Search Paradigms: Exploring Dense, Sparse, and Hybrid Search Techniques

Table of contents
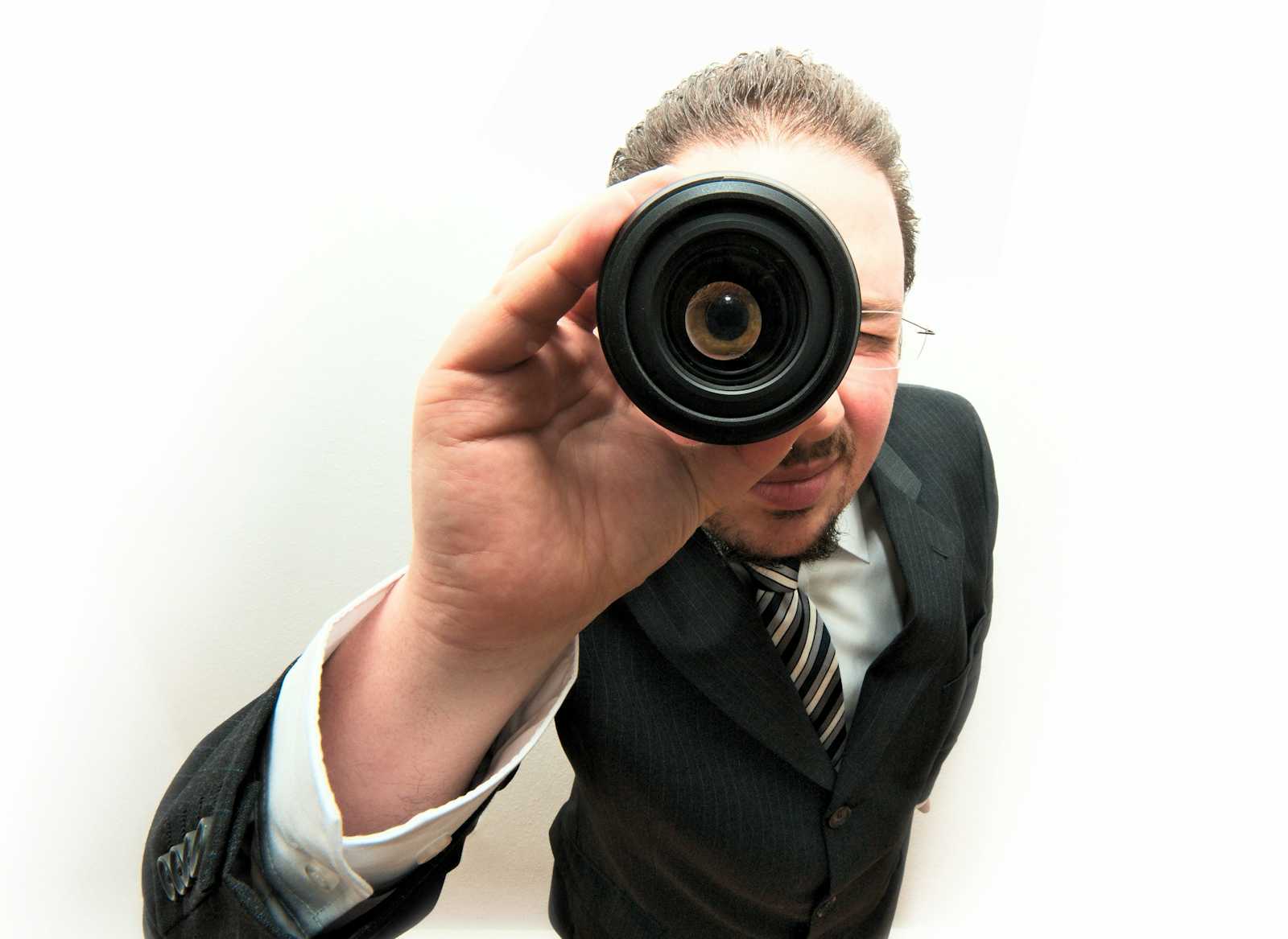
Introduction:
In the realm of information retrieval, search methodologies vary widely, each offering unique advantages and limitations. This technical blog delves into three prominent search paradigms: Dense Search, Sparse Search, and Hybrid Search. From leveraging vector embeddings for semantic similarity to employing keyword-based approaches, we explore the nuances of each technique and discuss how Hybrid Search combines the strengths of both dense and sparse methodologies. Through this exploration, we aim to provide insights into optimizing search strategies for diverse applications.
Dense Search:
Dense Search harnesses vector embeddings to represent data, enabling semantic similarity-based retrieval. While this approach offers powerful capabilities, it is not without limitations. For instance, neural networks powering dense search are only as effective as the data they are trained on. Consequently, queries falling outside the scope of the training data may not yield accurate results.
Sparse Search:
Sparse Search addresses the shortcomings of Dense Search by employing keyword-based or bag-of-words approaches. In this methodology, a dictionary of all possible words is created, and the occurrence count of each word is maintained. However, the presence of numerous zero counts in the dictionary poses a significant disadvantage, impacting the search efficiency and relevance.
Hybrid Search:
Hybrid Search emerges as a promising solution by combining the strengths of Dense and Sparse Search techniques. By integrating both semantic similarity-based dense search and keyword-based sparse search, Hybrid Search utilizes a scoring system to assess the relevance of search results comprehensively. This approach enhances search accuracy and robustness by leveraging the complementary nature of dense and sparse methodologies.
Conclusion:
In the dynamic landscape of information retrieval, selecting the appropriate search paradigm is crucial for achieving optimal results. Dense Search offers semantic similarity-based retrieval but may falter with out-of-scope queries. Sparse Search, on the other hand, relies on keyword-based approaches but suffers from sparse data representation. Hybrid Search bridges the gap by seamlessly integrating both dense and sparse methodologies, enabling comprehensive and accurate information retrieval. By understanding the nuances of each search paradigm, practitioners can tailor their search strategies to suit specific use cases and maximize the effectiveness of their search systems.
Subscribe to my newsletter
Read articles from Saurabh Naik directly inside your inbox. Subscribe to the newsletter, and don't miss out.
Written by

Saurabh Naik
Saurabh Naik
๐ Passionate Data Enthusiast and Problem Solver ๐ค ๐ Education: Bachelor's in Engineering (Information Technology), Vidyalankar Institute of Technology, Mumbai (2021) ๐จโ๐ป Professional Experience: Over 2 years in startups and MNCs, honing skills in Data Science, Data Engineering, and problem-solving. Worked with cutting-edge technologies and libraries: Keras, PyTorch, sci-kit learn, DVC, MLflow, OpenAI, Hugging Face, Tensorflow. Proficient in SQL and NoSQL databases: MySQL, Postgres, Cassandra. ๐ Skills Highlights: Data Science: Statistics, Machine Learning, Deep Learning, NLP, Generative AI, Data Analysis, MLOps. Tools & Technologies: Python (modular coding), Git & GitHub, Data Pipelining & Analysis, AWS (Lambda, SQS, Sagemaker, CodePipeline, EC2, ECR, API Gateway), Apache Airflow. Flask, Django and streamlit web frameworks for python. Soft Skills: Critical Thinking, Analytical Problem-solving, Communication, English Proficiency. ๐ก Initiatives: Passionate about community engagement; sharing knowledge through accessible technical blogs and linkedin posts. Completed Data Scientist internships at WebEmps and iNeuron Intelligence Pvt Ltd and Ungray Pvt Ltd. successfully. ๐ Next Chapter: Pursuing a career in Data Science, with a keen interest in broadening horizons through international opportunities. Currently relocating to Australia, eligible for relevant work visas & residence, working with a licensed immigration adviser and actively exploring new opportunities & interviews. ๐ Let's Connect! Open to collaborations, discussions, and the exciting challenges that data-driven opportunities bring. Reach out for a conversation on Data Science, technology, or potential collaborations! Email: naiksaurabhd@gmail.com