Insider Threat Detection Using Behavioral Analysis
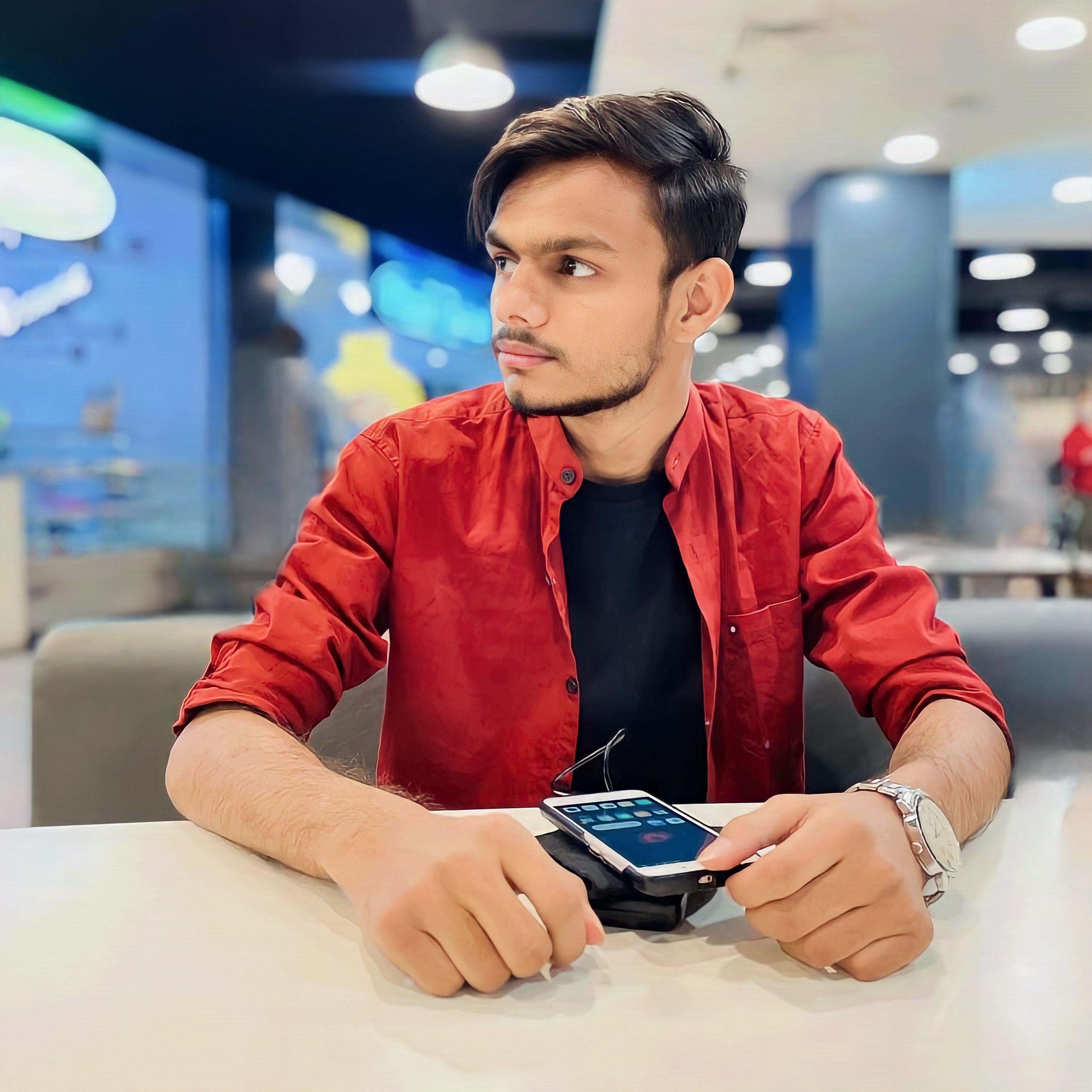

In the age of rapid digital transformation, cybersecurity challenges have grown more complex, and among them, insider threats remain one of the most perplexing. While most organizations focus on external attacks, such as hackers or phishing schemes, insider threats—where the risk comes from within—are equally, if not more, concerning.
So, what exactly is an insider threat?
Simply put, it is a security risk that originates from within an organization. The perpetrator could be a current or former employee, contractor, or business partner who has access to critical systems or data and uses that access to harm the organization intentionally or accidentally. This is where behavioral analysis becomes a vital tool in detecting and mitigating such threats.
The Invisible Enemy: Insider Threats
In contrast to external threats, insider threats can be more subtle and difficult to detect. The individuals behind them already have legitimate access to sensitive systems and data. This makes their actions appear less suspicious on the surface. Insider threats can range from malicious intent, like stealing sensitive data or intellectual property, to inadvertent actions, such as falling prey to phishing attacks, causing unintentional damage.
Why are insider threats so dangerous?
Because they often go unnoticed for long periods, causing significant damage before they are discovered. According to a study by the Ponemon Institute, the average cost of an insider threat incident in 2023 was around $15.4 million, highlighting the enormous financial and reputational damage organizations face due to such threats.
Enter Behavioral Analysis: Uncovering the Unseen Patterns
Behavioral analysis is an advanced technique used to identify abnormal behaviors that could indicate an insider threat. By collecting and analyzing large amounts of data related to user activities, this approach can detect anomalies that deviate from the norm, offering a deeper understanding of potential risks.
But how does this work in practice? Let’s break it down.
1. Understanding Normal Behavior
The first step in behavioral analysis is to define what “normal” looks like for each user. This involves monitoring the day-to-day activities of individuals, such as login times, file access patterns, email communication frequency, and even physical access to certain areas of the organization.
For instance, if an employee usually accesses the system between 9 AM and 5 PM and suddenly starts logging in at odd hours or from unusual locations, that could raise a red flag. Similarly, an employee who normally interacts with a certain set of files but suddenly begins accessing confidential files outside their role might be demonstrating a sign of risky behavior.
2. Identifying Anomalies
Once a baseline of normal behavior is established, the next step is to look for deviations. These anomalies can be subtle or overt. For example, a spike in file downloads, unusual use of external storage devices, or excessive access to databases can be indications of an insider threat.
Behavioral analytics tools powered by machine learning algorithms can analyze these deviations in real-time, flagging potential risks before they escalate. Machine learning models are especially beneficial as they can continuously learn and adapt to changing behaviors, ensuring that the system stays up-to-date with new patterns.
3. Correlating Data for Context
Not all anomalies indicate malicious intent. An employee may work late on a project or download files to complete a task remotely. This is where context becomes essential. Behavioral analysis tools do more than just identify anomalies—they also correlate different pieces of data to give security teams a clearer picture of what is happening.
For instance, if an employee who is scheduled to leave the company in a few weeks suddenly begins transferring sensitive data to external drives, this could be cause for concern. The key is correlating behavior with other relevant data points, such as HR records, access logs, or even communication with competitors.
The Role of Machine Learning in Behavioral Analysis
Machine learning (ML) is revolutionizing the field of cybersecurity, and insider threat detection is no exception. With the ability to process massive amounts of data, ML algorithms can detect patterns that are too complex for human analysts to identify.
ML models can analyze user behavior in real-time, learning from past incidents to predict future risks. Over time, they become more accurate in detecting anomalies that could signal insider threats. For example, an ML model might recognize that a certain type of file transfer, when combined with a specific set of email interactions, is a strong predictor of data theft.
Moreover, machine learning models can be designed to reduce false positives. Traditional rule-based systems might flag a high volume of false positives, overwhelming security teams with alerts. But with machine learning, the system can better distinguish between legitimate activities and those that truly pose a risk.
Real-World Example: Detecting Insider Threats Using Behavioral Analysis
Let’s consider a real-world example to illustrate how behavioral analysis can effectively detect insider threats.
A large financial institution was facing issues with data leakage. Sensitive customer data was being accessed by unauthorized personnel, but traditional security measures weren’t able to identify the source. The institution deployed a behavioral analysis tool that monitored the daily activities of all employees.
The system detected that an employee who typically accessed client data related to one department began accessing confidential information from a completely different department. Additionally, this employee was logging in from a personal device outside of normal working hours.
Upon further investigation, it was discovered that the employee was planning to leave the company and was in talks with a competitor. The employee had been collecting sensitive data to share with their new employer. The behavioral analysis tool allowed the institution to detect this malicious activity before any data was leaked, preventing a potentially massive security breach.
The Challenges of Insider Threat Detection
Despite the benefits of behavioral analysis, detecting insider threats is not without its challenges.
1. Data Privacy Concerns: Monitoring employee activities can raise privacy concerns. Organizations need to strike a balance between protecting their data and respecting the privacy of their employees. It’s essential to have clear policies in place that outline how data will be collected, monitored, and used.
2. False Positives: While machine learning can reduce the number of false positives, they are still a challenge. Security teams must be able to distinguish between legitimate anomalies and those that pose a real threat. This requires constant fine-tuning of the system.
3. Insufficient Data: Behavioral analysis relies heavily on data, and sometimes, the available data may not be sufficient to detect threats accurately. Organizations need to ensure that they are collecting comprehensive data without overwhelming their systems.
Conclusion: Strengthening Security from Within
Insider threats represent a significant risk to organizations, but with the right tools, such as behavioral analysis, these threats can be mitigated. By monitoring user behavior, identifying anomalies, and correlating data for context, organizations can detect potential risks before they escalate into full-blown security breaches.
While challenges remain, the integration of machine learning with behavioral analysis offers a promising approach to insider threat detection. As organizations continue to evolve in the digital age, protecting against insider threats will be crucial to maintaining data integrity and safeguarding valuable assets.
At the end of the day, the question organizations must ask themselves is: Do we have the visibility into our users’ behavior to catch the threats lurking within?
Stay Vigilant, Stay Secure.
Subscribe to my newsletter
Read articles from Atharv Patil directly inside your inbox. Subscribe to the newsletter, and don't miss out.
Written by
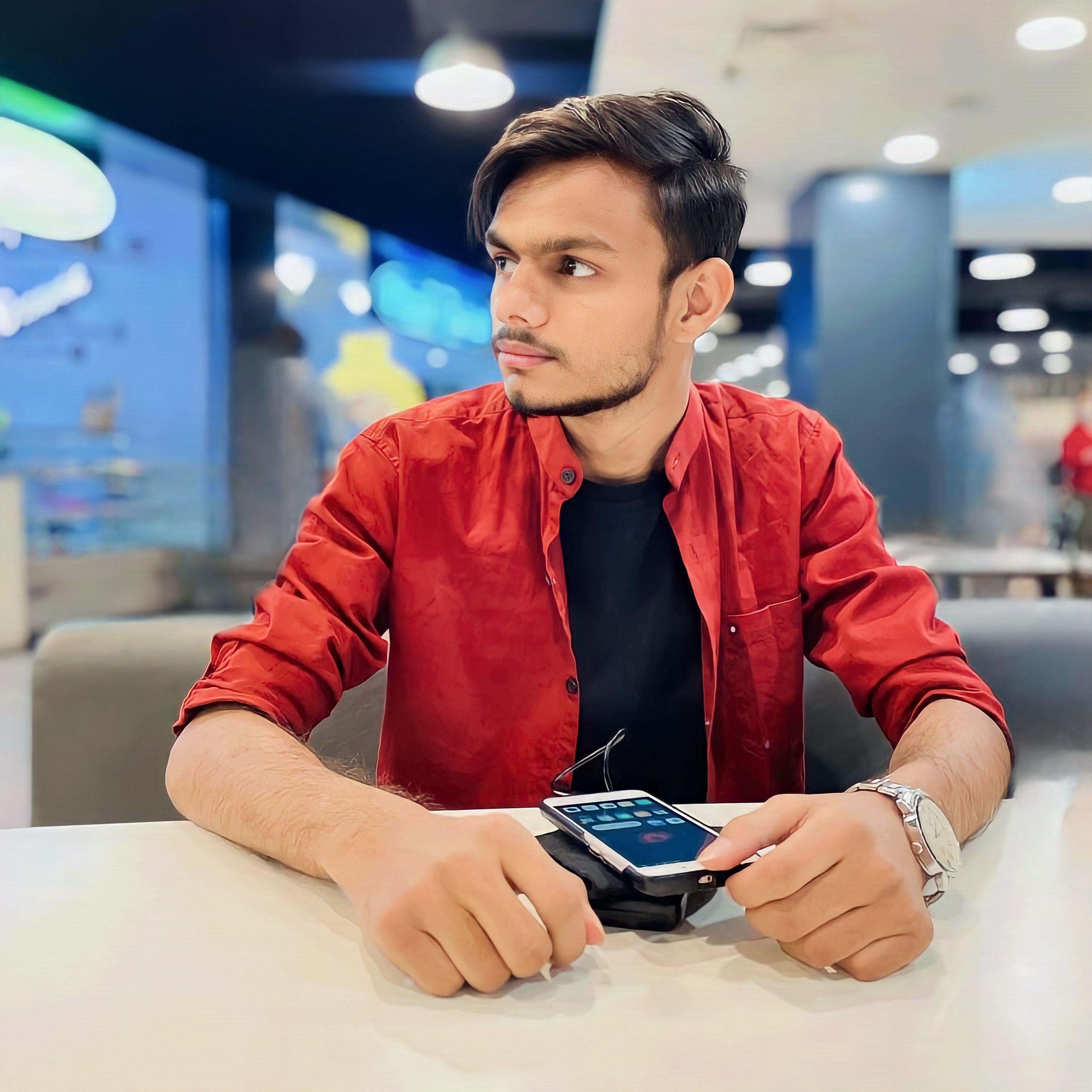
Atharv Patil
Atharv Patil
Encrypting my life one bit at a time from the comforts of 127.0.0.1