Calculating Moving Averages in Python: A Comprehensive Guide

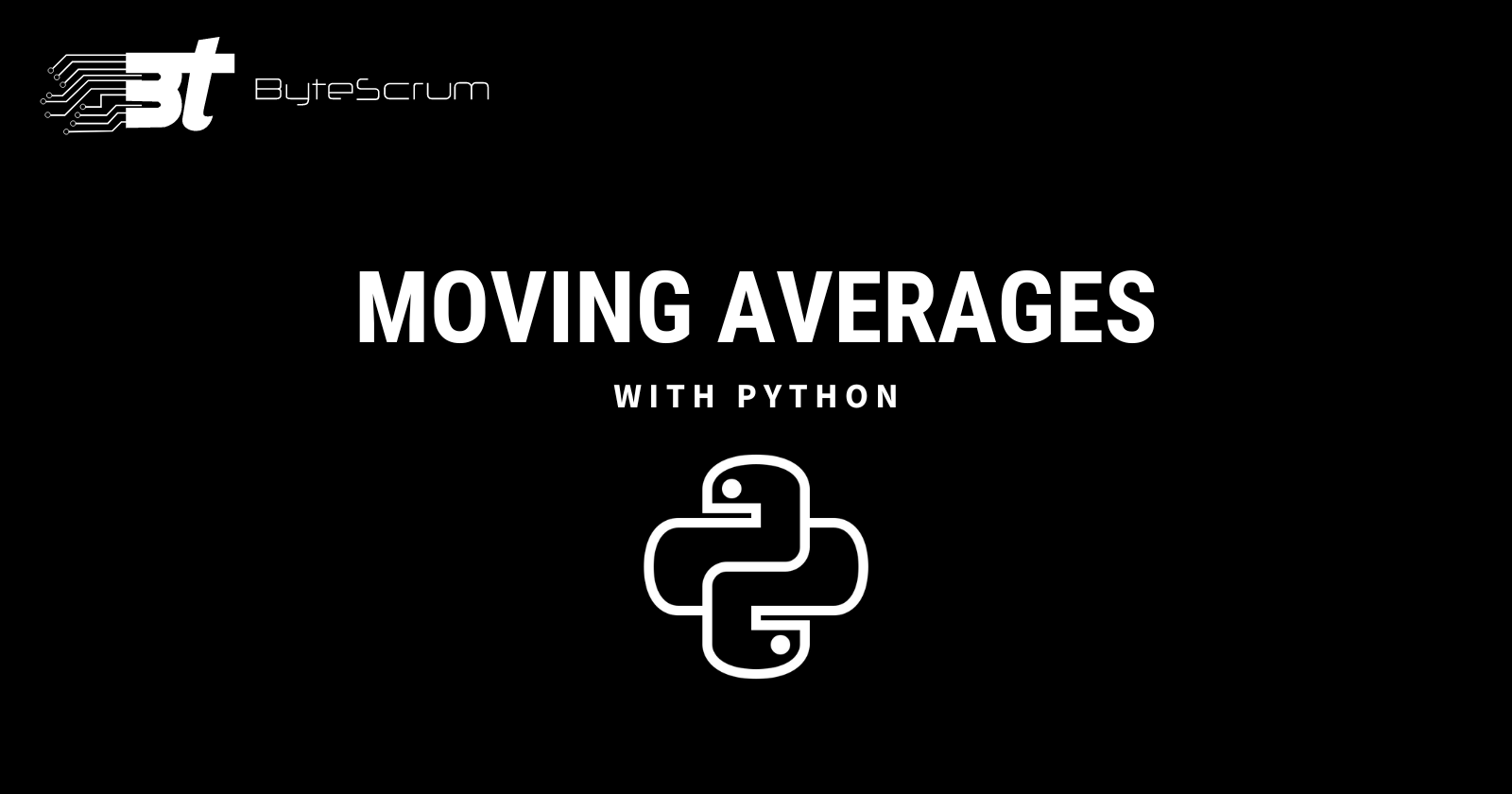
Moving averages are essential tools in data analysis and financial markets, used to smooth out short-term fluctuations and highlight longer-term trends. In this blog, we'll explore how to calculate different types of moving averages in Python, using popular libraries like Pandas.
Understanding Moving Averages
A moving average is a statistical technique used to analyze data points by creating a series of averages of different subsets of the full data set. There are several types of moving averages:
Simple Moving Average (SMA): The unweighted mean of the previous n data points.
Exponential Moving Average (EMA): A weighted mean where more recent data points have more influence.
Weighted Moving Average (WMA): Similar to EMA, but uses different weights for each data point.
Prerequisites
Before diving into the code, make sure you have the following libraries installed:
Pandas: For data manipulation.
pip install pandas
NumPy: For numerical operations.
pip install numpy
Importing Libraries and Sample Data
Let's start by importing the necessary libraries and creating a sample dataset.
import pandas as pd
import numpy as np
import matplotlib.pyplot as plt
# Sample data
data = {
'Date': pd.date_range(start='1/1/2020', periods=100),
'Price': np.random.randn(100).cumsum() + 100
}
df = pd.DataFrame(data)
df.set_index('Date', inplace=True)
print(df.head())
Calculating Simple Moving Average (SMA)
The Simple Moving Average (SMA) is the most straightforward moving average. It's calculated by taking the average of a fixed number of previous periods.
def calculate_sma(data, window):
return data.rolling(window=window).mean()
# Calculate 10-day SMA
df['SMA_10'] = calculate_sma(df['Price'], 10)
print(df.head(15))
Calculating Exponential Moving Average (EMA)
The Exponential Moving Average (EMA) gives more weight to recent prices, making it more responsive to new information.
def calculate_ema(data, span):
return data.ewm(span=span, adjust=False).mean()
# Calculate 10-day EMA
df['EMA_10'] = calculate_ema(df['Price'], 10)
print(df.head(15))
Calculating Weighted Moving Average (WMA)
The Weighted Moving Average (WMA) assigns different weights to each data point, with the most recent data points usually having the highest weights.
def calculate_wma(data, window):
weights = np.arange(1, window + 1)
return data.rolling(window).apply(lambda prices: np.dot(prices, weights) / weights.sum(), raw=True)
# Calculate 10-day WMA
df['WMA_10'] = calculate_wma(df['Price'], 10)
print(df.head(15))
Visualizing Moving Averages
Visualizing moving averages can provide a clearer picture of the underlying trends in the data.
plt.figure(figsize=(14, 7))
plt.plot(df['Price'], label='Price', linewidth=2)
plt.plot(df['SMA_10'], label='10-day SMA', linestyle='--')
plt.plot(df['EMA_10'], label='10-day EMA', linestyle='--')
plt.plot(df['WMA_10'], label='10-day WMA', linestyle='--')
plt.title('Price and Moving Averages')
plt.xlabel('Date')
plt.ylabel('Price')
plt.legend()
plt.show()
Conclusion
Keep exploring these techniques to enhance your data analysis skills and apply them to various datasets and scenarios.
Subscribe to my newsletter
Read articles from ByteScrum Technologies directly inside your inbox. Subscribe to the newsletter, and don't miss out.
Written by

ByteScrum Technologies
ByteScrum Technologies
Our company comprises seasoned professionals, each an expert in their field. Customer satisfaction is our top priority, exceeding clients' needs. We ensure competitive pricing and quality in web and mobile development without compromise.