Understanding AI and GDPR: A Guide to Data Protection Laws
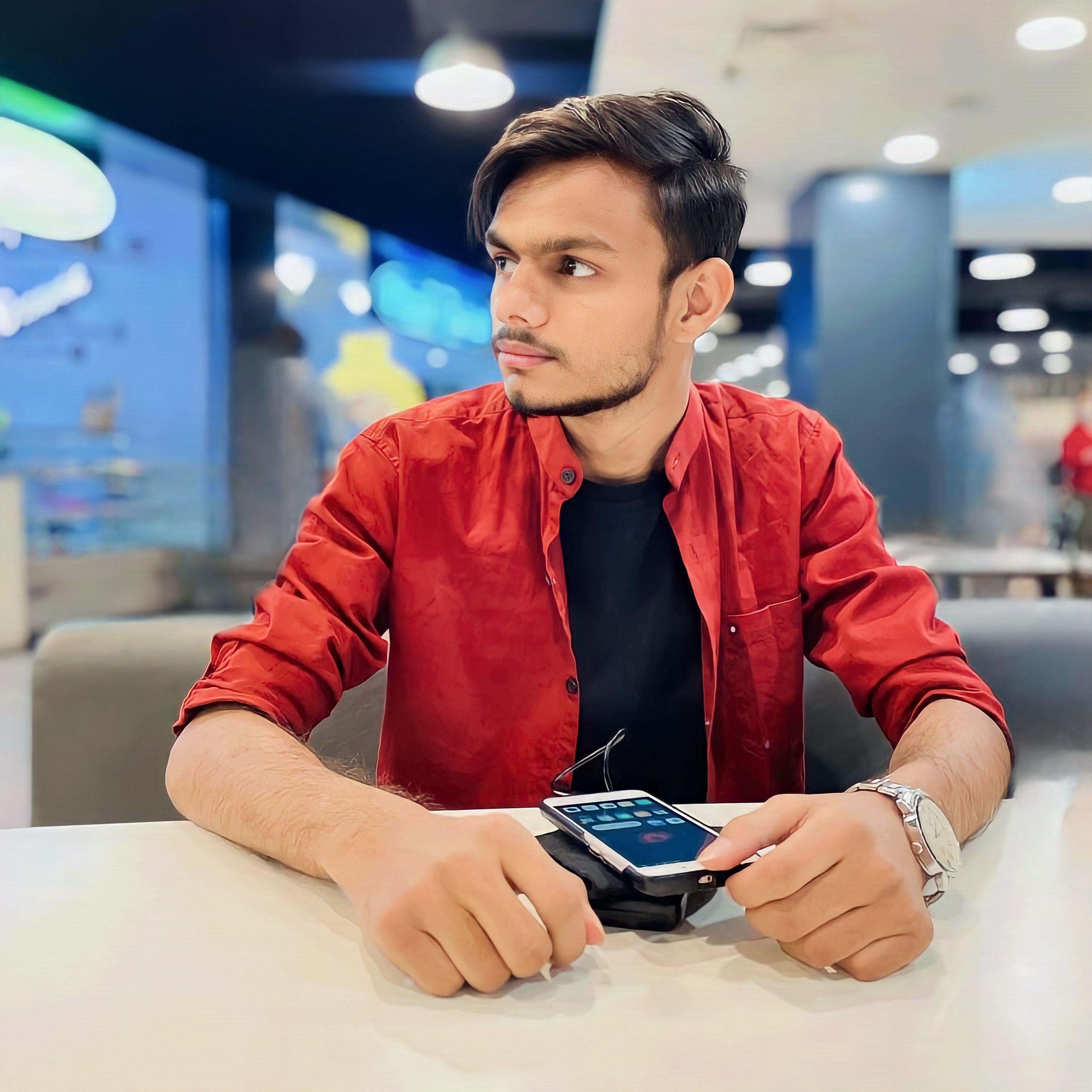
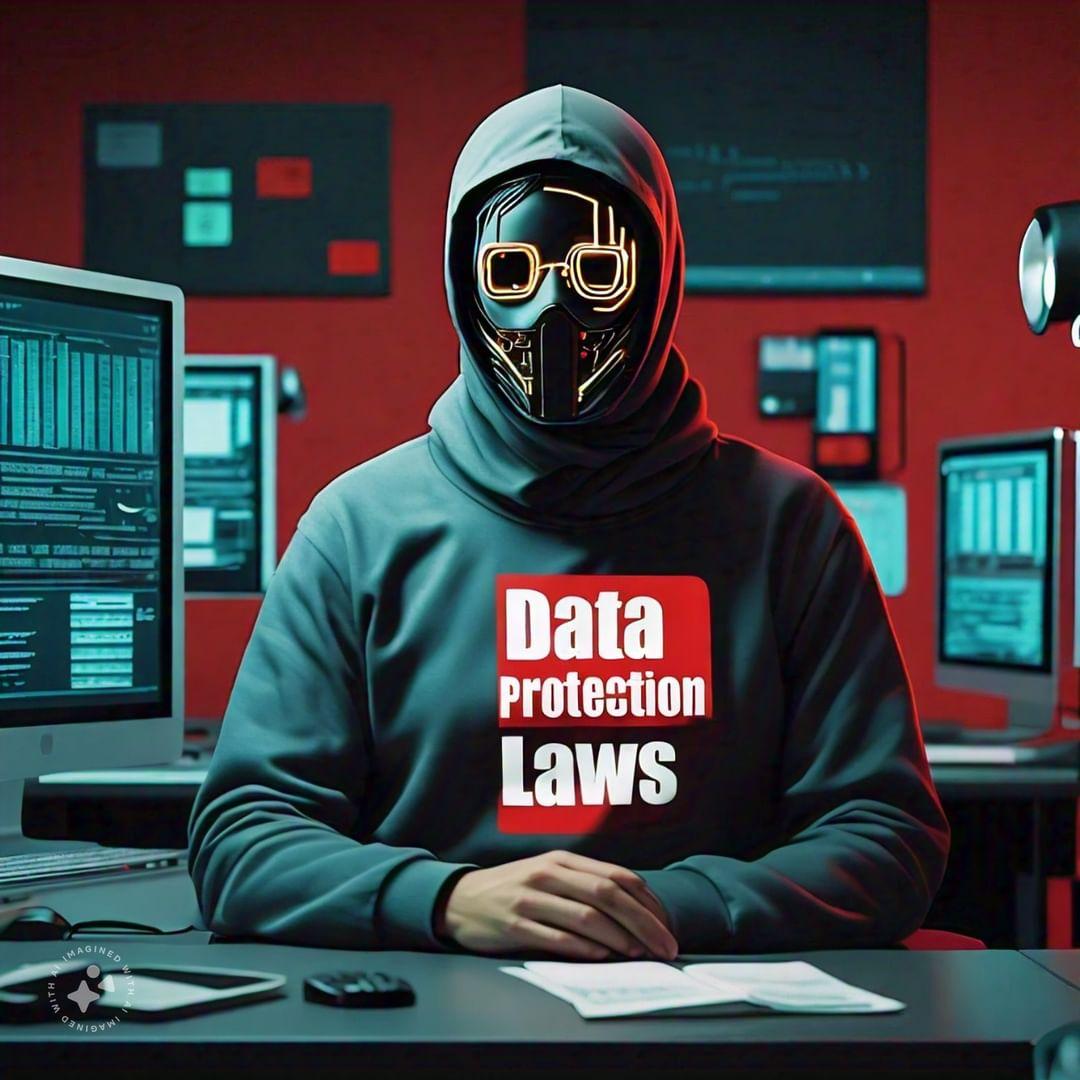
In today’s fast-paced digital landscape, artificial intelligence (AI) stands as a revolutionary force, transforming industries and reshaping the way businesses operate. Simultaneously, data privacy concerns have taken centre stage, with regulations like the General Data Protection Regulation (GDPR) in the European Union setting the gold standard for safeguarding individuals’ data rights. In this comprehensive article, we’ll look deeper into the intersection of AI and GDPR, exploring the legal landscape and strategies for ensuring compliance, all while emphasizing the paramount importance of responsible innovation.
The AI Revolution: A Data-Driven Paradigm
At the heart of AI’s power lies its remarkable ability to analyze vast datasets and derive invaluable insights. This data-driven paradigm, however, raises fundamental questions about privacy, consent, and ethical considerations. As AI systems process personal information with increasing sophistication, organizations are tasked with navigating the complex web of regulations to uphold individuals’ rights and expectations regarding data privacy. If you look at a very recent incident where, OpenAI’s CEO “Sam Altman” accused Google for using ChatGPT’s responses to train Google’s BardAI, which even tough does not break any laws but still highlights a underlying unethical use case of AI revolution for commercial purposes.
Understanding GDPR and Its Relevance to AI
The GDPR, implemented in May 2018, represents a monumental shift in data protection. Its primary aim is to harmonize data protection laws across the EU, providing individuals with greater control over their personal data. GDPR’s relevance to AI cannot be overstated; any AI system that processes personal data, from customer Chatbot’s to recommendation algorithms, must align with its provisions.
Challenges in AI-GDPR Compliance
Navigating the intricate terrain where AI and GDPR converge presents numerous challenges that organizations must address proactively:
- Data Minimization and Purpose Limitation: GDPR necessitates that AI models process only the minimum amount of data required for their intended purpose. This principle aligns seamlessly with the GDPR’s data minimization and purpose limitation requirements. AI models are trained on large datasets, but the data the organization has should be complete, accurate, and relevant for the intended purpose. Data minimization and purpose limitation are two key principles of the GDPR. Data minimization requires organizations to only collect and process the minimum amount of personal data necessary for their intended purpose. Purpose limitation requires organizations to only use personal data for the purpose for which it was collected, or for a purpose that is compatible with the original purpose. On the other hand AI models are often trained on large datasets of personal data. This is because the more data an AI model is trained on, the more accurate it is likely to be. However, it is important to ensure that the data used to train an AI model is complete, accurate, and relevant for the intended purpose. This will help to ensure that the AI model is fair and does not make discriminatory decisions.
For example, if an AI model is being used to predict the risk of a customer defaulting on a loan, the data used to train the model should include information about the customer's credit history, income, and employment status. However, the data should not include information such as the customer's race, religion, or gender. This is because this information is not relevant to the purpose of the AI model.
By following these tips, organizations can help to ensure that their AI models are consistent with the GDPR's data minimization and purpose limitation requirements.
- Transparency and Explainability: GDPR’s “right to explanation” places the restriction on organizations to ensure that AI models are transparent and provide understandable results. The integration of interpretable AI algorithms is pivotal to compliance, what this means is that AI models should be able to explain how they reached their decisions. Interpretable AI algorithms are algorithms that can explain their decisions in a way that is understandable to humans. These algorithms can be used to make AI models more transparent and compliant with the GDPR.
We can consider a hypothetical company could use an interpretable AI algorithm to explain why its AI model predicted that a customer is likely to default on a loan. The algorithm could explain the factors that were considered in making the prediction, such as the customer's credit history, income, and employment status.
Consent and User Rights: Organizations must obtain explicit consent for data processing and uphold user rights, such as the right to erasure (commonly known as the right to be forgotten) and the right to access their data. This basically allows the user to control how their personal data is used and processed by organizations. The GDPR gives individuals a number of rights over their personal data, including the right to, CONSENT, ERASURE and ACCESS.
Consent: Individuals have the right to give or withdraw consent to the processing of their personal data. Organizations must obtain explicit consent from individuals before collecting or processing their personal data.
Erasure: Individuals have the right to have their personal data erased. This is also known as the "right to be forgotten." Organizations must erase an individual's personal data upon request, unless there is a legitimate reason to retain it.
Access: Individuals have the right to access their personal data and to obtain a copy of it. Organizations must provide individuals with a copy of their personal data upon request.
Data Security and Accountability: GDPR mandates that organizations implement robust data security measures, which are indispensable for AI systems that handle sensitive personal information. AI systems that handle sensitive personal information should be designed and implemented with security in mind. The GDPR mandates that organizations implement robust data security measures to protect personal data from unauthorized access, use, disclosure, disruption, modification, or destruction. This is especially important for AI systems, which often handle large amounts of sensitive personal data.
Here are some tips for designing and implementing secure AI systems:
Use encryption to protect personal data at rest and in transit. Encryption is a process of scrambling data so that it can only be read by authorized individuals. This can help to protect personal data from unauthorized access, even if the data is stolen or lost.
Implement access controls to restrict who can access personal data. Access controls can be used to ensure that only authorized individuals can access personal data. This may include using passwords, two-factor authentication, and access control lists.
Monitor AI systems for security threats. It is important to monitor AI systems for security threats on an ongoing basis. This may include using security information and event management (SIEM) tools to monitor system logs for suspicious activity.
Have a plan for responding to security incidents. In the event of a security incident, it is important to have a plan for responding to the incident. This plan should include steps for containing the incident, investigating the incident, and notifying affected individuals.
Strategies for Compliance and Responsible Innovation
The pursuit of compliance in the intricate AI-GDPR landscape necessitates a proactive and multifaceted approach:
Comprehensive Data Mapping: Gain a comprehensive understanding of the data collected and processed by AI systems. Maintain a detailed inventory of data flows to ensure transparency and compliance.
Privacy by Design: Incorporate data protection into the very fabric of AI system development. Consider privacy implications at every stage of the AI lifecycle, from design and development to deployment.
Regular Audits and Continuous Monitoring: Continuously monitor AI systems for compliance and conduct regular audits to identify and rectify issues promptly. A proactive approach enhances security and compliance.
Legal Expertise and Collaboration: Seek legal counsel experienced in data protection and AI to ensure adherence to GDPR and other relevant regulations. Collaborate with experts to navigate the complex legal landscape.
Case Study: AI and GDPR: Navigating the Legal Landscape for Data Protection
Overview
This case study examines the legal landscape for data protection in the context of artificial intelligence (AI). It will explore the key challenges and considerations that organizations must be aware of when developing and deploying AI systems that process personal data.
Background
AI is rapidly transforming many industries and sectors, and its use is only expected to grow in the coming years. AI systems are already being used for a wide range of purposes, including fraud detection, medical diagnosis, and personalized marketing. However, the use of AI also raises a number of data protection concerns. AI systems often rely on large datasets of personal data to train and operate. This data may be collected from a variety of sources, such as social media, customer surveys, and medical records. The General Data Protection Regulation (GDPR) is the primary piece of legislation governing data protection in the European Union (EU). The GDPR imposes strict requirements on organizations that collect and process personal data. These requirements include:
Obtaining consent from individuals before collecting or processing their personal data
Providing individuals with access to their personal data and the right to have it erased
Taking appropriate security measures to protect personal data from unauthorized access, loss, or destruction
Challenges and Considerations
Organizations that are developing or deploying AI systems that process personal data must carefully consider how to comply with the GDPR. Some of the key challenges and considerations include:
Transparency: Organizations must be transparent with individuals about how their personal data is being used in AI systems. This includes providing information about the purpose of the processing, the types of data being used, and the potential consequences of the processing.
Explainability : AI systems can be complex and difficult to understand. This can make it difficult for individuals to understand how their personal data is being used and how the AI system is making decisions. Organizations must take steps to make their AI systems more explainable, such as providing individuals with feedback on the decisions that are made and the factors that were considered.
Fairness: AI systems can be biased, which can lead to unfair and discriminatory outcomes. Organizations must take steps to ensure that their AI systems are fair and unbiased. This includes using high-quality data to train the AI system and monitoring the system's performance for bias.
So consider A company that develops AI-powered facial recognition software is considering deploying its software in a new market. The company's software is used to identify individuals in CCTV footage and other video surveillance data. The company must carefully consider how to comply with the GDPR in this new market. One of the key challenges is transparency. The company must be transparent with individuals about how their personal data is being used in its facial recognition software. This includes providing information about the purpose of the processing, the types of data being used, and the potential consequences of the processing. The company must also make its facial recognition software more explainable. This means providing individuals with feedback on the decisions that are made by the software and the factors that were considered. For example, if the software is used to identify an individual as a potential suspect in a crime, the software should be able to explain to the individual how it made that decision.
Finally, the company must ensure that its facial recognition software is fair and unbiased. This means using high-quality data to train the software and monitoring the software's performance for bias. For example, the company should make sure that the software is equally accurate at identifying individuals of all races and genders.
The use of AI raises a number of data protection challenges. Organizations that are developing or deploying AI systems that process personal data must carefully consider how to comply with the GDPR. Key challenges and considerations include transparency, explainability, and fairness.
Recommendations
Organizations can take a number of steps to comply with the GDPR in the context of AI:
Conduct a data protection impact assessment (DPIA): A DPIA is a process for assessing the risks to individuals' rights and freedoms posed by a proposed data processing activity. A DPIA can help organizations to identify and mitigate data protection risks associated with AI systems.
Implement appropriate technical and organizational measures: Organizations must take appropriate technical and organizational measures to protect personal data from unauthorized access, loss, or destruction. This may include implementing measures such as encryption, access control, and data breach monitoring.
Obtain consent from individuals: Organizations must obtain consent from individuals before collecting or processing their personal data. This includes obtaining consent for the use of AI systems to process personal data.
Provide individuals with access to their personal data: Individuals have the right to access their personal data and to have it erased. Organizations must provide individuals with a way to exercise these rights.
Be transparent and accountable: Organizations must be transparent with individuals about how their personal data is being used in AI systems. Organizations must also be accountable for the decisions made by AI systems.
Conclusion: Responsible Innovation in the AI-GDPR Era
AI undeniably holds immense potential for transformative change. However, this potential comes hand-in-hand with significant responsibilities. Navigating the legal landscape of data protection, particularly in the context of GDPR, is not merely a legal requirement but a testament to an organization’s commitment to ethical AI practices.
In the AI-GDPR era, responsible innovation becomes the key to achieving a harmonious future where technology enhances lives while respecting privacy and individual rights. As businesses and individuals alike navigate this intricate landscape, it is essential to prioritize transparency, informed consent, and robust data security. In doing so, organizations can not only achieve compliance but also build and maintain trust with customers and users in the ever-evolving digital ecosystem.
Subscribe to my newsletter
Read articles from Atharv Patil directly inside your inbox. Subscribe to the newsletter, and don't miss out.
Written by
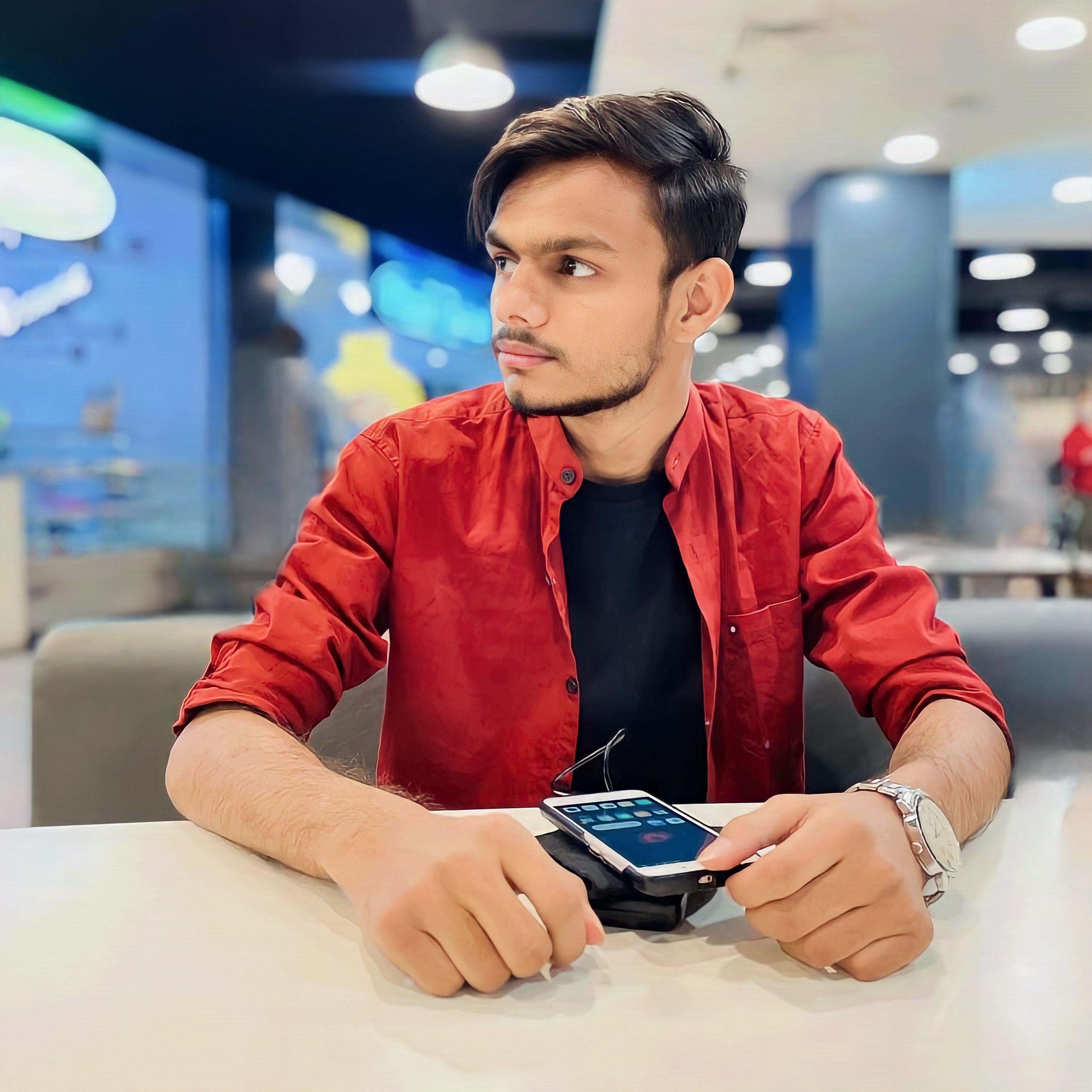
Atharv Patil
Atharv Patil
Encrypting my life one bit at a time from the comforts of 127.0.0.1