Multimodal AI: The New Sensation in Artificial Intelligence
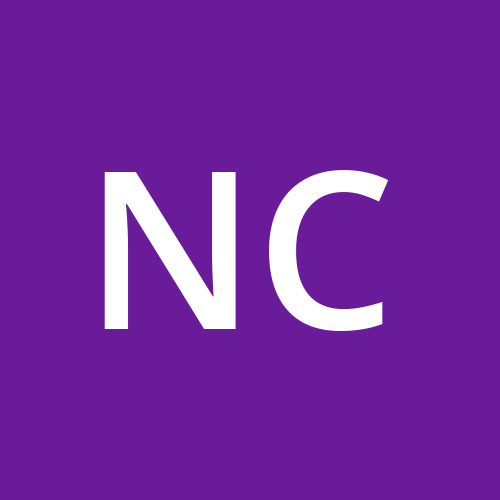
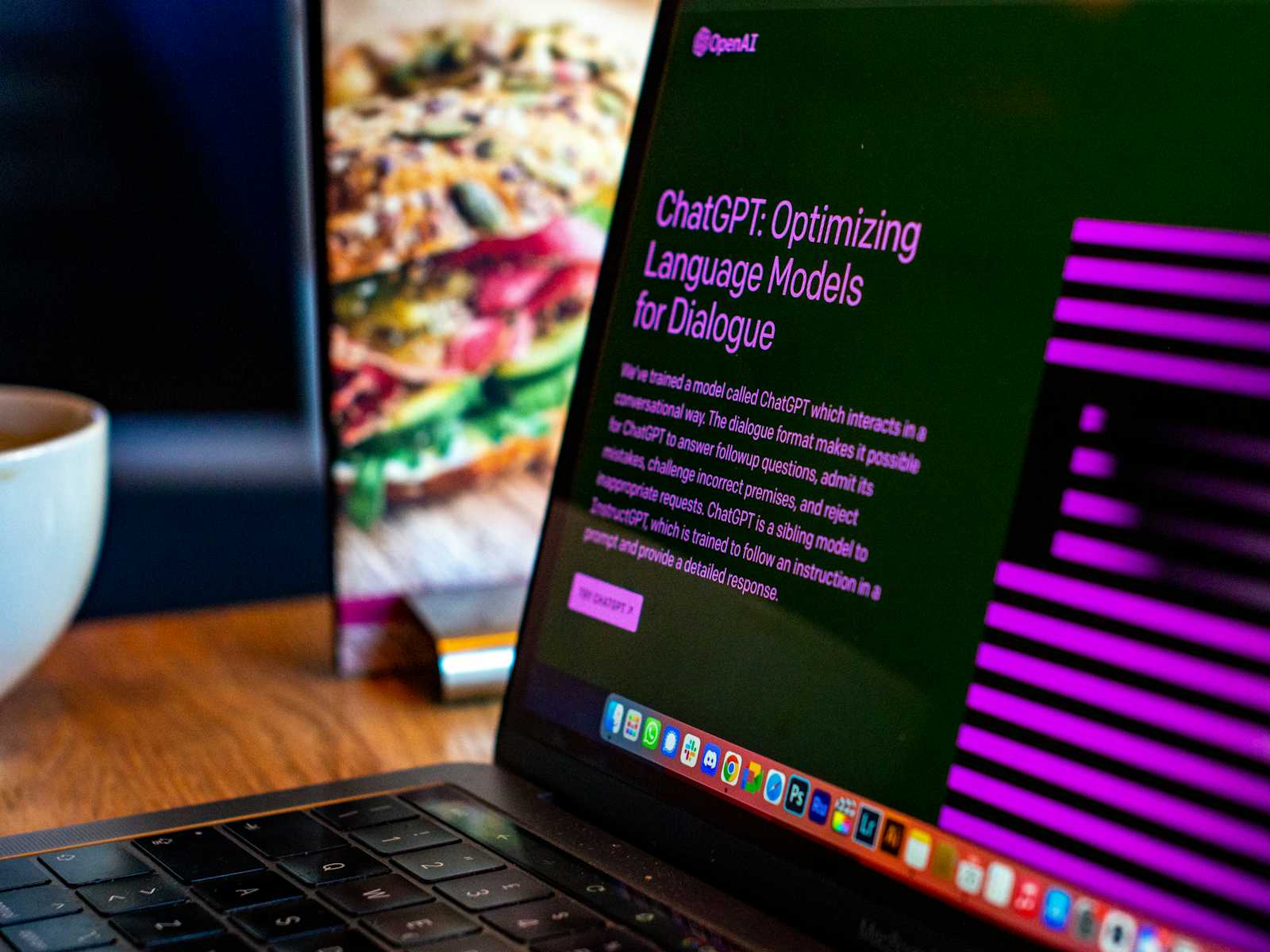
What exactly is this “Multimodal AI,” and how does it work? Let’s break it down.
Multimodal AI refers to an artificial intelligence system that processes and integrates multiple types of data inputs, like text, audio, and images, to produce a unified output. The term “multimodality” signifies an AI model’s ability to handle diverse forms of data.
A prime example of this is OpenAi’s GPT-4V(ision). The key difference between GPT-4 and the Vision variant is its ability to process image inputs alongside text. Other examples include Runway Gen-2 for video generation and Inworld AI for character creation in gaming and digital environments.
Fundamentals of Multimodality
At their core, multimodal models operate on similar principles regardless of the specific modalities in question. For instance, consider a text-to-image model like DALL-E. Modern image models are often built on diffusion models, which generate images from random noise. Text-to-image models, however, integrate text as a modality to guide this image generation.
It’s essential to recognize that different modalities, like text and images, can represent the same semantic concept. For instance, the word “woman” and an image of a woman both represent the concept of a woman but in different forms. Instead of dealing with these representations separately, multimodal AI models work with the underlying meaning. They achieve this by using embeddings that convert both text and images into vectors to capture their semantic content.
These models are typically trained by matching the embeddings of text and visual data based on their cosine similarity, ensuring that the encoders map both text and images into the same semantic space, preserving their meaning.
How Multimodal AI Works
Multimodal AI systems identify patterns across different types of data inputs. They usually consist of three primary elements:
1. An Input Module: Consisting of multiple unimodal neural networks, each receiving a specific type of data.
2. A Fusion Module: This module combines, aligns, and processes the data from each modality using techniques like early fusion, where raw data is concatenated.
3. An Output Module: Generates the final output, which varies based on the initial input.
Challenges & Drawbacks of Multimodal AI
Like any emerging technology, multimodal AI has its challenges:
-Higher Data Requirements: Multimodal AI requires vast and diverse datasets for effective training, which can be expensive and time-consuming to collect and label.
- Data Fusion: Different modalities often exhibit various types of noise and may not be temporally aligned, making the fusion process complex.
- Alignment Issues: Properly aligning relevant data across different modalities, such as text and images, can be challenging.
- Multimodal Translation: Translating content across different modalities or languages, known as multimodal translation, is complex. For example, generating an image from a text description requires the model to understand and maintain semantic consistency across modalities.
Subscribe to my newsletter
Read articles from Nithin ch directly inside your inbox. Subscribe to the newsletter, and don't miss out.
Written by
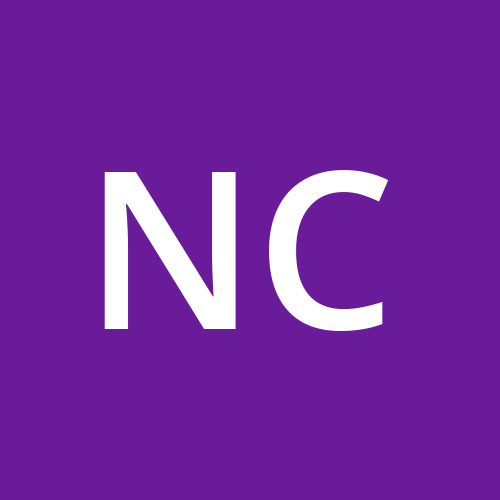