Big Data and Beyond: Types of Data Essential for Effective Analytics

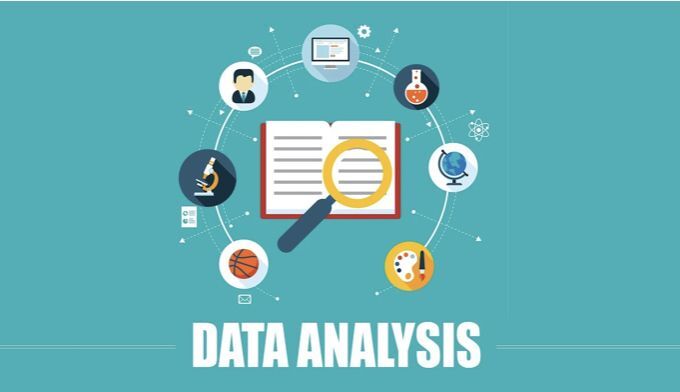
In today's data-driven world, analyzing vast amounts of information is crucial for businesses and organizations. While Big Data plays a significant role in this process, understanding the various types of data that feed into effective analytics is equally important. This article explores the different types of data essential for effective analytics, providing insights into their sources, characteristics, and applications.
1. Structured Data
Definition and Characteristics
Structured data refers to highly organized and easily searchable information. It is typically stored in relational databases and can be easily entered, queried, and analyzed. Examples of structured data include customer records, transaction histories, and inventory data.
Applications in Analytics
Customer Insights: Analyzing structured data from customer relationship management (CRM) systems allows businesses to gain valuable insights into customer behavior, preferences, and purchase patterns.
Operational Efficiency: Inventory data analysis helps companies optimize stock levels, reduce waste, and improve supply chain efficiency.
Financial Analysis: Structured financial data allows precise tracking of revenues, expenses, and profitability, aiding in financial planning and decision-making.
2. Unstructured Data
Definition and Characteristics
Unstructured data lacks a predefined format or structure, making it more challenging to analyze. It includes text documents, emails, social media posts, videos, and images. Despite its complexity, unstructured data contains a wealth of information that can provide deep insights when properly analyzed.
Applications in Analytics
Sentiment Analysis: Analyzing social media posts, reviews, and customer feedback helps businesses gauge public sentiment and adjust their strategies accordingly.
Content Analysis: Mining unstructured data from documents, emails, and reports can extract valuable information, identify trends, and support decision-making.
Image and Video Analysis: Visual data analysis can help in various fields, such as security (facial recognition), marketing (advertisement effectiveness), and healthcare (medical imaging).
3. Semi-Structured Data
Definition and Characteristics
Semi-structured data contains elements of both structured and unstructured data. It does not reside in a relational database but has some organizational properties, such as tags or markers, that make it easier to analyze. Examples include JSON and XML files, web logs, and metadata.
Applications in Analytics
Web Analytics: Analyzing web logs and clickstream data helps businesses understand user behavior on their websites, optimize user experience, and improve online marketing strategies.
Data Integration: Semi-structured data serves as a bridge between structured and unstructured data, enabling seamless data integration and comprehensive analysis.
IoT Data: Internet of Things (IoT) devices generate semi-structured data, which can be analyzed to monitor equipment performance, predict maintenance needs, and improve operational efficiency.
4. Real-Time Data
Definition and Characteristics
Real-time data is information delivered immediately after collection, enabling instant analysis and decision-making. It is critical in scenarios where timely information is essential, such as financial trading, emergency response, and online data analytics training courses in Patna and all cities in India.
Applications in Analytics
Financial Markets: Real-time data is crucial for traders and investors to make informed decisions quickly, respond to market changes, and manage risks effectively.
Healthcare: Real-time data from patient monitors and medical devices allows healthcare providers to make timely interventions and improve patient outcomes.
Supply Chain Management: Real-time tracking of shipments and inventory helps companies respond to changes in demand, avoid stockouts, and enhance customer satisfaction.
5. Historical Data
Definition and Characteristics
Historical data is past data that has been collected and stored over time. It provides a wealth of information for trend analysis, forecasting, and benchmarking. Historical data can be structured, unstructured, or semi-structured.
Applications in Analytics
Trend Analysis: Analyzing historical data helps businesses identify long-term trends, understand market dynamics, and make strategic decisions.
Forecasting: Historical sales data, weather patterns, and economic indicators are used to develop predictive models that forecast future events and conditions.
Benchmarking: Comparing current performance against historical data allows organizations to measure progress, set goals, and identify areas for improvement.
6. External Data
Definition and Characteristics
External data is information sourced from outside the organization. It includes data from market research, social media, public records, and third-party providers. Incorporating external data into analytics provides a broader perspective and enhances decision-making.
Applications in Analytics
Market Research: External data helps businesses understand market trends, customer preferences, and competitive landscapes, enabling them to make informed strategic decisions.
Risk Management: Analyzing external data such as economic indicators, weather forecasts, and geopolitical events helps organizations anticipate and mitigate risks.
Enrichment: Combining external data with internal data enriches the analysis, providing deeper insights and more accurate predictions.
Conclusion
Effective analytics relies on a diverse array of data types, each offering unique insights and applications. Structured data provides clarity and precision, while unstructured data uncovers hidden patterns and sentiments. Semi-structured data bridges the gap, enabling comprehensive analysis. Real-time data offers immediacy, and historical data provides context and foresight. Finally, external data broadens the analytical horizon, incorporating external influences and enhancing decision-making.
As businesses and organizations continue to navigate the complexities of Big Data, understanding and leveraging these various data types will be essential for driving innovation, improving performance, and achieving strategic objectives. Whether you're looking to enhance your skills through an online data analytics training course in Patna or any other city in India, mastering the art of data analysis will undoubtedly open doors to new opportunities and success.
Subscribe to my newsletter
Read articles from Brijesh Prajapati directly inside your inbox. Subscribe to the newsletter, and don't miss out.
Written by

Brijesh Prajapati
Brijesh Prajapati
I'm a digital marketer eager to expand my skills and knowledge. Passionate about staying updated with the latest trends, I thrive on learning new techniques and strategies to enhance my expertise.