Understanding Large Language Models as Social, Metafunctional, Semiotic Systems
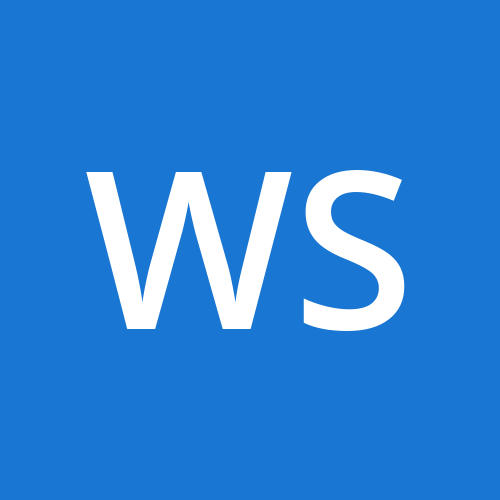
In the rapidly evolving field of artificial intelligence, Large Language Models (LLMs) like GPT-4o have garnered significant attention for their impressive capabilities in natural language understanding and generation. Traditionally, these models have been viewed through the lenses of computational efficiency, algorithmic complexity, and performance metrics. However, there's a growing recognition that to fully grasp their impact and potential, we need to consider them more holistically. This brings us to an intriguing perspective: viewing LLMs as social, metafunctional, semiotic systems.
The Social Dimension of LLMs
At their core, LLMs are designed to engage in interaction. They simulate conversation, process natural language inputs, and generate contextually relevant responses. This interaction mirrors human communication, making LLMs participants in a social system. Here's how:
Interaction and Communication
LLMs are built to understand and produce language that feels natural to humans. They engage in dialogue, respond to questions, provide information, and even participate in debates. This capability positions them as social agents capable of meaningful interaction.
Cultural Reflection
The data used to train LLMs encompasses a vast array of human knowledge, including cultural norms, idiomatic expressions, and societal values. Consequently, these models reflect the cultures and societies from which their training data is derived. This mirroring can both reinforce and challenge existing social norms, making LLMs a significant force in shaping public discourse.
The Metafunctional Perspective
Systemic Functional Linguistics (SFL), a theory developed by Michael Halliday, introduces the idea of metafunctions in language. These metafunctions—ideational, interpersonal, and textual—can be applied to understand how LLMs operate.
Ideational Function
The ideational function concerns the representation of content. LLMs excel at this by generating and organizing information about the world. Whether they're writing essays, summarizing articles, or answering questions, LLMs represent processes, participants, and circumstances in a coherent manner.
Interpersonal Function
This function involves managing social interactions and relationships. LLMs adapt their responses based on the perceived relationship with the user. They can be formal, casual, authoritative, or empathetic, depending on the context of the conversation. This adaptability makes them effective communicators in diverse scenarios.
Textual Function
Coherence and cohesion are essential for effective communication. LLMs ensure that their responses are logically structured and contextually appropriate, maintaining a smooth flow in conversation. They manage thematic progression and reference, much like human communicators do.
The Semiotic Framework
Semiotics, the study of signs and symbols, provides another valuable perspective. Language, after all, is a system of signs used to convey meaning. LLMs, in this sense, operate as sophisticated semiotic systems.
Sign Systems
LLMs interpret and generate signs—words, phrases, sentences—to create meaning. They process these signs according to syntactic (structure), semantic (meaning), and pragmatic (contextual use) rules. This triadic relationship mirrors the semiotic process of sign interpretation and production.
Meaning Construction
Meaning in language is not static; it is constructed dynamically through interaction. LLMs participate in this construction by interpreting user inputs and generating appropriate outputs. This aligns with the semiotic view of communication as an active process of meaning-making.
Holistic Integration: The Way Forward
Viewing LLMs as social, metafunctional, semiotic systems offers a comprehensive understanding of their capabilities and limitations. It emphasizes the importance of context in generating and interpreting responses and highlights the dynamic nature of language.
Contextual Awareness
This perspective underscores the significance of context in shaping meaning. LLMs must be contextually aware to produce relevant and accurate responses. Understanding their role as social participants can enhance their ability to manage contextual nuances.
Addressing Biases and Ethics
Recognizing LLMs as social systems brings ethical considerations to the forefront. It highlights the need to address biases in training data and ensure that these models are developed and deployed responsibly. Ethical AI development must consider the societal impact of LLMs, aiming to mitigate biases and promote fairness.
Dynamic Interaction
LLMs are not static tools; they are dynamic communicators. This perspective encourages a nuanced approach to their development, ensuring they remain adaptive and responsive to the ever-changing landscape of human communication.
Conclusion
Large Language Models represent a significant advancement in AI, but to harness their full potential, we must adopt a holistic view. Seeing LLMs as social, metafunctional, semiotic systems provides a richer understanding of their role in communication and society. This approach not only enhances their development but also ensures they contribute positively to the world, reflecting and shaping the ways we interact and understand each other.
Subscribe to my newsletter
Read articles from William Stetar directly inside your inbox. Subscribe to the newsletter, and don't miss out.
Written by
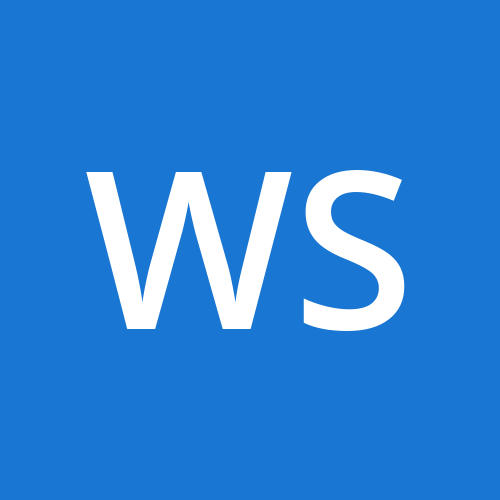