LLMs - Still far From Human Intelligence

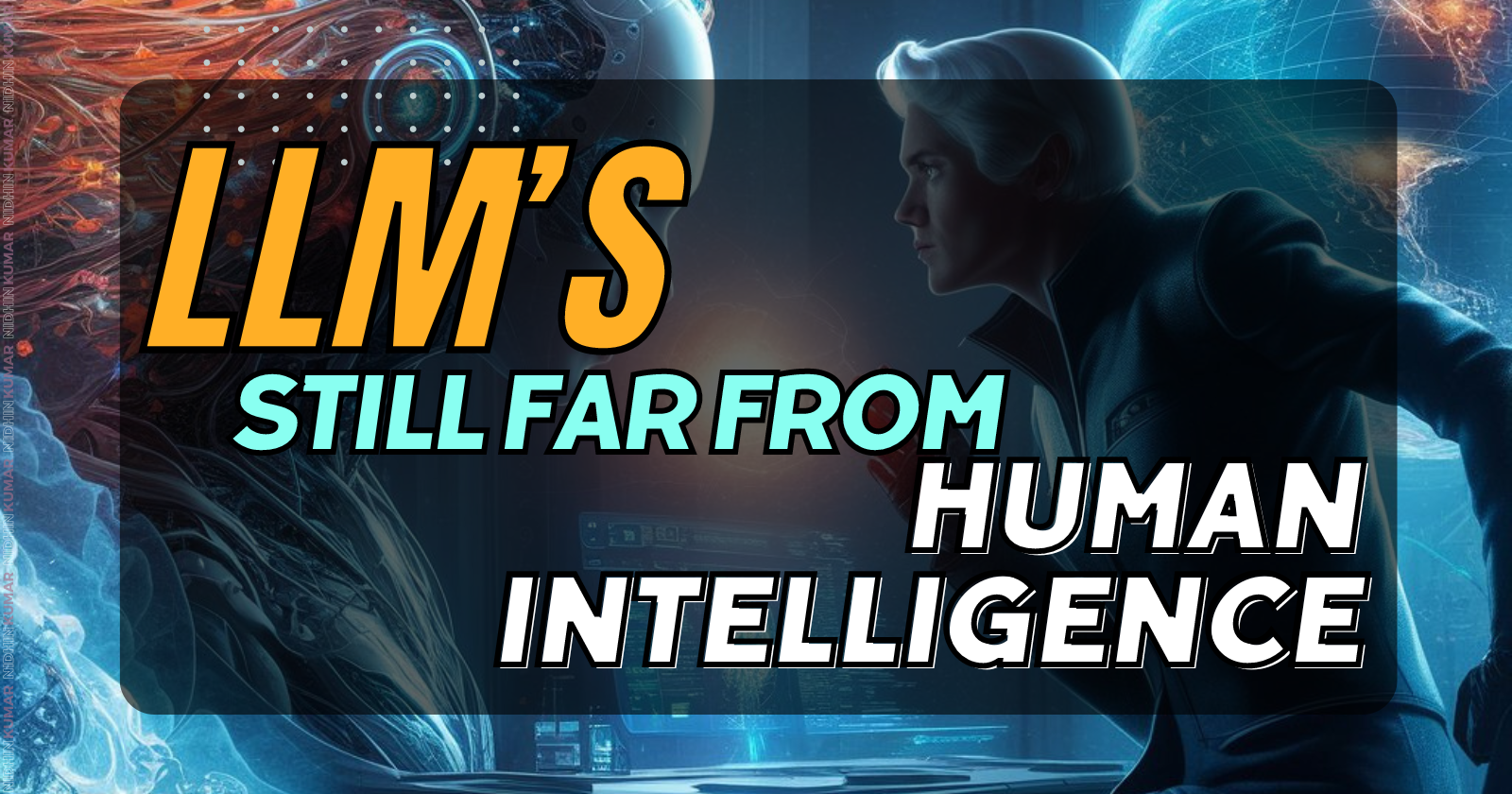
We surpass everyday with a headline about the concept, which envisions computer systems outperforming humans at various cognitive tasks.
In the last month alone, a trio of tech luminaries have added fresh proclaimations.
Nvidia's CEO Jensen Huang suggested that AGI (Artificial General Intelligence) would arrive withing 5 years.
Ben "Father of AGI" Goertzel forecasted a mere of 3 years. And Musk made a bold prediction of by the end of 2025
Still not everyone is bullish. One notable sceptic is Yann LeCun, Meta's chief AI scentist and the winner of Prestigious Turing Award. Often referred as one of three "Godfathers of AGI".
LeCun goes as far as to argue that there is "No such thing as AGI" because "Human intelligence nowhere near to general"
LeCun pointed out to a quartet of cognitive challenges
Reasoning
Planning
Persistent memory
Understanding the physical world
Those are the four essential characteristics of human-intelligence that current AI systems can't do. Without these capabilities, AI applications remain limited and errorprone.
Autonomous vehicles are still aren't safe for public roads. Domestic robots still struggle with basic household chores and even our smart assistants can complete the basic tasks.
These shortcomings are prominent in Large Language Models (LLM) from LeCun's PoV
We're easily fooled into thinking that they are intelligent because of their fluency with language, but really their understanding of reality is very superficial
They’re useful, and there’s no question about that. But on the path towards human-level intelligence, an LLM is basically an off-ramp, a distraction, a dead end.
Why LLM's aren't as smart as they seem
Meta’s LLaMA, OpenAI’s GPT-3, and Google’s Bard are examples of AI models trained on vast amounts of data. According to LeCun, it would require approximately 100,000 years for a human to read all the text processed by a leading LLM. However, this isn't our primary mode of learning.
Our main source of information absorption is through our interactions with the environment. LeCun suggests that an average four-year-old has been exposed to 50 times more data than the largest LLMs in the world.
"Most of human knowledge isn't solely language-based, so these systems can't attain human-level intelligence unless the fundamental architecture is altered," LeCun remarked.
Naturally, the 63-year-old proposes an alternative architecture, which he terms "objective-driven AI."
Objective-driven AI
Objective-driven AI systems are like robots with a job to do. Instead of just reading lots of words, they learn by seeing and feeling things in the world, like watching videos.
They make a picture in their minds of how things work and what happens when they do something. So if they move a chair, they know what might happen if they move it left or right. This helps them plan what to do next.
LeCun thinks these AI systems will become really smart someday, even smarter than people, but it'll take some time. It won't happen right away.
Subscribe to my newsletter
Read articles from nidhinkumar directly inside your inbox. Subscribe to the newsletter, and don't miss out.
Written by
