Machine Learning in Cybersecurity: Detecting and Preventing Threats with AI

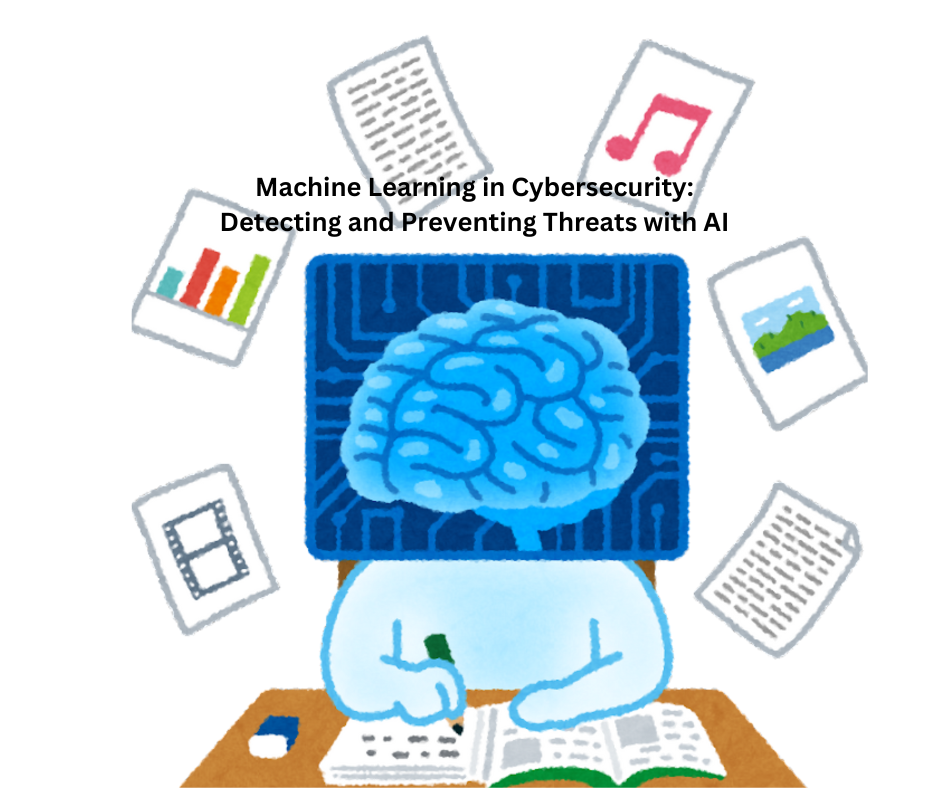
In today’s digital age, cybersecurity threats are becoming increasingly sophisticated and frequent. Traditional security measures often struggle to keep up with the evolving nature of these threats. Enter machine learning (ML) and artificial intelligence (AI), which are revolutionizing the way we detect and prevent cyber threats. This article explores how machine learning is enhancing cybersecurity, providing a deeper understanding of its applications and benefits.
What is Machine Learning?
Machine learning is a branch of artificial intelligence (AI) where computers learn from data to get better at tasks over time without being specifically programmed for each task. Instead of being told exactly what to do, these systems use algorithms and statistical models to find patterns in data, make decisions, and predict future outcomes based on what they have learned from past information.
How Machine Learning Enhances Cybersecurity
1. Threat Detection
Anomaly Detection: Machine learning algorithms can analyze vast amounts of data to identify unusual patterns that may indicate a security threat. For instance, if a network is experiencing traffic that deviates significantly from its normal behavior, machine learning models can flag this anomaly as a potential threat.
Malware Detection: Traditional antivirus solutions rely on signature-based detection, which requires knowing about a threat before it can be detected. Machine learning models, however, can identify new and unknown malware by analyzing the behavior and characteristics of files, even if they have never been encountered before.
Phishing Detection: Phishing attacks often involve deceptive emails or websites designed to steal sensitive information. Machine learning algorithms can scrutinize email content and URLs to detect suspicious patterns or anomalies, helping to filter out phishing attempts before they reach users.
2. Threat Prevention
Behavioral Analysis: Machine learning can continuously monitor user behavior to establish a baseline of normal activity. If a user's behavior deviates from this baseline, the system can automatically respond to mitigate potential threats. For example, if an employee suddenly accesses sensitive files they don’t normally work with, an alert can be triggered, and access can be restricted.
Automated Response: Machine learning models can be integrated with cybersecurity tools to automate responses to detected threats. For example, if an intrusion attempt is detected, the system can automatically isolate affected systems or block malicious IP addresses without requiring human intervention.
Predictive Analytics: Machine learning can analyze historical attack data to predict future threats. By identifying trends and patterns, organizations can proactively enhance their security measures and prepare for potential vulnerabilities before they are exploited.
Key Applications of Machine Learning in Cybersecurity
1. Intrusion Detection Systems (IDS)
Machine learning enhances Intrusion Detection Systems by improving their ability to identify and respond to malicious activities. Traditional IDS rely on predefined rules, which may not be sufficient for detecting sophisticated attacks. Machine learning-based IDS can learn from historical attack data and adapt to new threats, providing more accurate and timely alerts.
2. Security Information and Event Management (SIEM)
Machine learning algorithms can enhance SIEM systems by correlating events, identifying patterns, and reducing false positives. This allows security teams to focus on genuine threats and respond more effectively.
3. Endpoint Protection
Machine learning is increasingly used in endpoint protection solutions to detect and respond to threats on individual devices. By analyzing data from endpoints, such as computers and mobile devices, machine learning models can identify and block malicious activities, including ransomware and unauthorized access attempts.
4. Network Security
Network security benefits from machine learning through advanced threat detection and response capabilities. Machine learning models can analyze network traffic in real-time to identify potential threats, such as Distributed Denial of Service (DDoS) attacks or data exfiltration attempts. This enables organizations to take swift action to protect their networks.
Challenges and Considerations
1. Data Privacy and Security
The effectiveness of machine learning in cybersecurity relies on access to large volumes of data. However, this raises concerns about data privacy and security. Organizations must ensure that sensitive data is protected and that machine learning models do not inadvertently expose or misuse personal information.
2. Model Accuracy and False Positives
Machine learning models are not infallible and may produce false positives or false negatives. Ensuring the accuracy of these models is crucial for maintaining trust in automated security systems. Continuous monitoring and refinement of machine learning models are necessary to minimize errors and improve their reliability.
3. Adversarial Attacks
Adversaries can also leverage machine learning to enhance their attacks. For example, attackers may use techniques to deceive or bypass machine learning models, making it essential for security professionals to stay ahead of evolving threats and continuously update their defenses.
Future Trends in Machine Learning and Cybersecurity
1. Integration with Zero Trust Architecture
The Zero Trust Architecture (ZTA) model assumes that threats may exist both inside and outside the network, and thus, no entity should be trusted by default. Machine learning can play a significant role in ZTA by continuously analyzing and validating user and device behavior, ensuring that only authorized entities can access resources.
2. Enhanced Threat Intelligence
Machine learning can improve threat intelligence by aggregating and analyzing data from various sources, such as threat feeds, vulnerability databases, and security incidents. This enhanced threat intelligence enables organizations to stay informed about emerging threats and adapt their security strategies accordingly.
3. Evolution of AI-Driven Security Tools
The development of AI-driven security tools is expected to advance significantly, with machine learning algorithms becoming more sophisticated and capable of handling complex threats. These tools will likely offer more accurate detection and prevention capabilities, further enhancing cybersecurity defenses.
Conclusion
Machine learning is transforming the field of cybersecurity by providing advanced capabilities for threat detection and prevention. By leveraging machine learning algorithms, organizations can enhance their ability to identify and respond to cyber threats in real-time. To stay ahead in this rapidly evolving field, enrolling in a Machine Learning Course in Noida, Delhi, Mumbai, Indore, and other parts of India can be crucial. However, it is essential to address challenges related to data privacy, model accuracy, and adversarial attacks to maximize the benefits of machine learning in cybersecurity. As technology continues to evolve, the integration of machine learning with other security strategies will play a crucial role in safeguarding digital assets and maintaining a secure digital environment.
Subscribe to my newsletter
Read articles from Ruhi Parveen directly inside your inbox. Subscribe to the newsletter, and don't miss out.
Written by

Ruhi Parveen
Ruhi Parveen
I am a Digital Marketer and Content Marketing Specialist, I enjoy technical and non-technical writing. I enjoy learning something new.