How Accurate is AI Text Generator in Generating Human-like Text?
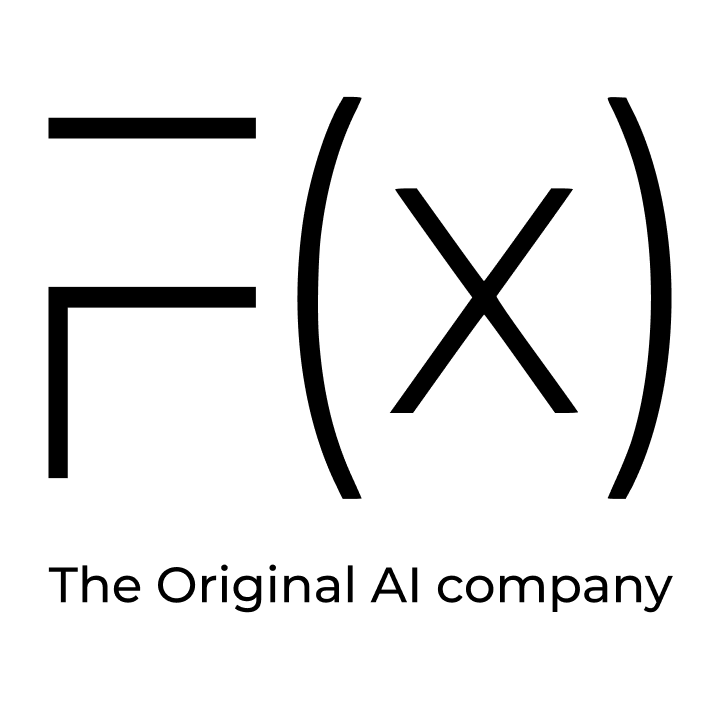
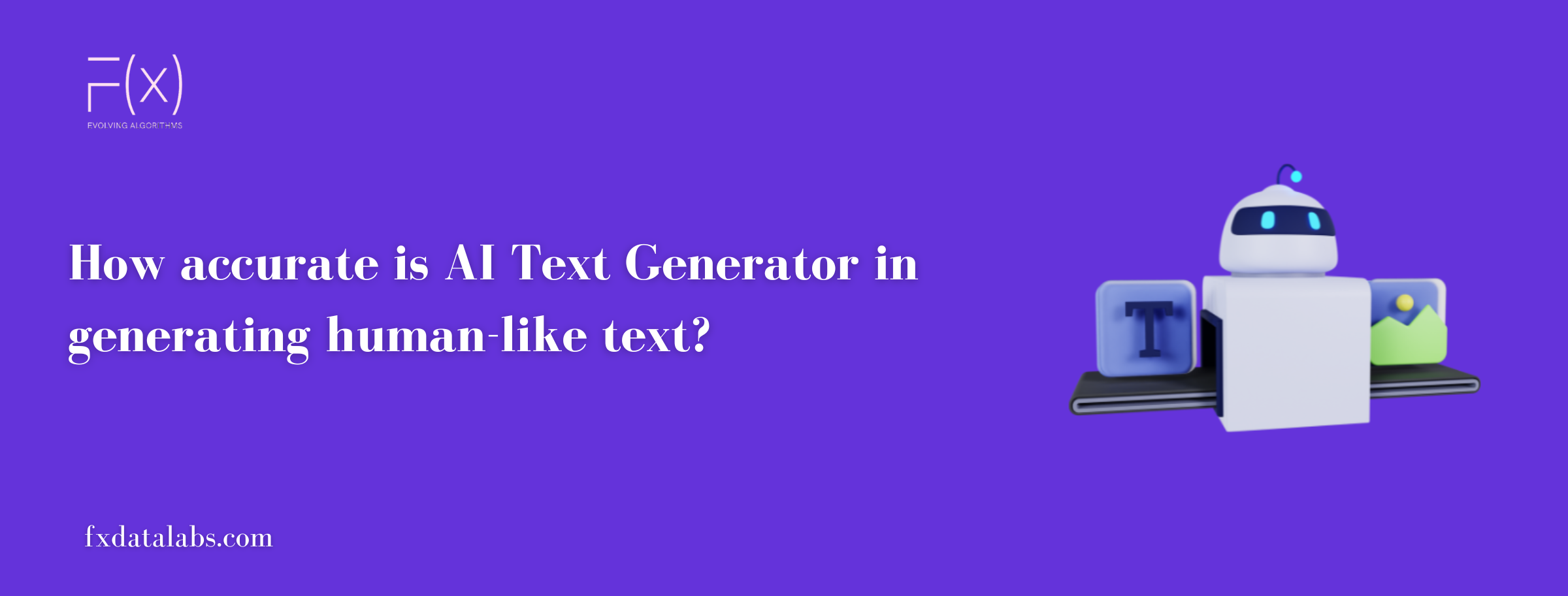
Artificial Intelligence (AI) has made significant strides in recent years, particularly in the realm of natural language processing. AI text generators, also known as language models, have gained widespread attention for their ability to generate human-like text autonomously.
But just how accurate are these AI-powered systems in mimicking human language? In this article, we explore the accuracy of AI text generators, the factors influencing their performance, and their implications for various applications.
Introduction
AI text generators leverage deep learning techniques to analyze and mimic patterns in human language. These models have demonstrated remarkable proficiency in generating coherent and contextually relevant text across a wide range of domains. However, their accuracy can vary depending on several factors, including the quality of training data, model architecture, and fine-tuning techniques.
Understanding AI Text Generators
Definition and Explanation
AI text generators are algorithms trained on vast amounts of text data to understand and replicate human language patterns. They employ techniques such as deep learning and recurrent neural networks to generate text that resembles human-written content.
How AI Text Generators Work ?
At their core, AI text generators utilize a process known as "unsupervised learning" to predict the next word in a sequence of text based on the words that precede it. This enables them to generate coherent and contextually relevant text autonomously.
Accuracy of AI Text Generators
Evaluation Metrics
Several metrics are used to assess the accuracy of AI text generators, including perplexity, BLEU score, and human evaluation. Perplexity measures the model's ability to predict the next word in a sequence, while the BLEU score evaluates the similarity between generated text and human-written references.
Human-Likeness of Generated Text
While AI text generators have made significant advancements, they may still fall short in producing text that is indistinguishable from human-written content. Factors such as grammar errors, lack of contextual understanding, and occasional nonsensical output can detract from the human-likeness of generated text.
Factors Affecting Accuracy Training Data Quality
The quality and diversity of training data play a crucial role in determining the accuracy of AI text generators. Models trained on well-curated and diverse datasets tend to perform better than those trained on limited or biased data sources.
Model Complexity
The complexity of the AI model architecture can also impact its accuracy. More sophisticated models, such as GPT-3, with larger parameter sizes and deeper layers, generally exhibit higher levels of accuracy compared to simpler models.
Fine-Tuning Techniques
Fine-tuning involves adjusting the parameters of pre-trained AI models to better align them with specific tasks or domains. Effective fine-tuning techniques can significantly improve the accuracy of AI text generators for specific applications.
Applications and Use Cases
Content Creation
AI text generators are widely used for content creation tasks such as generating articles, blog posts, and product descriptions. While they can produce text quickly and efficiently, human oversight is often required to ensure the quality and relevance of the generated content.
Language Translation
AI text generators equipped with machine translation capabilities can translate text from one language to another with varying degrees of accuracy. While they can facilitate communication across language barriers, they may struggle with nuances and idiomatic expressions.
Writing Assistance
AI text generators can serve as valuable writing assistants, providing real-time suggestions and feedback on grammar, spelling, and style. They can help writers overcome writer's block, improve productivity, and enhance the overall quality of their writing.
Limitations and Challenges
Contextual Understanding
One of the primary challenges faced by AI text generators is their limited ability to grasp context and infer meaning from text. As a result, they may produce text that is contextually inaccurate or lacks coherence, particularly in complex or ambiguous situations.
Domain-Specific Knowledge
AI text generators may struggle with generating text related to specialized domains or technical subjects that require domain-specific knowledge. They may produce generic or inaccurate content when faced with topics outside their training data.
Ethical Considerations
Ethical considerations surrounding AI text generators include concerns about plagiarism, misinformation, and bias. There are instances where generated content may inadvertently reproduce copyrighted material or propagate false information, raising ethical dilemmas for content creators and consumers alike.
Improvements and Innovations
Advancements in AI Technology
Ongoing research and development efforts are driving advancements in AI text generation technology. Innovations such as larger model architectures, improved training techniques, and better fine-tuning methods are enhancing the accuracy and reliability of AI text generators.
Hybrid Approaches
Some researchers are exploring hybrid approaches that combine the strengths of AI text generators with human oversight. By incorporating human feedback and intervention into the text generation process, these hybrid systems aim to mitigate the limitations of purely automated approaches.
User Feedback Mechanisms
AI text generators can benefit from user feedback mechanisms that enable continuous learning and improvement. By soliciting feedback from users and incorporating it into future iterations, these systems can adapt to evolving language patterns and user preferences.
Future Trends and Predictions
Enhanced Accuracy and Reliability
As AI text generation technology matures, we can expect to see further improvements in accuracy, reliability, and human-likeness of generated text. Future models may surpass current benchmarks and approach human-level performance in specific tasks.
Integration with Other Tools
AI text generators are likely to become more integrated with other content creation tools and platforms, offering seamless workflows and enhanced user experiences. Integration with writing software, content management systems, and collaboration tools can streamline the content creation process.
Adoption Across Industries
The adoption of AI text generators is expected to continue across a wide range of industries and applications. From journalism and marketing to customer service and education, these tools have the potential to transform how content is created, consumed, and shared.
Conclusion
AI text generators hold immense promise for streamlining content creation workflows, enhancing productivity, and unlocking new creative possibilities. While their accuracy and capabilities continue to improve, it's essential to approach them with a critical eye, understanding their limitations and ethical implications.
By leveraging the strengths of AI text generators while mitigating their challenges, content creators can harness the full potential of these tools to create engaging, high-quality content.
For more insights into AI|ML and Data Science Development, please write to us at: contact@htree.plus| F(x) Data Labs Pvt. Ltd.
#AI #ContentGeneration #LanguageAI #Innovation ๐ค๐
Subscribe to my newsletter
Read articles from FxisAi - Yagnesh Pandya directly inside your inbox. Subscribe to the newsletter, and don't miss out.
Written by
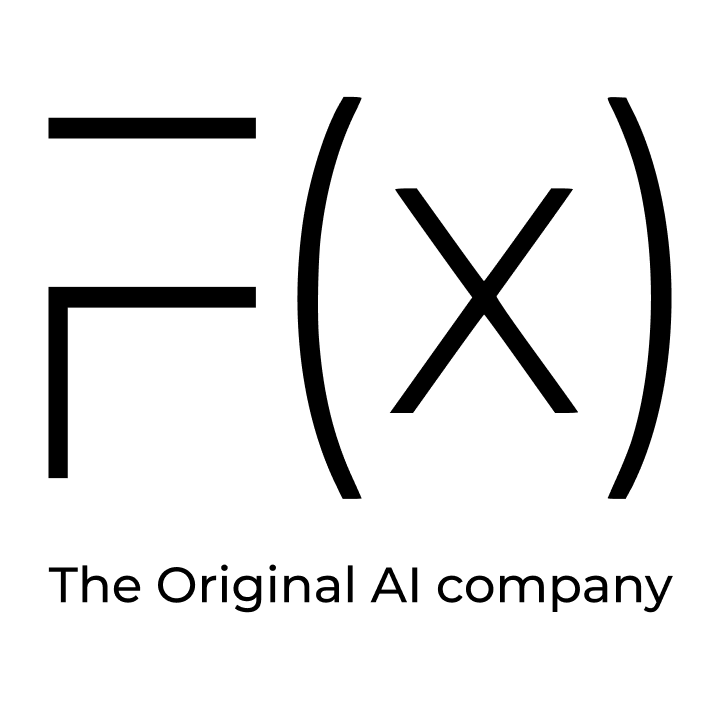
FxisAi - Yagnesh Pandya
FxisAi - Yagnesh Pandya
Business Developer with IT - AI & Data Science