The Essential Guide to Audio Data Collection for Machine Learning
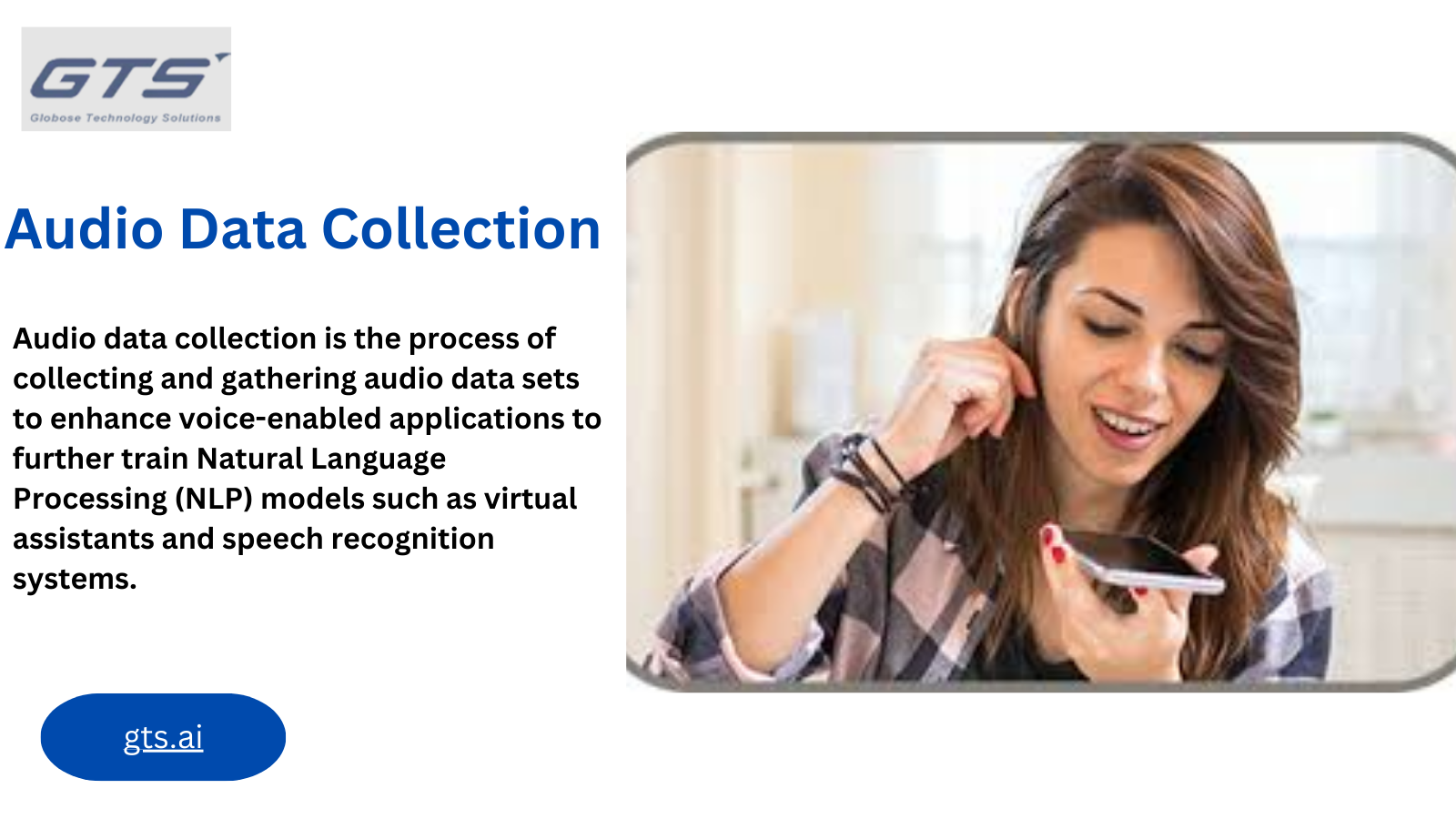
In the realm of machine learning, the importance of high-quality data cannot be overstated. When it comes to audio-based applications, such as speech recognition, voice authentication, and sound classification, the collection of diverse and well-annotated audio datasets is crucial for training accurate and robust models.
What is Audio Data Collection?
Audio data collection involves the process of gathering and organising audio recordings that will be used to train machine learning models. This process typically includes capturing audio samples using microphones or recording devices, storing the recordings in a suitable format, and annotating the data with relevant metadata.
Why is Audio Data Collection Important?
Accurate and comprehensive audio datasets are essential for developing machine learning models that can perform tasks such as speech recognition, speaker identification, and environmental sound classification. Without high-quality data, models may struggle to generalise to new, unseen data, leading to poor performance in real-world applications.
Best Practices for Audio Data Collection
- Diverse Dataset: Collect audio samples from a wide range of sources to ensure that your dataset is representative of real-world conditions. This can include different speakers, environments, and recording devices.
- Annotation: Annotate your audio data with relevant metadata, such as speaker identities, transcription of speech, or labels for different sounds. This information is essential for training supervised machine learning models.
- Quality Control: Ensure that your audio recordings are of high quality, with minimal background noise or interference. Use high-quality microphones and recording equipment to capture clear and accurate audio samples.
Data Privacy: Respect the privacy and consent of individuals whose voices are being recorded. Obtain permission before recording and use anonymization techniques to protect sensitive information.
Data Augmentation: To enhance the diversity of your dataset, consider augmenting your audio data by adding background noise, altering pitch or speed, or mixing audio samples from different sources.
Challenges in Audio Data Collection
Collecting and annotating audio data can be a challenging and time-consuming process. Some common challenges include:
Noise and Interference: Background noise can degrade the quality of audio recordings, making it harder to extract useful information.
Speaker Variability: Different speakers may have varying accents, speech patterns, and vocal characteristics, requiring a diverse dataset to capture this variability.
Data Annotation: Annotating audio data with accurate labels and metadata can be labour-intensive, especially for large datasets.
Conclusion
Audio data collection is a critical step in the development of machine learning models for audio-based applications. By following best practices and addressing common challenges, researchers and developers can create high-quality datasets that enable the creation of accurate and reliable machine learning models for a variety of audio-related tasks.
Subscribe to my newsletter
Read articles from GLOBOSE TECHNOLOGY SOLUTIONS PRIVATE LIMITED directly inside your inbox. Subscribe to the newsletter, and don't miss out.
Written by
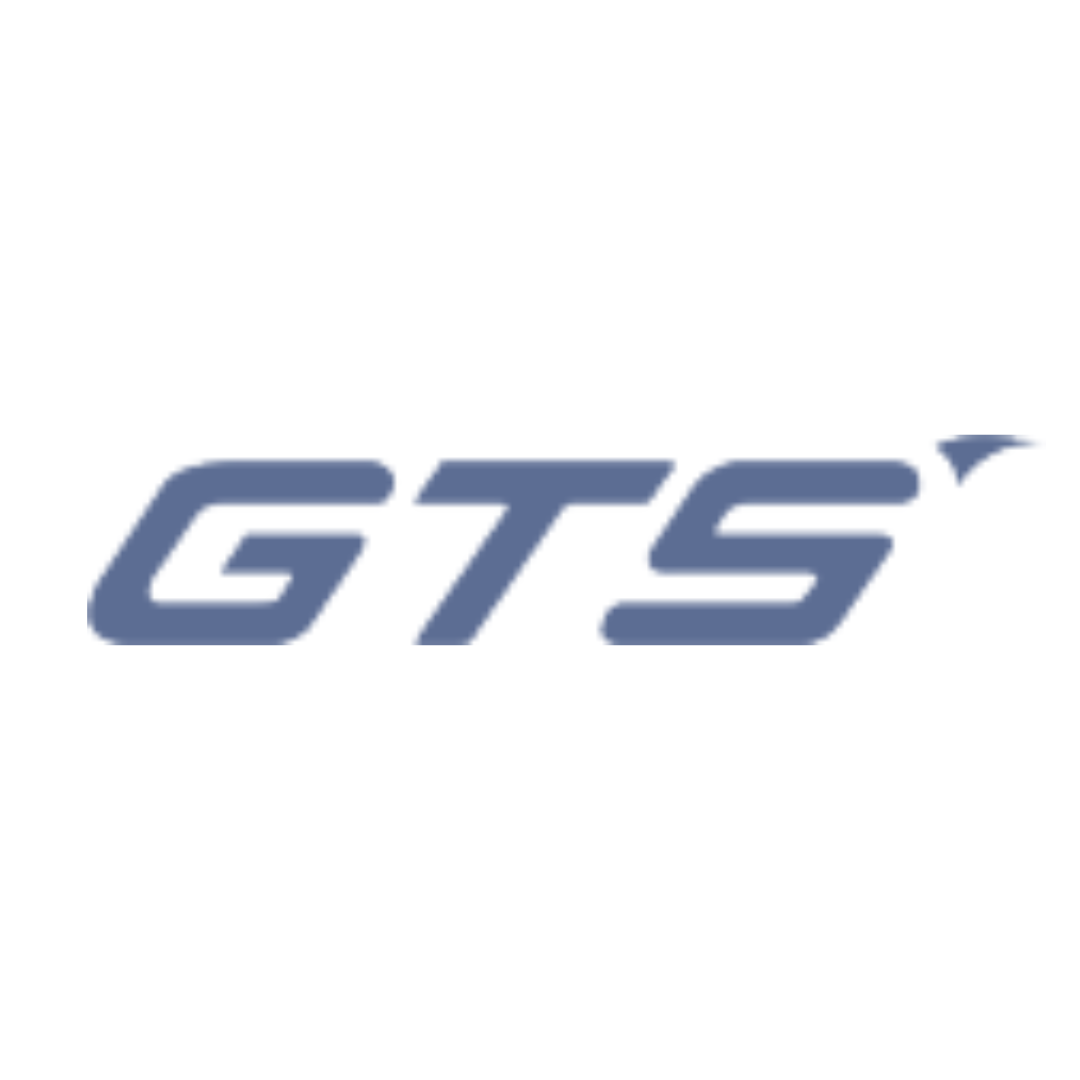
GLOBOSE TECHNOLOGY SOLUTIONS PRIVATE LIMITED
GLOBOSE TECHNOLOGY SOLUTIONS PRIVATE LIMITED
Globose Technology Solutions Pvt Ltd (GTS) is an AI data collection Company that provides different Datasets like image datasets, video datasets, text datasets, speech datasets, etc. to train your machine learning model.