Mastering Prompt Engineering: The Art of Creating Effective AI-Language Model Prompts

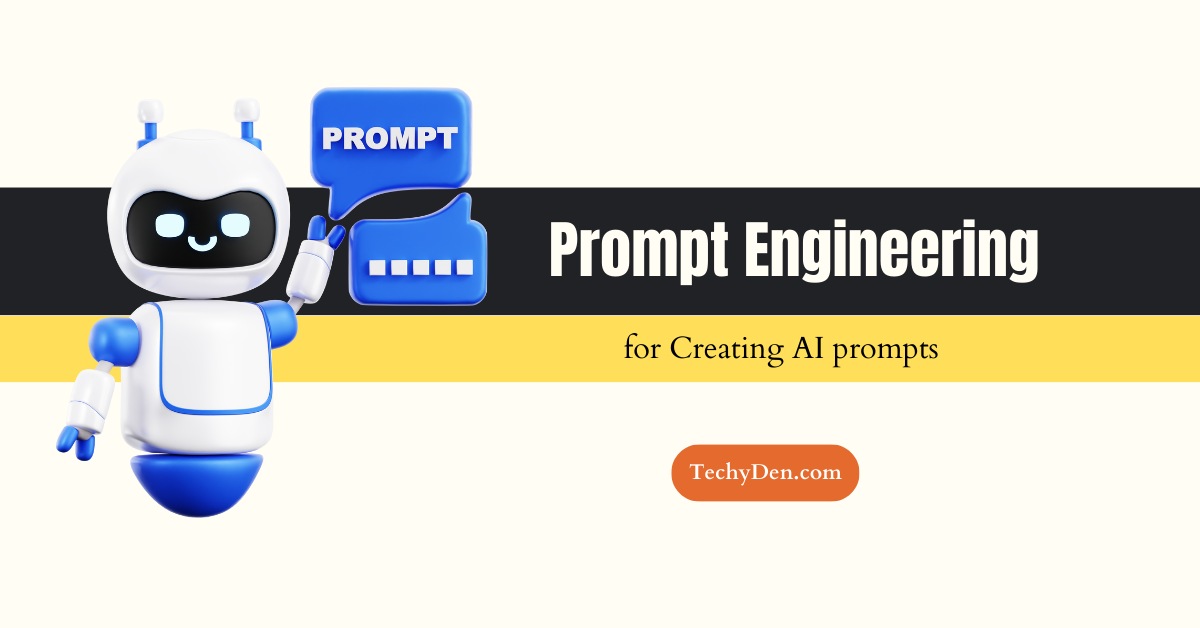
In the rapidly advancing world of artificial intelligence, language models have emerged as game-changing tools capable of generating human-like text, answering complex questions, and tackling creative writing tasks with remarkable prowess. However, the effectiveness of these AI systems hinges heavily on the quality of the prompts or instructions provided to them. This is where the art of prompt engineering comes into play.
Prompt engineering refers to the meticulous process of designing and refining input prompts to elicit desired outputs from AI language models. These prompts serve as the initial guidance for the model, shaping the context, tone, and direction of the generated text. Practical prompt engineering involves carefully curating the prompt's wording, structure, and framing to optimize the model's performance and align its responses with the intended goals.
The Principles of Effective Prompt Engineering
Crafting compelling prompts is a delicate balance of art and science, guided by several fundamental principles that are essential for achieving success:
Clarity and Specificity: Prompts should be clear, concise, and unambiguous, leaving little room for misinterpretation. Providing specific instructions and context helps the model better understand the desired output, reducing the likelihood of irrelevant or off-topic responses.
Task Framing: Properly framing the task or goal within the prompt is crucial. This involves explicitly stating the objective, such as generating a blog post, answering a question, or summarizing a text and providing necessary contextual information to guide the model's response.
Prompt Structure: The structure of the prompt is not just a formality but a powerful tool that can influence the model's interpretation and output. Well-structured prompts often begin with background information, followed by specific questions or instructions, and end with examples or suggested output formats.
Priming and Few-Shot Learning: Priming involves providing the model with a few examples of desired outputs, known as few-shot learning. This technique can help the model grasp the intended format, tone, and style, improving the quality of its generated text.
Iterative Refinement: Prompt engineering is a continuous and iterative process. By analyzing the model's outputs and refining the prompts, developers can steadily enhance the quality and accuracy of the generated text, ensuring consistent improvement.
Context Awareness: Effective prompts consider the broader context in which the model's output will be used or consumed, including the target audience, domain, and intended application. This context-aware approach ensures the generated text is appropriate, relevant, and tailored to the specific situation.
Ethical Considerations: As language models can potentially generate biased, harmful, or inappropriate content, prompt engineering must incorporate ethical principles and guidelines, actively promoting fairness, inclusivity, and responsible language generation.
The Importance of Prompt Engineering
Prompt engineering plays a pivotal role in the success of language model applications across various domains. Well-crafted prompts can significantly enhance the quality, relevance, and coherence of the generated text, ensuring that the model's outputs meet the specific requirements of the task at hand. Conversely, poorly designed prompts can lead to suboptimal or nonsensical outputs, undermining the model's capabilities and limiting its usefulness.
Applications of Prompt Engineering
The impact of prompt engineering spans a wide range of industries and domains, including:
Content Generation: Crafting effective prompts enables language models to generate high-quality content, such as articles, blog posts, stories, and marketing copy, streamlining the content creation process.
Question Answering: By providing well-designed prompts, language models can serve as robust question-answering systems, retrieving relevant information from vast datasets and providing concise and accurate responses.
Summarization and Analysis: Prompt engineering facilitates the creation of summaries, reports, and analyses from large volumes of text, allowing for efficient information extraction and synthesis.
Creative Writing: Language models can be prompted to engage in creative writing tasks, such as generating poetry, fiction, or screenplays, opening new avenues for artistic expression and collaboration between humans and AI.
Customer Service and Conversational AI: Effective prompts can enhance the capabilities of conversational AI assistants, enabling them to understand and respond to user queries better, improving the overall customer experience.
Challenges and Considerations
While prompt engineering offers significant benefits, it also presents several challenges and considerations:
Bias and Ethical Concerns: Language models can inadvertently perpetuate biases present in their training data or reflect societal biases through their outputs. Prompt engineering must address these issues by incorporating debiasing techniques and promoting fair and ethical language generation.
Domain Specificity: Crafting effective prompts often requires domain-specific knowledge and expertise. Developers may need to collaborate with subject matter experts to ensure prompts accurately capture the nuances and terminology of a particular field.
Scalability and Consistency: As the number of prompts and use cases grows, maintaining consistency and ensuring scalability become increasingly challenging. Developing standardized prompt engineering practices and tools can help address this issue.
Intellectual Property and Privacy: Language models can generate text that infringes on intellectual property rights or compromises sensitive information. Prompt engineering strategies must consider these risks and implement appropriate safeguards.
Best Practices for Prompt Engineering
To optimize the effectiveness of prompt engineering, developers and organizations can adopt the following best practices:
Collaborate and Iterate: Involve diverse stakeholders, subject matter experts, and end-users in the prompt engineering process. Continuously refine and iterate on prompts based on feedback and performance evaluations.
Maintain Style Guides: Develop style guides and templates for prompt creation, ensuring consistency in tone, structure, and formatting across different prompts and use cases.
Leverage Prompt Libraries: Build and maintain libraries of well-crafted prompts for everyday tasks or domains, allowing for easy reuse and adaptation.
Implement Prompt Testing and Validation: Establish processes for testing and validating prompts, including techniques like human evaluation, automated metrics, and adversarial testing, to identify and mitigate potential biases or issues.
Stay Updated: Stay informed about the latest advancements, techniques, and best practices in prompt engineering as the field evolves rapidly.
By mastering the art of prompt engineering, developers and organizations can unlock the full potential of AI language models, enabling a wide range of applications and driving innovation across various domains. As this field continues to evolve, collaborative efforts, best practices, and ethical considerations will be crucial in shaping the future of prompt engineering and ensuring its responsible and impactful use.
Subscribe to my newsletter
Read articles from Satish Kumar Ithamsetty directly inside your inbox. Subscribe to the newsletter, and don't miss out.
Written by

Satish Kumar Ithamsetty
Satish Kumar Ithamsetty
Satish Kumar Ithamsetty is a professional blogger and tech enthusiast. He has a background in biochemistry, having worked as a lecturer in the field. Despite his busy schedule, he finds time to pursue his passion for blogging. Satish is particularly interested in writing about blogging concepts, SEO, affiliate marketing, monetization methods, and YouTube. His knowledge and experience in these areas make him a valuable resource for anyone looking to start or improve their own blog.