OBJECT DETECTION IN DARK IMAGES: Challenges and Innovation
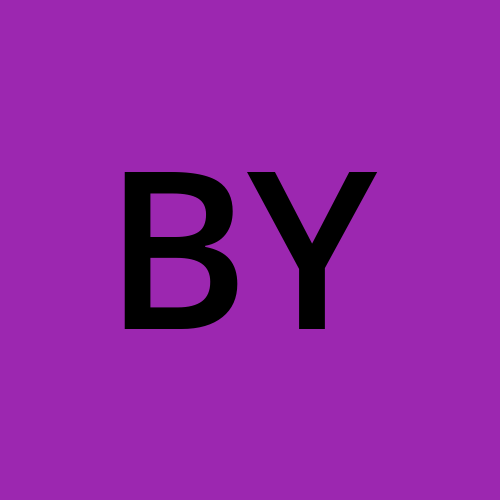
Table of contents
- Introduction:
- Challenges in Object Detection under Low-Light Conditions
- Techniques for Enhancing Image Quality
- Deep Learning Models for Low-Light Object Detection
- Image Augmentation for Low-Light Conditions
- Using Infrared and Thermal Imaging
- Transfer Learning and Pretrained Models
- Evaluation Metrics for Low-Light Object Detection
- Real-World Applications and Case Studies
- Future Trends and Innovations
- Additional Considerations
- Conclusion
Introduction:
Object detection has become an essential technology in various fields, ranging from surveillance to autonomous driving. However, one of the significant challenges faced by this technology is detecting objects in low-light or dark conditions. The ability to accurately identify objects in such environments is crucial for applications where lighting conditions are not always optimal, such as nighttime security surveillance or driving at night. This blog explores the challenges and innovations in object detection under dark conditions, providing insights into the techniques and technologies that enhance detection accuracy.
Challenges in Object Detection under Low-Light Conditions
Object detection in low-light conditions poses several unique challenges:
Noise and Image Quality Degradation: Images captured in low light often suffer from high levels of noise, which can obscure important features and details necessary for accurate object detection.
Limited Visibility and Contrast: Low-light images typically have poor visibility and contrast, making it difficult to distinguish objects from the background.
Motion Blur and Other Artifacts: Longer exposure times needed to capture more light can result in motion blur, further complicating object detection.
Techniques for Enhancing Image Quality
To address these challenges, several techniques can be employed to enhance image quality:
Image Preprocessing Methods: Preprocessing techniques such as histogram equalization and gamma correction can improve the brightness and contrast of low-light images.
Noise Reduction Techniques: Algorithms like Gaussian blur, median filtering, and non-local means denoising can help reduce noise while preserving essential details.
Contrast Enhancement Algorithms: Methods like adaptive histogram equalization (AHE) and contrast-limited adaptive histogram equalization (CLAHE) can enhance the contrast in dark images, making objects more distinguishable.
Deep Learning Models for Low-Light Object Detection
Deep learning has revolutionized object detection, and several models can be adapted for low-light conditions:
Adaptations for Low-Light Conditions: These models can be fine-tuned using datasets that include low-light images to improve their performance in dark environments.
Training Datasets and Techniques: Specialized datasets containing low-light images can be used to train models, and techniques such as transfer learning can be applied to leverage pretrained models.
Image Augmentation for Low-Light Conditions
Image augmentation techniques can help improve the robustness of object detection models in low-light conditions:
Creating Synthetic Low-Light Images: Generating synthetic low-light images from well-lit images can help augment the training dataset.
Data Augmentation Strategies: Techniques such as random cropping, rotation, and flipping can increase the diversity of the training data, making the model more robust.
Balancing Datasets for Better Model Performance: Ensuring a balanced dataset with an adequate number of low-light images is crucial for training effective models.
Using Infrared and Thermal Imaging
Infrared (IR) and thermal imaging technologies can complement conventional imaging in low-light conditions:
Benefits of Infrared and Thermal Imaging: These technologies can capture images in complete darkness, providing additional data for object detection.
Integration with Conventional Image Data: Combining IR or thermal images with conventional images can enhance the overall detection accuracy.
Case Studies and Applications: Examples of using IR and thermal imaging in surveillance, wildlife monitoring, and industrial applications demonstrate their effectiveness.
Transfer Learning and Pretrained Models
Transfer learning can significantly improve the performance of object detection models in low-light conditions:
Leveraging Pretrained Models: Using models pretrained on large datasets such as ImageNet can provide a solid foundation for low-light object detection.
Fine-Tuning Techniques: Fine-tuning these pretrained models on low-light datasets can enhance their performance for specific applications.
Performance Benchmarks: Comparing the performance of fine-tuned models with baseline models highlights the benefits of transfer learning.
Evaluation Metrics for Low-Light Object Detection
Evaluating the performance of object detection models in low-light conditions requires specific metrics:
Precision, Recall, and F1-Score: Standard metrics such as precision, recall, and F1-score are used to evaluate model performance.
Specific Challenges in Evaluating Low-Light Models: Low-light conditions introduce unique challenges that must be considered during evaluation, such as varying noise levels and contrast.
Real-World Testing and Validation: Testing models in real-world low-light scenarios is essential to ensure their effectiveness in practical applications.
Real-World Applications and Case Studies
Object detection in dark images has numerous real-world applications:
Surveillance and Security: Enhancing security camera systems to detect intruders in low-light conditions.
Autonomous Vehicles: Improving the safety of autonomous driving systems by detecting obstacles in low-light environments.
Wildlife Monitoring: Monitoring nocturnal wildlife activities using low-light and thermal imaging.
Industrial and Manufacturing Applications: Ensuring the safety and efficiency of industrial processes that occur in low-light conditions.
Future Trends and Innovations
The field of object detection in dark images is continuously evolving, with several exciting trends and innovations:
Advances in Sensor Technology: Improvements in sensor technology can enhance the quality of low-light images.
Emerging Algorithms and Techniques: New algorithms and techniques are being developed to address the challenges of low-light object detection.
Integration with Other AI Technologies: Combining object detection with other AI technologies, such as natural language processing and decision-making systems, can create more comprehensive solutions.
Additional Considerations
Infrared Imaging: Explore the use of infrared cameras for improved object detection in low-light conditions.
Loss Function Optimization: Customize the loss function to prioritize detections in dark regions.
Ensemble Methods: Combine multiple models for better performance.
Conclusion
Object detection in dark images is a critical area of research with significant practical implications. By addressing the challenges of low-light conditions and leveraging advanced techniques and technologies, we can improve the accuracy and reliability of object detection systems. Future research and innovations will continue to enhance our ability to detect objects in the dark, opening up new possibilities for various applications.
Subscribe to my newsletter
Read articles from Badam Yasaswi directly inside your inbox. Subscribe to the newsletter, and don't miss out.
Written by
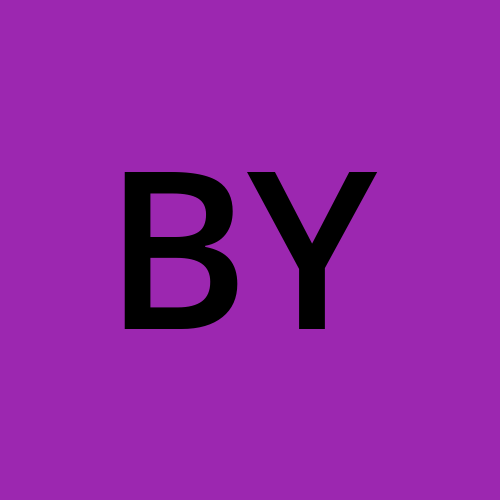