Beyond "Explainable AI (XAI): Lifting the Black Box Lid''
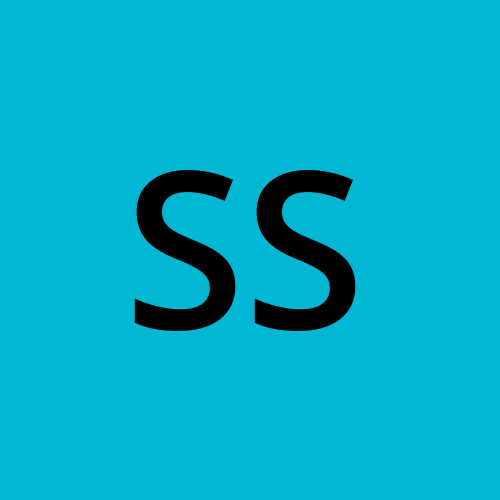
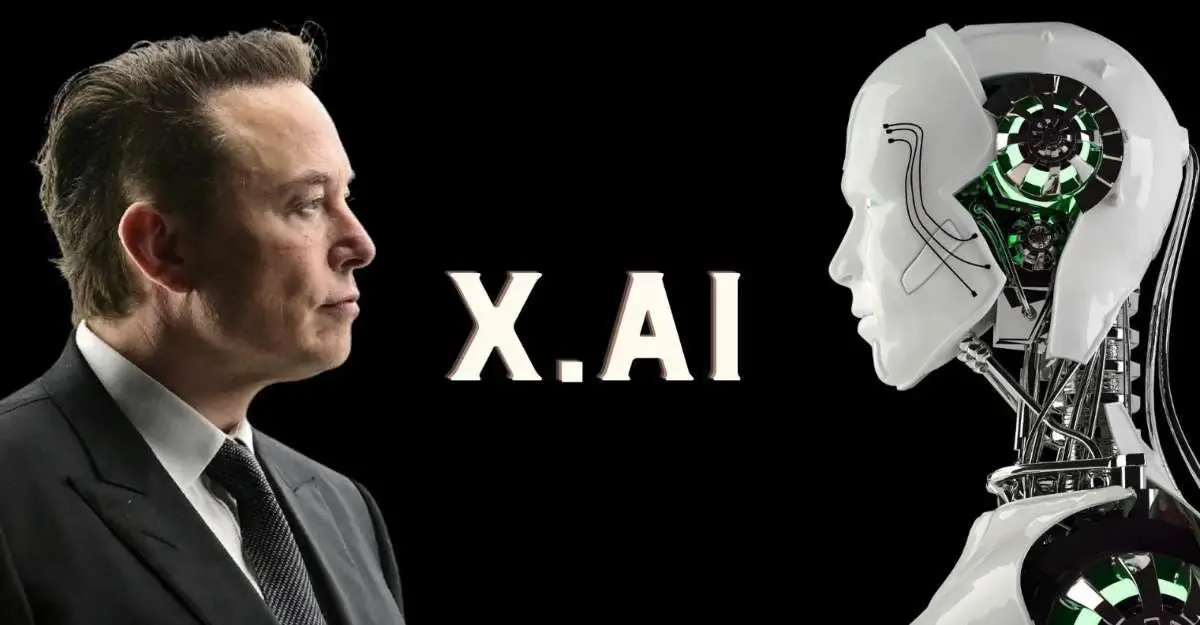
Artificial intelligence (AI) has become a ubiquitous force, transforming industries and reshaping our world. However, the inner workings of complex AI models, often referred to as "black boxes," can raise concerns about transparency and bias. This lack of explainability can hinder trust and limit the responsible application of AI. Enter Explainable AI (XAI), a burgeoning field dedicated to demystifying AI models and fostering trust in their decision-making processes. Data science programs are playing a crucial role in equipping professionals with the skills to develop and understand XAI techniques.
This blog delves into the world of XAI, exploring its core principles, applications, and the increasing importance of data science programs in nurturing XAI expertise.
Demystifying the Black Box: Unveiling the Need for XAI
The power of AI comes at a cost – the opaqueness of complex models. Here's why XAI is becoming increasingly important:
Understanding Decision-Making: Traditional AI models can be opaque, making it difficult to understand how they arrive at their outputs. XAI techniques aim to shed light on the reasoning behind these decisions, fostering trust and confidence in their applications.
Mitigating Bias: AI models are susceptible to biases present in the data they are trained on. XAI techniques can help identify and address these biases, ensuring fairness and ethical use of AI.
Improved Human-AI Collaboration: When humans understand how AI models work, they can better collaborate with them, leading to more effective and reliable outcomes.
Lifting the Lid: Techniques and Approaches in XAI
Several XAI techniques are used to peel back the layers of complex AI models:
Model-Agnostic Methods: These techniques are independent of the specific AI model and focus on explaining the model's overall predictions. Techniques like feature importance analysis help identify which features in the data have the greatest influence on the model's outputs.
Model-Specific Methods: These techniques leverage the internal workings of specific AI models to explain their decisions. For example, in rule-based systems, the explanation focuses on the rules that triggered the prediction.
Counterfactual Explanations: This technique explores what changes in the input data would lead to different model outputs. This can help explain why a specific prediction was made for a particular data point.
Data Science Programs: Equipping the Workforce for XAI
The rise of XAI necessitates a skilled workforce equipped to develop, deploy, and interpret XAI models. Here's where data science programs come into play:
Foundational Knowledge in AI and Machine Learning: These programs provide a strong foundation in machine learning algorithms, the building blocks of AI. Students gain an understanding of how different algorithms work, laying the groundwork for understanding how to explain their outputs.
Courses in XAI Techniques: Many data science programs now offer dedicated courses in XAI techniques. These courses delve into the various methods employed to explain AI models, equipping students with the necessary skillset to implement XAI in real-world applications.
Developing Critical Thinking Skills: Data science programs foster critical thinking skills crucial for XAI. Students learn to analyze AI models, identify potential biases, and communicate the limitations and explainability levels of these models.
Hands-on Learning with XAI Tools: Modern data science programs incorporate practical projects utilizing XAI tools. Students gain hands-on experience applying XAI techniques to real-world data sets, preparing them to tackle XAI challenges in their future careers.
Beyond the Black Box: A Future of Transparency and Trust
XAI holds the key to unlocking the full potential of AI. By fostering transparency and trust in AI models, XAI can pave the way for responsible and ethical applications across diverse industries. Data science programs will continue to be at the forefront of this evolution, nurturing a generation of data scientists equipped to develop and utilize XAI techniques effectively.
This collaborative effort between AI developers, XAI experts, and policymakers will usher in a future where AI operates with transparency, fosters trust, and empowers us to harness its power for good.
Subscribe to my newsletter
Read articles from sanjana shah directly inside your inbox. Subscribe to the newsletter, and don't miss out.
Written by
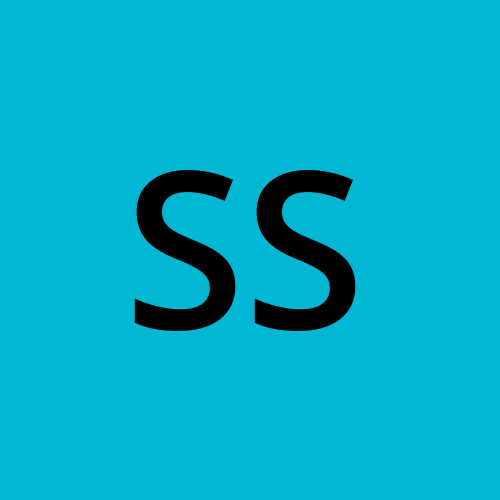