Statistical Genetics
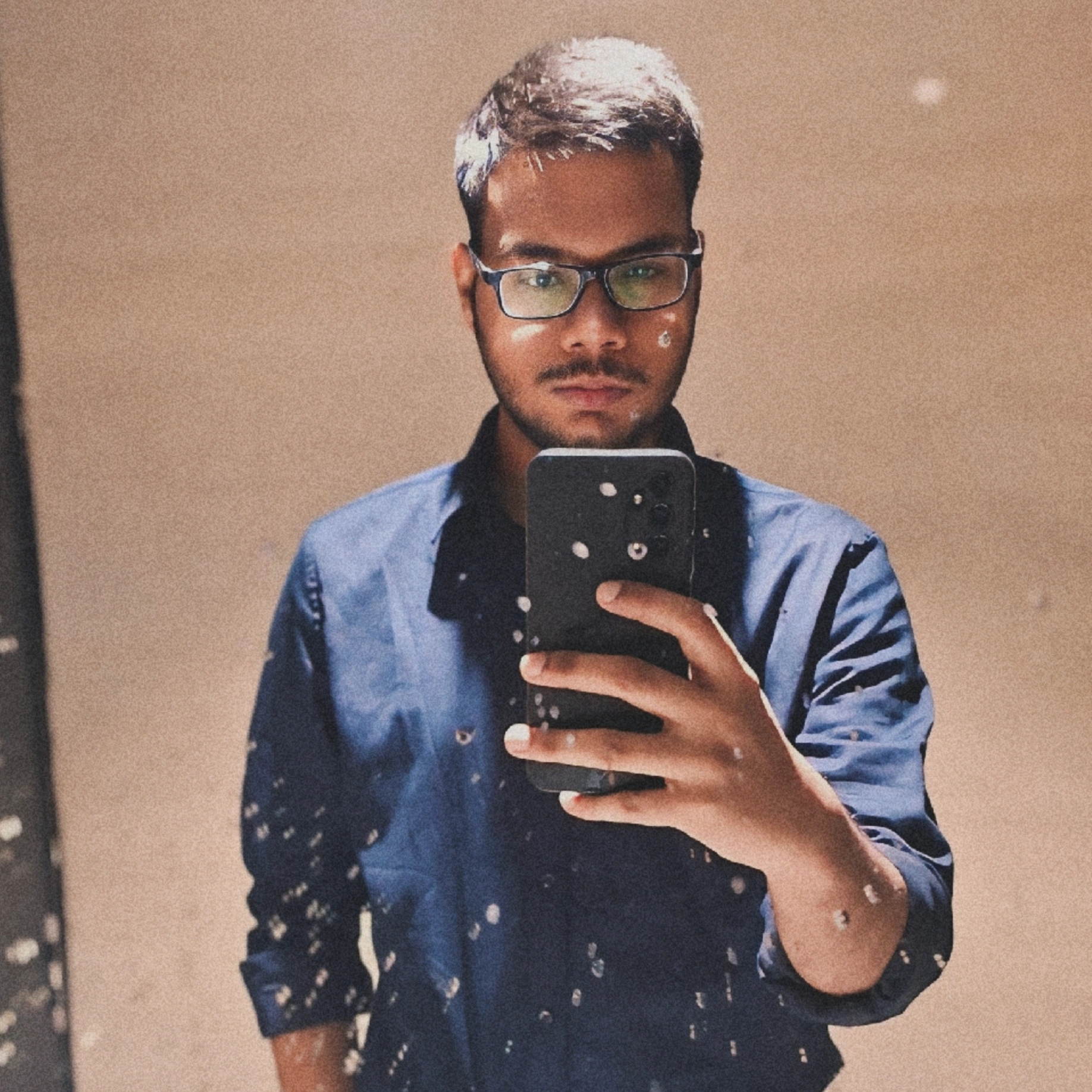
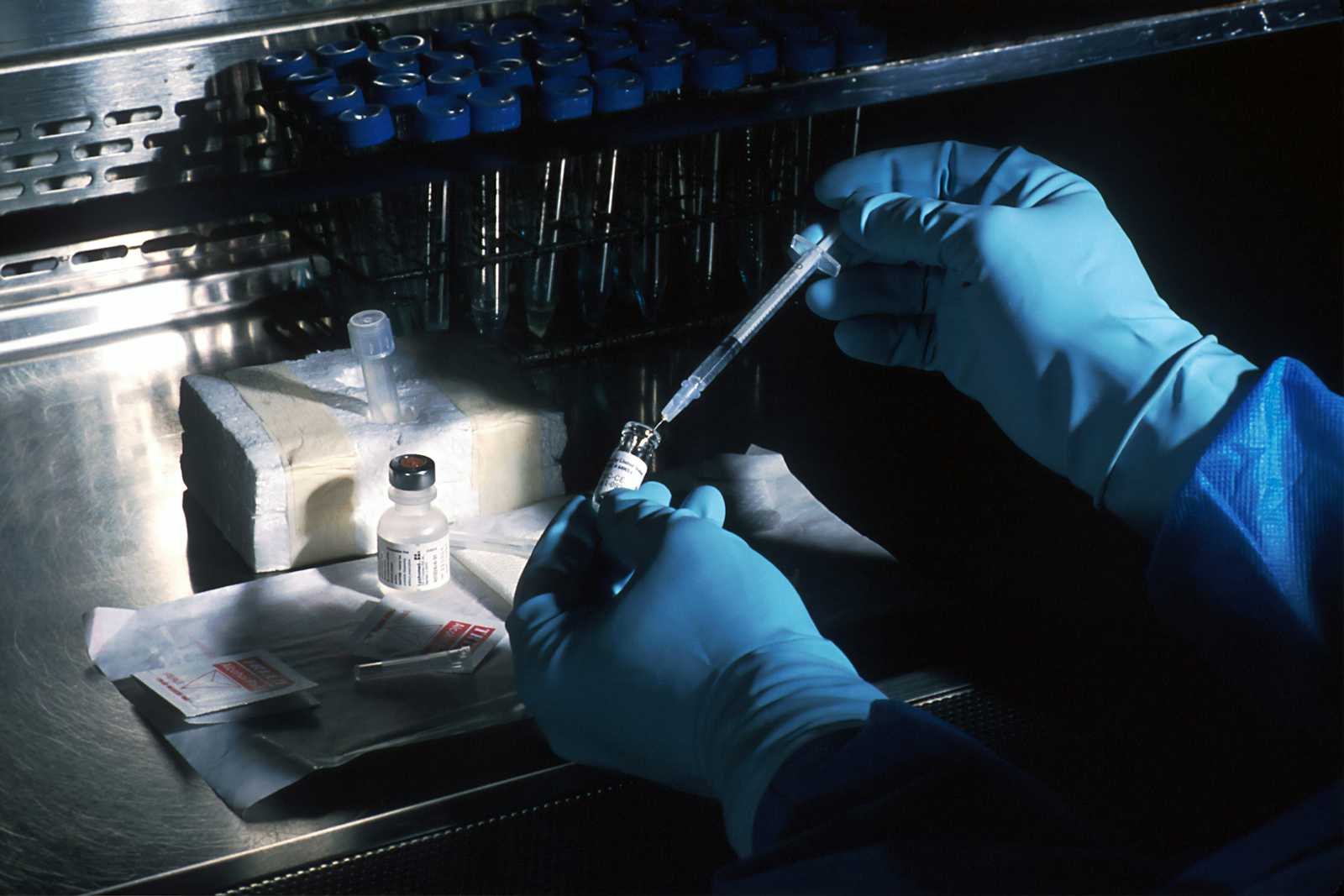
1. Introduction to Statistical Genetics:
- Definition: Statistical genetics is a field that applies statistical methods to understand the genetic basis of complex traits and diseases. It involves the analysis of genetic data to identify associations between genetic variants and phenotypes.
2. Key Concepts:
Phenotype: Observable characteristics or traits of an organism resulting from the interaction of its genotype with the environment.
Genotype: The genetic makeup of an organism, typically referring to specific genetic variants.
Alleles: Different versions of a gene at a particular locus.
Polymorphism: The presence of two or more genetically distinct variants in a population.
3. Genetic Variation:
Single Nucleotide Polymorphisms (SNPs): The most common type of genetic variation, involving a change of a single nucleotide.
Insertions/Deletions (Indels): Variations involving the insertion or deletion of small DNA segments.
Copy Number Variants (CNVs): Larger segments of DNA that are duplicated or deleted.
Structural Variants: Large-scale structural changes in the genome, such as inversions, translocations, and duplications.
4. Heritability:
Definition: The proportion of phenotypic variance in a population that is attributable to genetic variance.
Types:
Broad-Sense Heritability (H²): Includes all genetic contributions to phenotypic variance (additive, dominance, epistatic).
Narrow-Sense Heritability (h²): Includes only additive genetic variance, which is most relevant for predicting response to selection.
5. Quantitative Trait Loci (QTL) Mapping:
Definition: A method to identify regions of the genome associated with quantitative traits.
Process:
Phenotyping: Measure the trait of interest in a population.
Genotyping: Obtain genetic markers across the genome.
Linkage Analysis: Identify correlations between markers and trait variation.
Statistical Methods: Interval mapping, composite interval mapping, and multiple-QTL mapping.
6. Genome-Wide Association Studies (GWAS):
Definition: A study approach that involves scanning the genome to find genetic variants associated with a trait.
Process:
Sample Collection: Obtain DNA samples from a large number of individuals.
Genotyping: Use high-throughput methods to genotype millions of SNPs.
Statistical Analysis: Use methods like linear regression, logistic regression, and mixed models to test for associations.
Multiple Testing Correction: Methods like Bonferroni correction and False Discovery Rate (FDR) to account for the large number of tests performed.
7. Population Genetics:
Hardy-Weinberg Equilibrium: A principle stating that allele and genotype frequencies in a population will remain constant from generation to generation in the absence of evolutionary influences.
Genetic Drift: Random changes in allele frequencies due to sampling variation.
Selection: Differential survival and reproduction of individuals due to differences in phenotype.
Migration: Movement of individuals between populations, introducing new genetic variation.
Mutation: Changes in the DNA sequence that introduce new alleles into a population.
Effective Population Size (Ne): The number of individuals in a population who contribute offspring to the next generation.
8. Statistical Models in Genetics:
Linear Models: Used to model the relationship between genetic variants and quantitative traits.
Mixed Models: Include both fixed effects (genetic variants) and random effects (polygenic background) to account for population structure and relatedness.
Bayesian Methods: Use prior distributions and Markov Chain Monte Carlo (MCMC) techniques for parameter estimation.
Machine Learning: Techniques such as random forests, support vector machines, and neural networks for predictive modeling and classification.
9. Data Sources and Software Tools:
Databases: Public repositories like dbSNP, 1000 Genomes Project, HapMap, and UK Biobank.
Software: PLINK, GEMMA, GCTA, TASSEL, and R packages for statistical genetics.
10. Ethical Considerations:
Privacy and Data Security: Ensuring the confidentiality of genetic data.
Informed Consent: Obtaining consent from participants for genetic studies.
Data Sharing: Balancing the benefits of data sharing with the need to protect individual privacy.
Genetic Discrimination: Preventing discrimination based on genetic information.
11. Applications of Statistical Genetics:
Personalized Medicine: Using genetic information to tailor medical treatments to individuals.
Disease Gene Discovery: Identifying genetic variants that contribute to the risk of diseases.
Evolutionary Biology: Understanding the genetic basis of adaptation and speciation.
Agriculture: Breeding programs to improve crop yield, disease resistance, and other traits.
12. Challenges and Future Directions:
Complex Traits: Understanding the genetic architecture of traits influenced by many genes and environmental factors.
Big Data: Managing and analyzing large-scale genetic datasets.
Integration with Other Omics: Combining genetic data with transcriptomic, proteomic, and metabolomic data for a comprehensive understanding of biological processes.
Functional Genomics: Linking genetic variants to their biological functions and mechanisms.
By applying statistical methods to genetic data, statistical genetics helps unravel the complex relationship between genotype and phenotype, advancing our understanding of biology and improving human health and agriculture.
Subscribe to my newsletter
Read articles from Anshuman Sinha directly inside your inbox. Subscribe to the newsletter, and don't miss out.
Written by
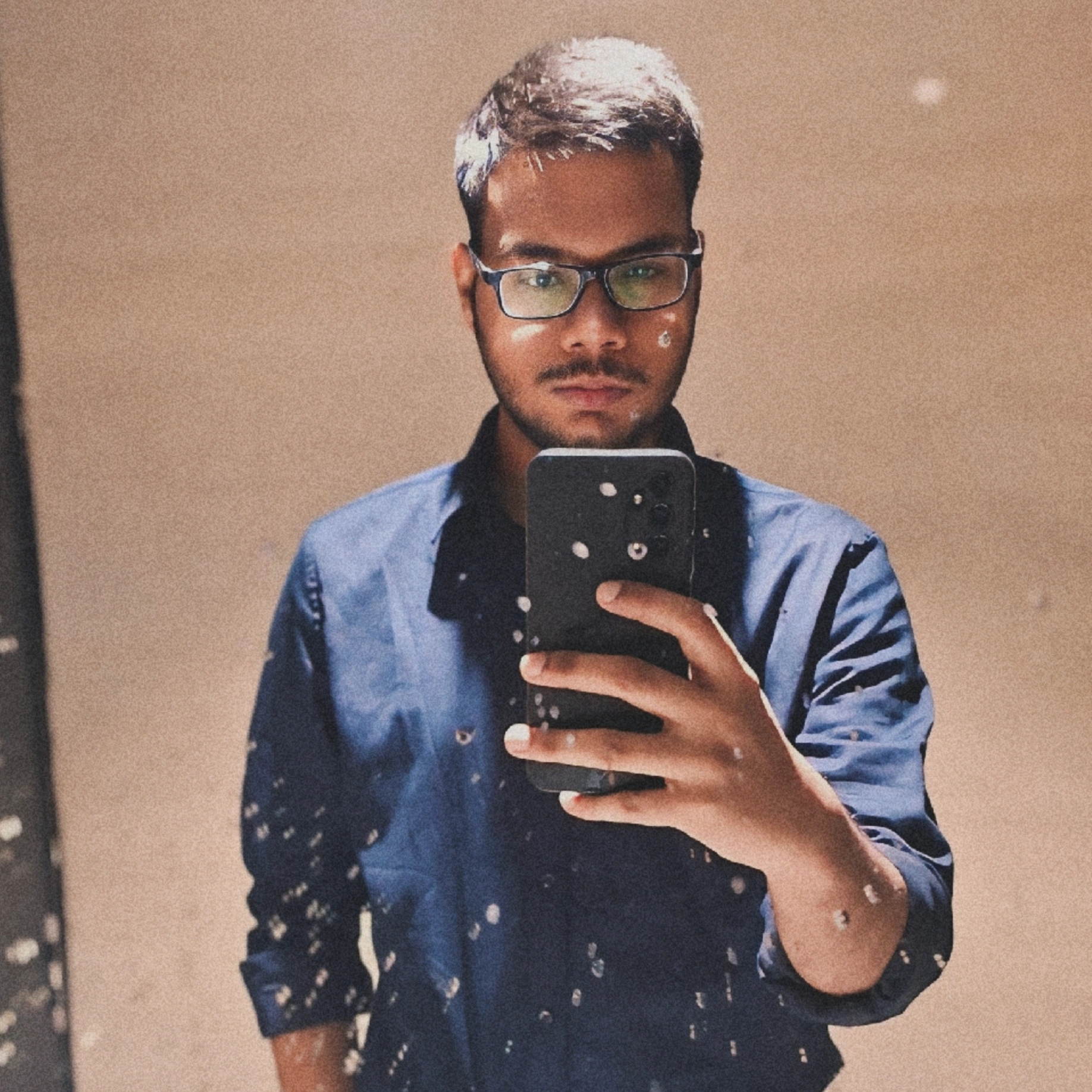
Anshuman Sinha
Anshuman Sinha
Software Developer who previously worked as an SDE Intern at a consulting firm and as a Data Science intern at an IT Firm. Currently pursuing BCA from Amity University Patna.