Box Plots: A Key Visualization for Data Analysis

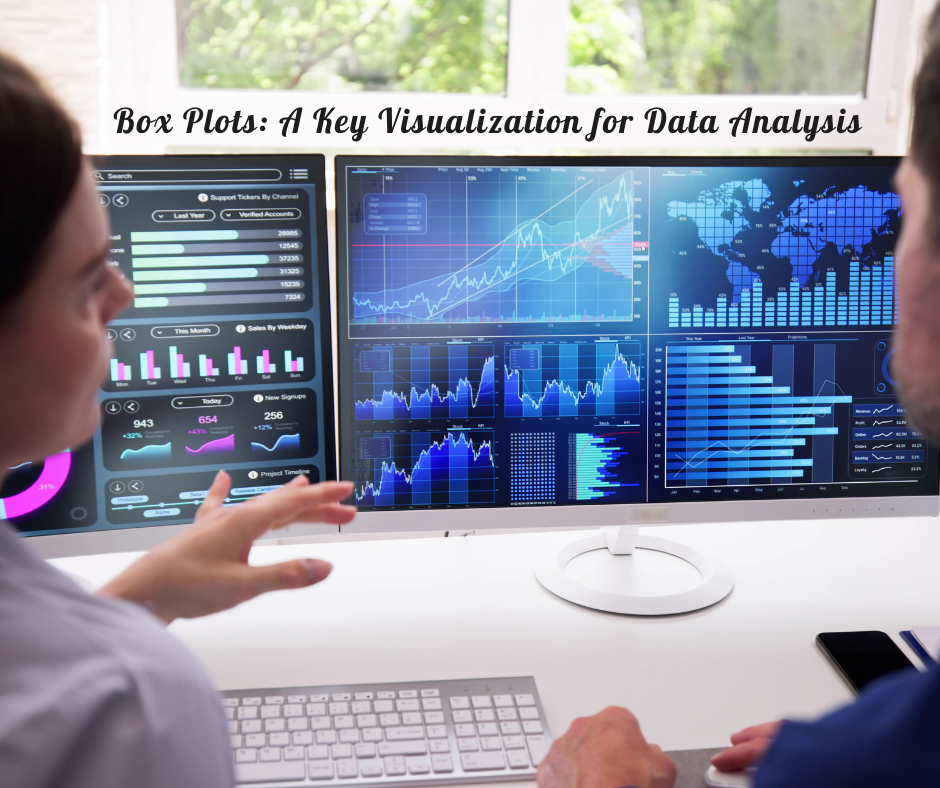
Box plots, also known as box-and-whisker plots, are essential tools in data analysis and statistics. They provide a visual summary of a dataset, highlighting its central tendency, dispersion, and potential outliers. This guide will explain the key components of box plots, their advantages, how to interpret them, and their applications in data analysis.
What is a Box Plot?
A box plot is a standardized way of displaying the distribution of data based on a five-number summary: minimum, first quartile (Q1), median (Q2), third quartile (Q3), and maximum. These plots are particularly useful for comparing distributions across different groups or datasets.
Key Components of a Box Plot
Box: The box represents the interquartile range (IQR), which contains the middle 50% of the data.
Median Line: A line inside the box indicates the median (Q2) of the dataset.
Whiskers: Lines extending from the box to the minimum and maximum values within 1.5 times the IQR from Q1 and Q3.
Outliers: Points outside the whiskers represent outliers, which are values that are significantly higher or lower than the rest of the data.
Five-Number Summary
Minimum: The smallest data point excluding outliers.
Median (Q2): The 50th percentile or the middle value of the data.
Third Quartile (Q3): The 75th percentile of the data.
Maximum: The largest data point excluding outliers.
Why Use Box Plots?
Box plots offer several advantages:
Simplicity: They provide a clear and concise summary of data distribution.
Comparison: They make it easy to compare multiple datasets or groups.
Outlier Detection: They highlight outliers, which can be critical for understanding data variability.
Skewness Identification: They help identify the skewness of the data distribution.
How to Interpret Box Plots
Understanding box plots involves recognizing the position and spread of the data.
Center and Spread
Median Line Position: If the median line is near the center of the box, the data is symmetrically distributed. If it’s closer to Q1 or Q3, the data is skewed.
Box Width: A wider box indicates more variability within the middle 50% of the data.
Whisker Length: Longer whiskers suggest greater variability outside the middle 50% of the data.
Outliers
Above the Whiskers: Outliers above the upper whisker are higher than expected.
Below the Whiskers: Outliers below the lower whisker are lower than expected.
Skewness
Left-Skewed: If the median is closer to Q3 and the left whisker is longer, the data is left-skewed.
Right-Skewed: If the median is closer to Q1 and the right whisker is longer, the data is right-skewed.
Comparing Groups
Box plots are particularly useful for comparing distributions across different groups. By aligning multiple box plots side by side, you can quickly assess differences in medians, variability, and outliers among groups.
Applications of Box Plots
Box plots are versatile tools used in various fields for different purposes:
1. Descriptive Statistics
Box plots provide a visual summary of data, making them ideal for presenting descriptive statistics. They help convey essential information about the central tendency, dispersion, and outliers of a dataset.
2. Comparative Analysis
Box plots are effective for comparing distributions across different groups. For example, they can compare the test scores of students from different schools, the performance of different products, or the income levels across different regions.
3. Outlier Detection
Identifying outliers is crucial in many analyses, especially in fields like finance, where outliers might indicate fraud, or in quality control, where outliers could signify defects. Box plots make outlier detection straightforward and visually intuitive.
4. Skewness Detection
Box plots help identify skewness in data distributions. Understanding whether data is skewed to the left or right can influence the choice of statistical methods and models for further analysis.
5. Data Cleaning
Box plots assist in data cleaning by highlighting outliers and inconsistencies. Analysts can use box plots to identify data points that require further investigation or correction.
6. Exploratory Data Analysis (EDA)
During EDA, box plots are used to explore data characteristics before applying more complex statistical or machine learning models. They provide a quick overview of the data, helping analysts make informed decisions about subsequent steps.
Example Scenarios
Example 1: Comparing Test Scores
Imagine you have test scores from three different classes and want to compare their performance. By plotting box plots for each class, you can easily compare their medians, variability, and identify any outliers.
Example 2: Analyzing Sales Data
Box plots can help compare the sales distributions, identify regions with consistent sales, and highlight regions with significant variability or outliers.
Example 3: Quality Control
In a manufacturing setting, box plots can be used to monitor the quality of products. By comparing box plots of defect rates across different production lines, you can identify lines with higher variability or outliers, indicating potential issues in the production process.
Limitations of Box Plots
While box plots are powerful tools, they have some limitations:
Loss of Detail: Box plots summarize data, which means they may omit detailed information about the distribution.
Sensitivity to Sample Size: Box plots can be less informative with small sample sizes.
Non-Symmetric Data: Box plots assume some degree of symmetry. Highly skewed data might require additional context or complementary visualizations.
Best Practices for Creating Box Plots
To ensure your box plots are effective and informative, consider the following best practices:
Label Clearly: Clearly label the axes and include a legend if comparing multiple groups.
Use Consistent Scales: When comparing groups, use consistent scales for accurate comparisons.
Highlight Outliers: Ensure outliers are visible and clearly marked.
Provide Context: Include additional context or annotations to help interpret the plot.
Combine with Other Visualizations: Use box plots in conjunction with other visualizations, like histograms or scatter plots, for a more comprehensive analysis.
Conclusion
Box plots are indispensable tools in data analysis, offering a clear and concise way to visualize data distributions, compare groups, and identify outliers. By understanding their key components and how to interpret them, you can leverage box plots to gain valuable insights into your data naturally. Whether you're a student, analyst, or researcher, mastering box plots will enhance your ability to communicate findings and make data-driven decisions effectively. For those interested in the Best Data Analytics Training Course in Delhi, Noida, Mumbai, Indore, and other parts of India, acquiring these skills can significantly elevate your proficiency in data analysis and interpretation.
Subscribe to my newsletter
Read articles from Ruhi Parveen directly inside your inbox. Subscribe to the newsletter, and don't miss out.
Written by

Ruhi Parveen
Ruhi Parveen
I am a Digital Marketer and Content Marketing Specialist, I enjoy technical and non-technical writing. I enjoy learning something new.