Analyzing NYC Citi Bike Ride Data

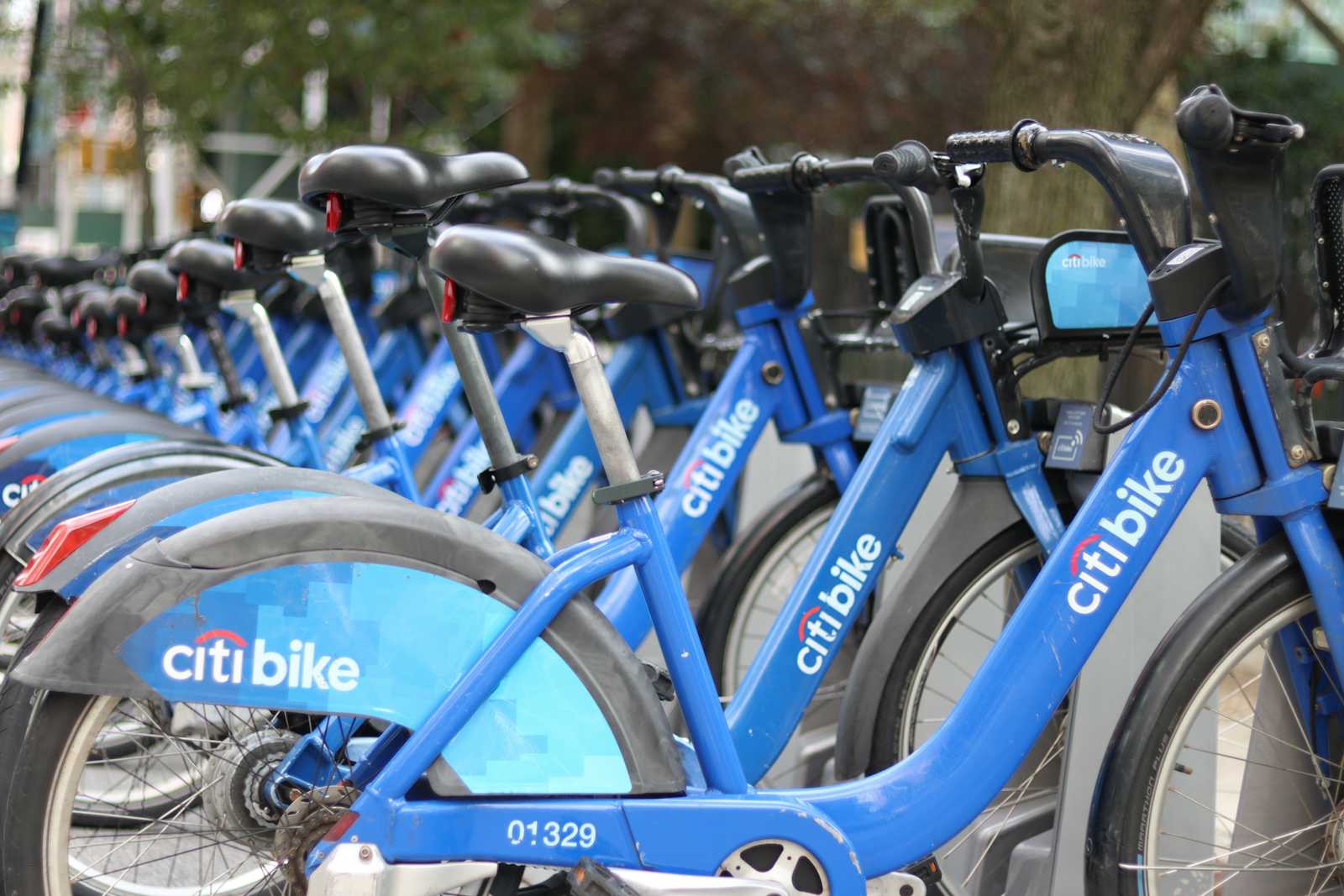
Project Overview
Citi Bike, New York City’s bike-sharing system, has been a transformative addition to the city’s transportation landscape since its launch in 2013. With thousands of bikes and docking stations spread across the city, Citi Bike serves both residents and tourists, offering a sustainable alternative to traditional transport.
As part of the Intro to Data Analytics course by Career Foundry, I assumed the role of a Junior Data Analyst working alongside the operations and marketing teams at Citi Bike. My task was to analyze Citi Bike rider data to understand how different customer segments utilize the service across New York City. By exploring patterns in rider behavior, I aimed to provide actionable insights that would help optimize Citi Bike's offerings and improve overall customer satisfaction.
Problem Statement
As Citi Bike continues to grow, understanding user behavior across different demographics is critical to optimizing the service. This project seeks to explore how age, user type, and other factors influence ride patterns, to identify trends that can inform strategies for service expansion and customer satisfaction. Specifically, this analysis focuses on answering the following key business questions:
Key Business Questions:
What are the most popular pick-up locations across the city?
How does the average trip duration vary across different age groups?
Which age group rents the most bikes?
How does bike rental vary between one-time users and long-term subscribers on different days of the week?
Does user age impact the average trip duration?
Dataset
The dataset for this project comes from Career Foundry’s curated version of Citi Bike’s public trip history logs, which capture detailed information on ride duration, start and end locations, and user types. The original dataset is available on Kaggle.
Methodology
Ask Phase:
Defined the business problem: Optimize Citi Bike service by analyzing ride patterns based on user demographics and usage trends.
Identified stakeholders: Citi Bike management, marketing teams, and city transportation planners.
Prepare Phase:
Data Cleaning: Removed over 3,500 duplicate records and filtered out rows with missing data, which could indicate errors in data entry.
Feature Engineering: Added variables like "weekday" to explore how ride patterns differ throughout the week.
Data Validation: Ensured the dataset was complete and aligned with the project’s objectives for effective analysis.
Process Phase:
Processed the data to ensure it was free from errors, such as duplicates or incomplete records.
Structured the data to facilitate analysis by breaking down ride data by user types, age groups, and days of the week.
Analyze Phase:
Descriptive Statistics: Calculated summary statistics (mean, median, mode) for ride duration, age, and other relevant variables.
Pivot Tables: Used to segment data by age, user type, and day of the week, uncovering patterns in ride duration and popular pick-up locations.
Trend Analysis: Explored bike rent trends over time and identified the relationship between age and trip duration.
Analysis and Visualizations
Popular Pick-Up Locations:
The most popular pick-up stations are located in busy, commuter-heavy areas such as Grove St Path, Exchange Place, Sip Ave, Hamilton Park, and Morris Canal. These locations likely serve both daily commuters and tourists due to their accessibility.
Trip Duration by Age Group:
On average, the oldest age group (75+ years) had the longest trip durations, indicating a preference for more leisurely rides. This suggests opportunities for Citi Bike to cater to older users who might prioritize recreational cycling over commuting.
Bike Rentals by Age Group:
The 35-44 age group rented the most bikes, followed by the 25-34 group. The 18-24 group had the lowest rental rates. This highlights a potential opportunity to increase engagement with younger users, who may not fully appreciate the benefits of the service.
Rental Variations by User Type:
One-time users rented significantly more bikes on weekends, while long-term subscribers used the service more consistently throughout the week. This finding could inform marketing strategies targeting casual riders, particularly tourists, for weekend promotions.
Impact of Age on Trip Duration:
Surprisingly, there was no significant correlation between age and trip duration beyond the oldest age group. Most users, regardless of age, had similar trip lengths. This suggests that trip duration is more closely related to factors other than age, such as trip purpose (e.g., commuting vs. leisure).
Key Findings
Most Popular Locations: High-traffic areas like Grove St Path and Exchange Place are the busiest Citi Bike locations, serving as key hubs for bike rentals.
Trip Duration Trends: The average trip duration increases with age, particularly among older riders (75+), who tend to take longer, more leisurely rides.
Bike Rentals by Age: The 35-44 age group dominates bike rentals, followed by the 25-34 age group. Younger riders (18-24) rent bikes the least.
User Type Variations: One-time users prefer weekend rentals, while long-term subscribers show steady usage throughout the week.
Age Impact on Trip Duration: There is no significant correlation between age and trip duration across most age groups, suggesting other factors drive trip lengths.
Insights and Recommendations
Optimize Bike Availability: Install more bikes and docking stations in high-traffic areas like Grove St Path, Exchange Place, and Hamilton Park to meet demand and reduce wait times.
Targeted Marketing Campaigns: Citi Bike should focus on increasing engagement with younger users (18-24) through targeted campaigns highlighting the convenience, affordability, and health benefits of the service, particularly promoting long-term subscriptions.
Personalized User Experience: Citi Bike can enhance its app to offer personalized route recommendations, especially for older users who prefer longer, scenic rides.
Weekend Promotions: Given the surge in one-time user rentals on weekends, Citi Bike could introduce weekend promotions or discounts to attract more tourists and casual riders during high-demand periods.
Service Expansion: Expand the Citi Bike docking network in tourist-heavy areas and neighborhoods experiencing high weekend demand to cater to one-time users.
Conclusion
This Citi Bike ride data analysis provides actionable insights into how different demographics and user types utilize the service. By understanding these patterns, Citi Bike can make data-driven decisions to optimize its offerings, improve customer satisfaction, and continue its expansion across New York City. The findings point to opportunities to expand bike availability, enhance the app experience, and implement targeted marketing efforts to grow its customer base and meet the diverse needs of its users.
Subscribe to my newsletter
Read articles from Innocent Ezama directly inside your inbox. Subscribe to the newsletter, and don't miss out.
Written by

Innocent Ezama
Innocent Ezama
Data Analyst| Data Scientist | Technical Writer | Seeking Opportunities to Drive Impactful Solutions in Tech