.
Training: Provide training to annotators to ensure they understand the labeling guidelines and can provide accurate and consistent labeling.
By following these best practices, the quality of data annotation can be improved, leading to better machine learning models.
Conclusion
Data annotation is a crucial step in the machine learning pipeline, providing the necessary input for models to learn from. By following best practices and using the right tools and services, high-quality data annotation can be achieved, leading to more accurate and effective AI models. With the power of data annotation, organizations can unlock the full potential of machine learning and AI, improving the user experience, reducing errors, and increasing efficiency.
So, what are you waiting for? Start exploring the power of data annotation and unlock the full potential of your AI models.
Subscribe to my newsletter
Read articles from tagx directly inside your inbox. Subscribe to the newsletter, and don't miss out.
Written by
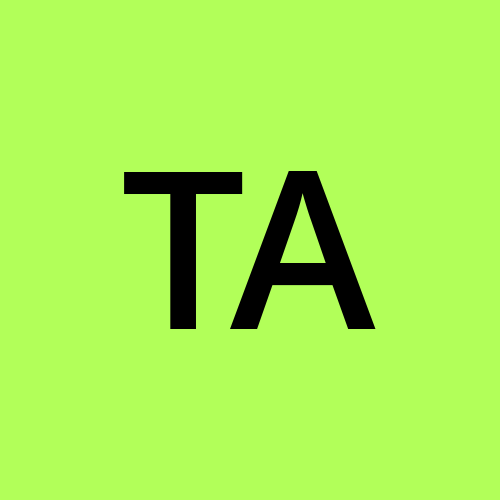