A Closer Look at Explainable AI (XAI)
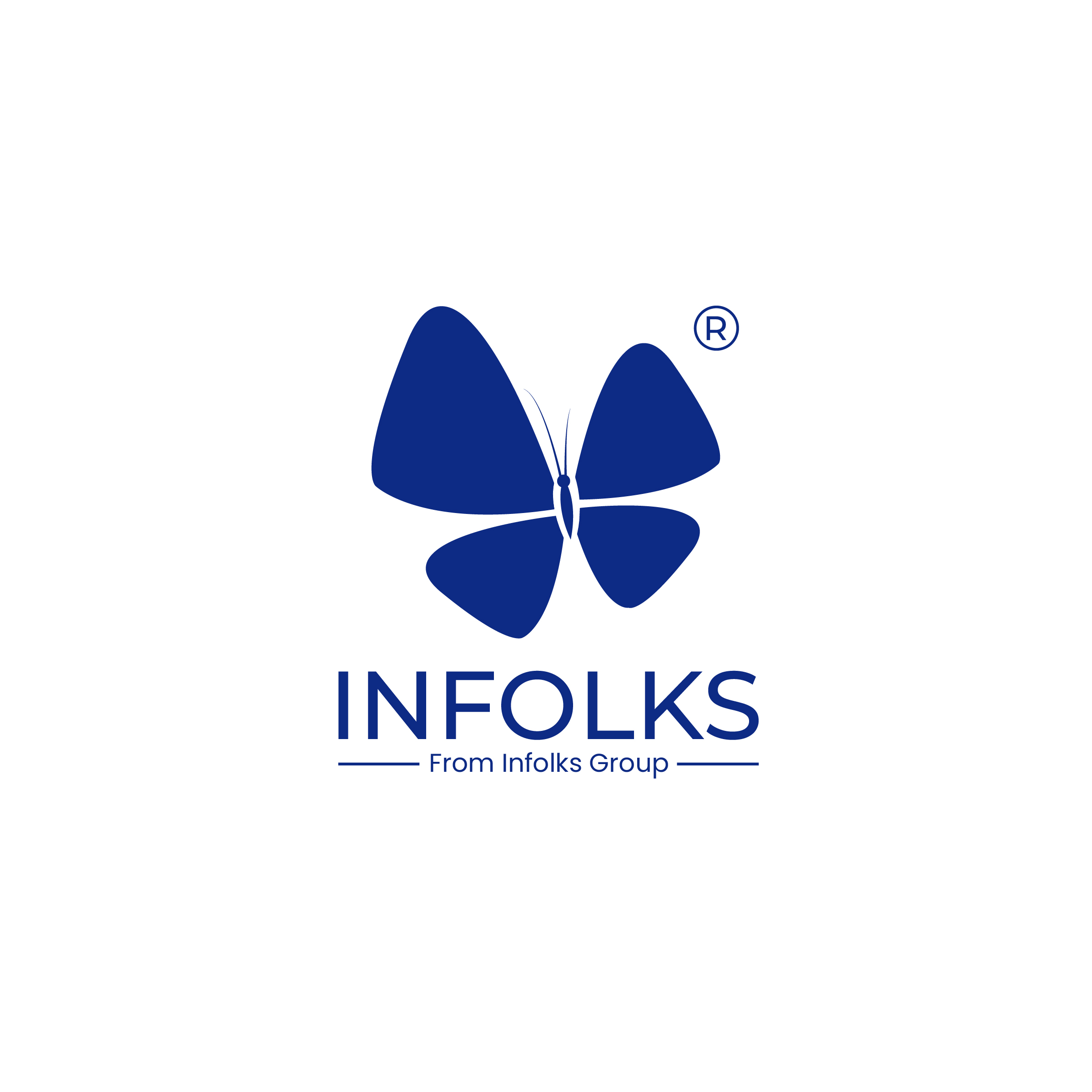
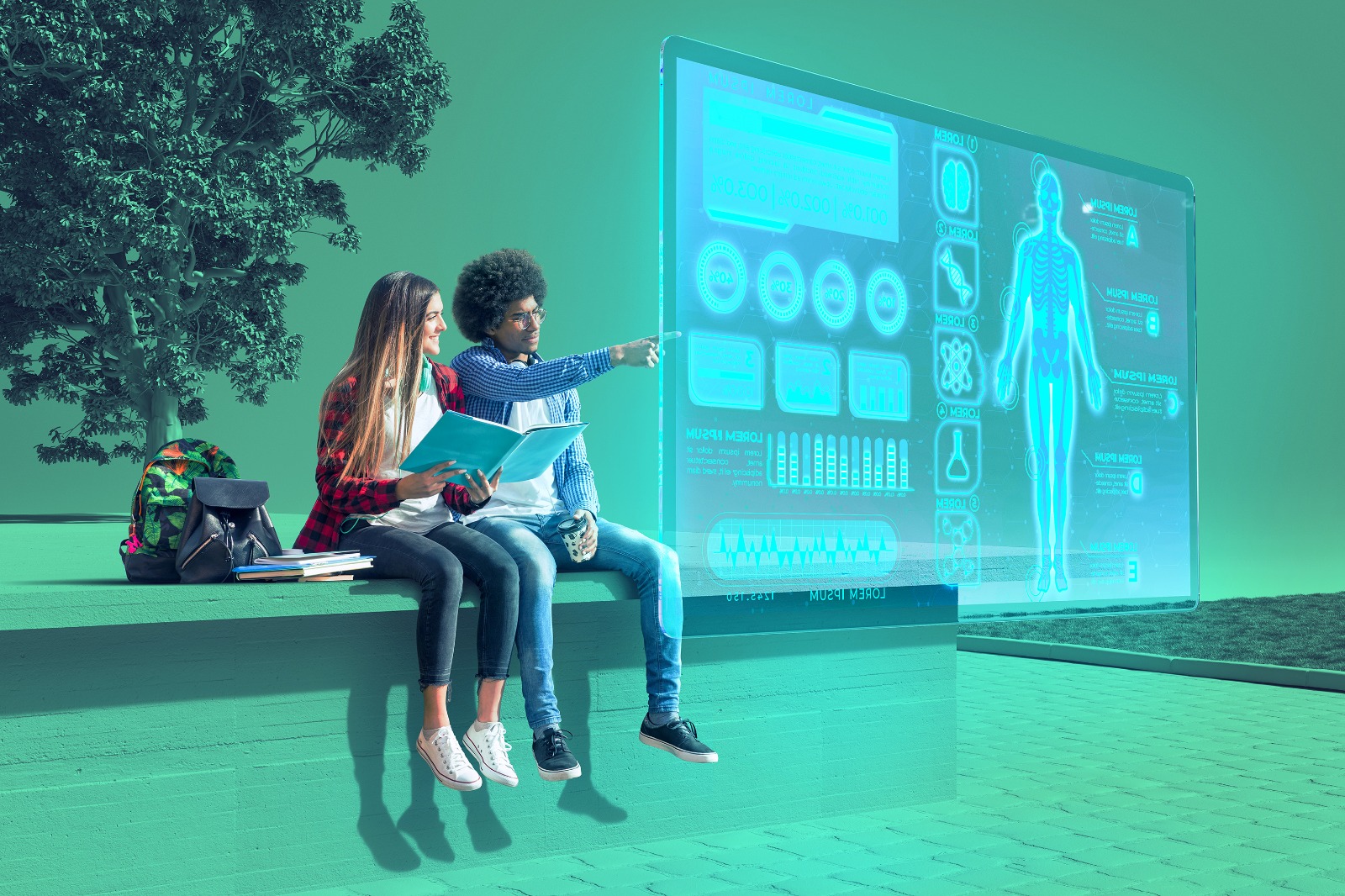
Artificial Intelligence (AI) has been making big strides lately, showing promise across various fields. However, one big challenge we face, especially in machine learning, is making AI understandable. New techniques like deep neural networks have made this harder compared to older AI methods like expert systems.
Explainable AI (XAI) is a field focused on making AI systems easier to understand and trust. In this article, we look at what's been done in XAI so far and what we still need to achieve. We define explainability in machine learning and talk about who needs to understand AI systems. We then categorize recent efforts to make different machine learning models understandable, especially deep learning methods, which are particularly tricky. We also explore how XAI intersects with other important issues like data fusion. Our aim is to promote responsible artificial intelligence, which means implementing AI in real-world settings with fairness, explainability, and accountability in mind. We hope this article helps newcomers understand XAI better and encourages experts from different fields to embrace AI without worrying too much about its complexity.
What is Explainable AI?
Explainable artificial intelligence (XAI) is all about making it easy for people to understand and trust what AI systems are doing. When we talk about explainable AI, we're basically describing an AI in a way that shows what it's supposed to do and points out any potential biases it might have. This is super important because it helps us know how accurate, fair, and transparent the AI is, especially when it's making decisions. The big deal with explainable AI is that it builds trust when we use AI in real-life situations. It also helps us be responsible when developing AI. As AI gets more advanced, it becomes tricky to figure out how algorithms come up with specific results. Sometimes, these processes turn into what we call "black box" models. This means it's hard for people, even the engineers and data scientists who made the algorithm, to understand or explain how it's making a decision.
Knowing how an AI system reaches a particular output has lots of benefits. It helps developers make sure the system is working correctly, follows rules, and lets people question or change the outcome. As companies work on making AI that's responsible on a large scale, including explainable AI is crucial. It helps keep things transparent and holds AI development accountable.
Why is Explainable AI?
Let’s check the need for explainable AI through some examples.
Medical Diagnosis with AI
In medical diagnostics, AI serves as a powerful tool for identifying potential health issues, such as cancer cells in medical imaging scans like computer tomography (CT) images. Take, for instance, the scenario where an AI system is deployed to assist radiologists in detecting cancerous cells. The AI analyzes a vast number of medical images and flags those that exhibit suspicious features indicative of cancerous growths. The challenge lies in ensuring that the AI's decision-making process is transparent and understandable to medical professionals. Radiologists and physicians need to comprehend why the AI highlights certain images for further examination while disregarding others. This transparency is crucial for instilling trust in AI systems among healthcare providers. Additionally, the AI must strike a delicate balance between sensitivity and specificity. It should accurately identify potential cancer cases to aid in early diagnosis and treatment while minimizing false positives to avoid overwhelming medical staff and reducing diagnostic accuracy.
Credit Scoring and Risk Prediction
AI plays a major role in assessing creditworthiness and predicting loan repayment risks based on various individual characteristics, including employment history, income level, and age. For instance, financial institutions utilize AI algorithms to evaluate loan applications and determine the likelihood of a borrower defaulting on payments. The use of AI in credit scoring raises concerns about potential biases and discrimination. Without transparent and explainable decision-making processes, AI algorithms may inadvertently perpetuate systemic biases or discriminate against certain demographic groups. It's essential for financial institutions to understand how AI models arrive at credit decisions to ensure fairness and compliance with regulatory requirements, such as anti-discrimination laws.
Predictive Policing and Law Enforcement
Predictive policing leverages AI-driven algorithms to analyze historical crime data and forecast future criminal activities. Law enforcement agencies use predictive analytics to identify crime hotspots, allocate resources effectively, and prevent criminal incidents. The deployment of predictive policing algorithms raises ethical and social implications, particularly regarding issues of privacy, civil liberties, and bias. Given the historical disparities in policing practices, there are concerns that AI models may exhibit implicit biases or reinforce existing inequities, such as racial profiling.
Therefore, it's necessary to ensure transparency and accountability in predictive policing algorithms. Law enforcement agencies must understand the underlying mechanisms of AI models and how they generate predictions. By promoting transparency and engaging in community dialogue, stakeholders can address concerns related to fairness, accountability, and the ethical use of AI in law enforcement.
Explainable AI’s Challenging Future
Understanding explainable AI is essential in navigating the complexities of modern technology. Imagine you have a super-smart computer that makes decisions, like predicting weather or suggesting movies. Explainable AI aims to make these decisions clear and understandable to humans. It's like trying to diagnose an indeterminate illness, it is tough to find a solution without clear details. Zachary Lipton, a machine learning professor, compares this challenge to diagnosing broad medical conditions that lack specificity.
AI used to handle simple data, but now it's dealing with complex stuff like language and images. Understanding these advanced systems is like solving a tough puzzle. Consider the shift from structured data, like numbers in spreadsheets, to unstructured data, such as natural language or raw images. It's much easier to question models based on clear data than those relying on unstructured information. As AI grows more sophisticated, explaining its decisions becomes increasingly complex. Still, explainable AI isn't going away anytime soon. As AI becomes more deeply integrated into our daily lives and faces increasing regulation, the need for accountability and transparency remains paramount. Whether AI is predicting the weather or diagnosing medical conditions, we need to trust that it's making sound decisions. But what's considered "explainable" today might change tomorrow as AI continues to evolve. As long as AI keeps improving, our understanding of it will need to keep pace.
Summing Up
In summary, while explainable AI faces significant challenges, it's crucial for building trust and confidence in technology. As the field advances, we'll continue to strive for clearer explanations and greater transparency in AI systems. After all, the better we understand AI, the better we can harness its potential for positive impact.
Subscribe to my newsletter
Read articles from Infolks directly inside your inbox. Subscribe to the newsletter, and don't miss out.
Written by
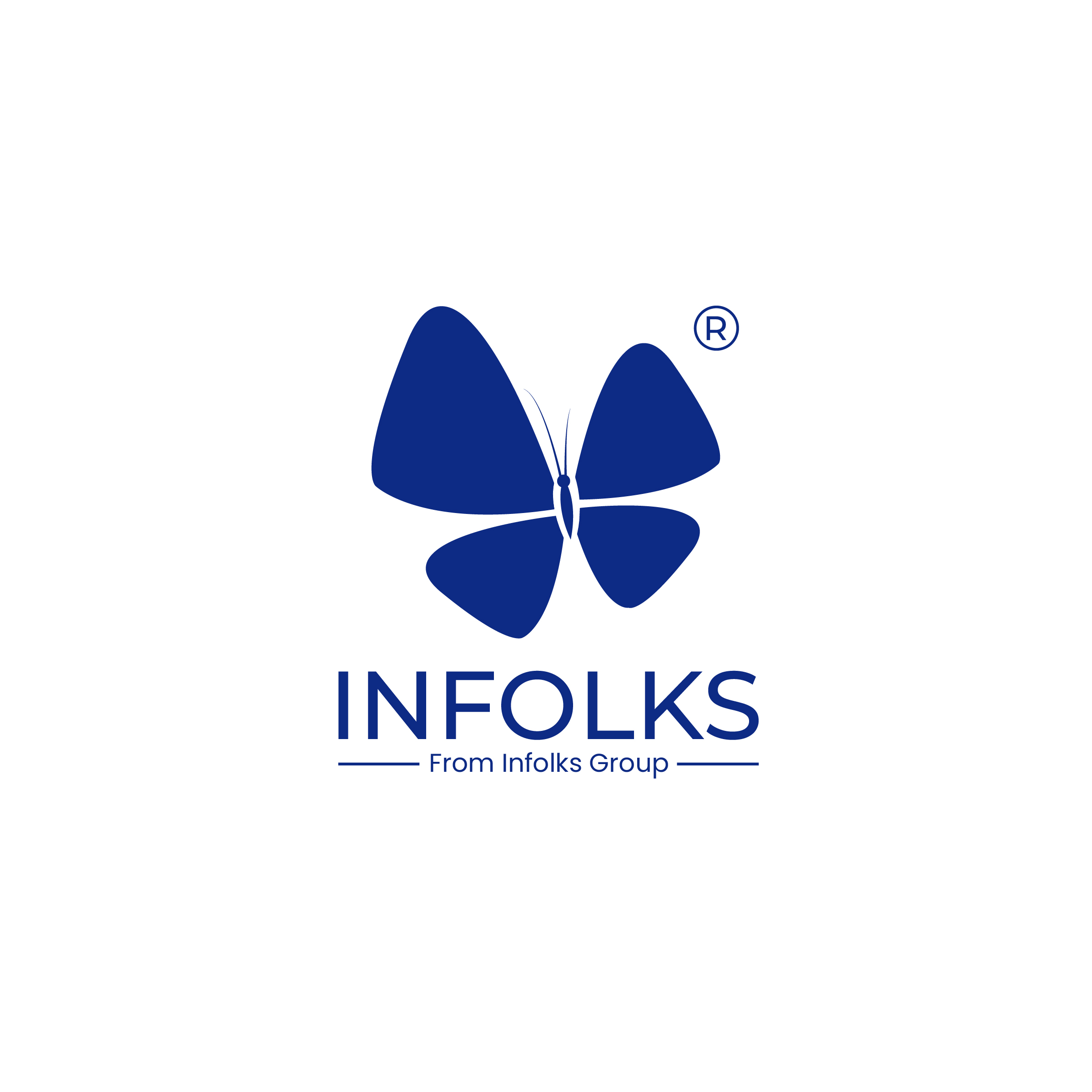
Infolks
Infolks
We are a leading data labeling service provider. Our super-powered workforce labels precise and quality training data sets for AI and ML modules. We’re here to bring someone’s AI dreams into reality.